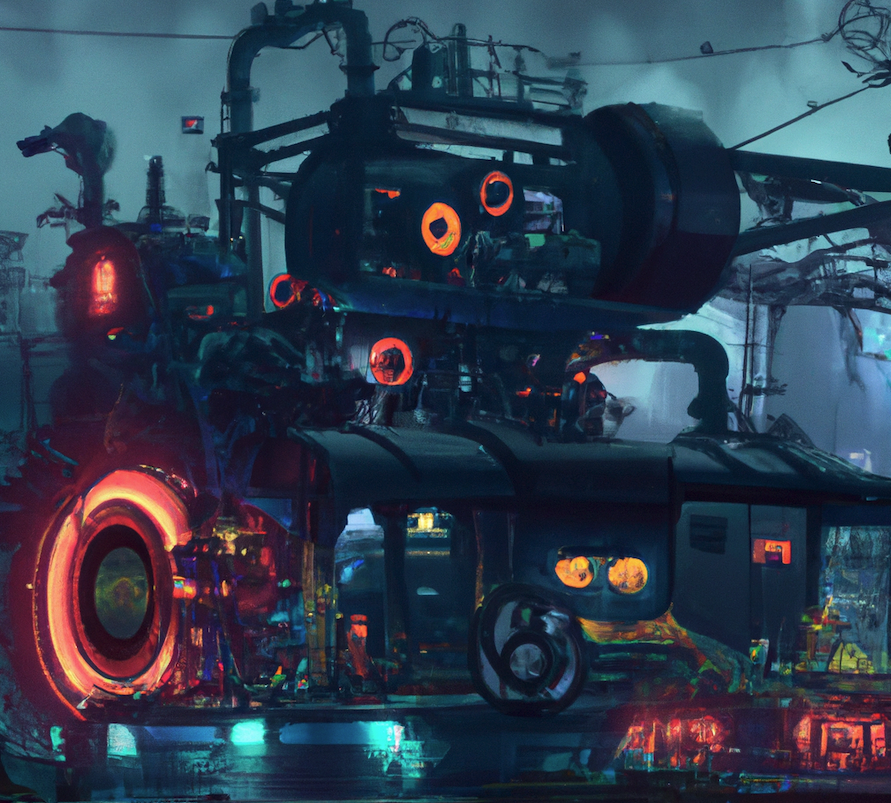
Sponsored By: Brilliant
This article is brought to you by Brilliant, the best way to learn math and computer science in just a few minutes a day.
Megan was brought into the embrace of the good lord Jesus Christ through the power of YouTube. She started with mommy bloggers who had, as she described it, a “really positive energy.” From there, she noticed that they frequented the same Targets and drank Diet Coke from the same drive-throughs and had the same bleached blonde hair and went to the same church—i.e., they were all from Utah.
Her investigation into their personal lives surfaced a video series entitled “I’m a Mormon.” She dove into the deep end of the baptismal font (metaphorically speaking), watching dozens of hours of sermons on YouTube. Eventually, she requested a Book of Mormon to be dropped off at her house. I would know, I was the zitty 20-year-old missionary YouTube put on her doorstep to deliver it. Shortly thereafter, she got dunked in a baptismal font (not metaphorically speaking) and joined the LDS Church. On that day, she reported feeling “hopeful and free for the first time in a long time.”
Jake escaped the grips of the same organization through YouTube. He had recently returned home from a mission to a far-off country and was watching the same “I’m a Mormon” videos. The system then recommended a new series: “I’m an Ex-Mormon.” Jake was sucked in—dozens of hours of videos were consumed. From there, Google directed him to various blogs where people questioned the tenets of the faith he had just spent two years preaching. After several years of questioning and doubting, he left the LDS church. I should know, Jake is my friend. When I asked him how he felt after leaving, he reported, “Hopeful and free for the first time in a long time.” Note: Both names have been changed to protect privacy.
Brilliant is the best way to sharpen your math, data, and computer science skills. Thousands of bite-size, interactive lessons get you hands-on with everything from AI and neural networks to data science, quantum computing, and beyond.
Because Brilliant’s lessons are built to help you master big topics a little at a time, it’s easy to fit it into your busy life.
Join 10M+ people around the world and start your free 30-day trial of Brilliant today. Plus, get 20% off a premium annual subscription.
You may or may not like religion, but that is irrelevant. What matters is this: Did the AI recommendation do good? The emotional outcome was identical for the individuals. To the best of my knowledge, neither person regrets the choice they made. And, still, neither person would’ve made the change they did without YouTube’s recommendation engine surfacing just the right video at just the right time.
The challenge is that “good” is stakeholder dependent. If you’re a devout Mormon, Jake’s choice was bad, potentially dooming his soul. If you’re a committed atheist, Megan was a fool, suckered into a cult. In either case, YouTube finds both outcomes good because the two consumed dozens of hours of ad-supported videos before making this decision. Other stakeholders—like society at large, content producers, governments, or advertisers—may have different perspectives on the relative good of YouTube’s AI-powered conversions.
To further muddy the waters, how much good is even attributable to YouTube is debatable. Ask yourself: What percentage of these two individuals' actions can be credited to the information they received versus their own free will? To what degree do you believe in individual agency?
This isn’t some mere philosophical debate. Over one billion hours of video are consumed by YouTube’s users every day. Over 70% of the videos consumed are surfaced by algorithmic feeds. It is the second most visited website in the world. And the beating heart of its success is a recommendation engine.
Recommendation engines, sometimes called recommendation algorithms, have been blamed for Trump’s election, Biden’s election, mass shootings, the proliferation of vegan restaurants, and TikTok’s dominance. The tech is responsible for you reading this very article. Whether Gmail put this in your “main” inbox, spam, or social tab, the destination was determined by some type of recommendation engine. They permeate e-commerce sites and travel websites. Anywhere there is more information than the eye can scan, algorithms are at work surfacing results.
AI has made that math far more potent. The same scientific advances powering products like ChatGPT or autonomous vehicles are also telling you to watch the new trailer for a Marvel movie. These algorithms don’t operate in a vacuum. They are battling head to head, formula to formula, vying for dominance in the market for eyeballs. I wrote about this phenomenon last May, arguing that “addiction is becoming the blood sacrifice required of consumers to allow businesses to win.” In the war zone of the internet, the most time spent wins.
In the meeting of these two concerns, of commercial interests and ethical conduct, the recent AI boom has me concerned. While we are all still debating the ethical implications of this technology, the algorithms keep getting better. Services are becoming more addicting and there isn’t much an individual can do about it. Tech companies will often defend recommendation engines by pointing out that when they are deployed, use time and customer ratings increase, thus proving that these algorithms are “good.” This is a circular argument—of course these things go up when the customer has access to them, that is what they are designed to do.
It feels like everyone has an opinion on this tech. Some people believe that algorithms are terrible and cause harm, while others believe that they are morally neutral or even helpful in giving people what they want. However, in my opinion, this debate is far too black-and-white and oversimplified. Instead, it would be more productive to examine how algorithms impact our decision-making and culture by considering three rules that are often overlooked by one or both sides.
- Tech companies need to acknowledge that algorithms are deeply editorial, meaning they have the power to shape opinions and perspectives.
- Critics must recognize that design sets the boundaries of influence.
- Third, monetization sets the boundaries for what companies are incentivized to do with these algorithms.
By examining these overlooked factors, we can have more nuanced discussions about the role of AI amplification in society.
Think of these rules as playing a similar role to what physics does during a game of basketball—gravity doesn’t determine who wins the game, but it does determine if the ball goes in the hoop. My three rules don’t determine which system is “good,” but they do allow us to understand how that goodness comes to be.
Now is the time to figure this out. Society stands in the center of an AI cage match. In the left-hand corner, are incredibly powerful AI recommendation engines, and in the right, are new Generative AI tools that are exponentially increasing the volume of content to filter. If we don’t get this right, the past few years of misinformation, election interference, and false panics will look quaint by comparison.
Editorial algorithms
As we discussed earlier, there is no universal "good" when it comes to recommendation engines because you have to balance stakeholder priorities. Editorial algorithms are one such way this phenomena manifests itself—the creators of a product get to determine what values they want to protect or dismantle in their app, by choosing what types of content and topics users will see. Technology isn’t created in an amoral vacuum. It is personal opinion forced upon the world. To see it in practice, we return to YouTube.
When the service was in its infancy, it had two simultaneous methods of recommending features. The first, a delightfully analog attempt, was a team of "cool hunters" whose job was to scour the website for good videos to feature on the homepage. This was paired with a simple algorithm that recommended related videos based on co-visitation; e.g., if you liked this video, another user liked one just like it. But even in those early days, there were editorial choices made with the algorithm’s design. For example, early recommendation experiments in the autos and vehicles category just surfaced a bunch of fetish videos of women’s feet revving engines in luxury cars. The choice was made by the cool hunter in charge of cars to designate this as “bad.” While a group of rather horny dudes who loved cars and feet might disagree with that call, the broader population was probably pleased. Around the same time, another tweak to the system accidentally made the related sections full of ”boobs and asses basically.”
For the first six years of YouTube, the recommendations mostly focused on optimizing videos that got clicks. The results were predictable—quick videos with catchy titles did great. However, in 2012, YouTube shifted the recommendation engine from primarily rewarding clicks to rewarding watch time. Now, rather than rewarding the videos with the most clickable thumbnails, the system would reward creators who had longer videos that people finished. This shift devastated all the creators who had built their editorial brand around being short and punchy. This change caused an initial 25% drop in revenue for YouTube.
By 2017, the platform was using an AI technique called reinforcement learning to recommend videos to viewers. The company made over 300 tweaks to the system that year alone, with the goal of getting the recommendation just right. Its theory was the better the video recommendations, the more time people would spend watching YouTube because they’d get sucked back in. It worked. 70% of YouTube views in 2017 came from recommended videos, and overall views increased by 1%.
However, even with the supposedly neutral AI running the show, editorial choices were made. In one instance, YouTube decided that an excessive amount of videos it deemed “gross” was being displayed on the homepage. To tackle this issue, the company adjusted the algorithm, utilizing a computer model called the "trash video classifier." Even the names of the programs have value statements.
At every decision, with every type of algorithm, Google’s ethical views are fed into the code, determining what videos see the light of day. Measuring engagement as a way to determine video quality, and then maximizing that, is an editorial choice with moral consequences. Each recommendation proffered to a user is a gentle form of persuasion, one that imbues certain values. The lack of transparency regarding the values coded in these recommendation systems obstructs our ability to understand what is happening—and the only reason we know any of this is because of years of dedicated journalism. The company has never been transparent about how it all works.
I like the way Instagram co-founder Kevin Systrom put it when discussing the recent launch of his news recommendation app Artifact. “[B]uilding the algorithm is enormously editorial. Because what you choose to train your algorithm on—the objective function, the data you put in, the data you include, the data you don’t include—is all in editorial judgment,” he said.
When we consider if an AI system is good, we must recognize the value system that the creators are imposing on it.
Design determines destiny
Just as values shape the algorithms’ performance, so does the design of the product in which the engine is housed. To understand the good or bad of an AI recommendation, you need to understand what data inputs and user signals that an app prioritizes.
No company has had a longer history of facing public criticism of their recommendation engine than Facebook has. It feels as if Mark Zuckerberg is called in front of Congress every other week to testify about something. No matter how much money was thrown at it, the recommendation engine could never really get to the point of satisfying everyone. An internal Facebook memo written in 2019, entitled “What Is Collateral Damage?” offers some clues as to why the platform was never able to balance a broader social good with its corporate growth demands:
“We also have compelling evidence that our core product mechanics, such as virality, recommendations, and optimizing for engagement, are a significant part of why these types of [negative] speech flourish on the platform.
“If integrity takes a hands-off stance for these problems, whether for technical (precision) or philosophical reasons, then the net result is that Facebook, taken as a whole, will be actively (if not necessarily consciously) promoting these types of [negative] activities. The mechanics of our platform are not neutral.”
According to the company’s own research, the data inputs for how Facebook makes recommendations, such as likes or shares, were biased toward surfacing negative content. The Facebook files further showed that the company had followed the path of YouTube. They’d notice a problem and make some changes, hoping that the outcome would be OK without necessarily understanding the black box of the algorithm. However, both companies operate at the scale of the entire human race. It isn’t possible for them to make any changes without causing significant harm somewhere.
On the other end of the spectrum, TikTok’s entire app design is entirely focused on improving its recommendation engine. The format of the videos (short) allows for rapid feedback. The video autoplays, meaning that users will immediately show their feelings about the suggestion by watching or swiping. By combining this with machine learning labels (this video has puppies, skiing, etc.), the algorithm can know what you like. Eugene Wei calls this “algorithm-friendly design.”
The outcome is that TikTok created the most addicting experience I’ve ever had in technology. It is TV on crack. It has become one of the few consumer social apps to achieve global success in the past few years. It is so powerful that it has convinced teenagers in the U.S. that they have Tourette syndrome. One stakeholder (TikTok shareholders) would be ecstatic. The kid's parents? Not so much.
Compare this to Facebook, where the recommendation engine was tacked on two years after the company launched via the invention of the News Feed. Because the input signals were less clear, Zuckerberg had a tough time understanding what was happening, meaning the company had a harder time fine-tuning the performance. When we consider whether a system is good, we have to look at how the design shapes the outcomes.
Monetization doesn’t care about your morals
Charlie Munger once said, “Show me the incentive and I will show you the outcome.” When evaluating a recommendation engine, how the company monetizes will affect the relative good. There are three monetization strategies, each with their own trade-offs and costs:
- The current bogeyman is ad models. Companies will deploy AI recommendations to incentivize people to scroll, swipe, and watch for longer. It is rare that someone says, “I am so glad I spent an extra 15 minutes on TikTok today,” but that is what the system is optimized for. The downsides are fairly obvious—at their extreme, ad models can foster misinformation and outrage. Prominent companies like Meta, Snapchat, Twitter, and Pinterest all tune their recommendation engines for this use case.
- The second most common use case for recommendation engines is discrete unit sales. Think about when you buy a product on Amazon and it asks, “Do you also want this?” It is useful, but it also increases the average order value and “makes” people spend more money. Once again, the good of this model is relative. It is good for Amazon, good for the small businesses that sell on the platform, but bad for compulsive shoppers. Sometimes you’ll see these engines not immediately monetize but try to keep people engaged for the chance to sell other products later on. Platforms that monetize via unit sales this way include Airbnb, Etsy, and Walmart.com.
- Finally, there are subscriptions. In this case, recommendation systems are designed to offer a stunted product experience, with embedded cliffhangers that make people pull out their credit cards. Think of Tinder—it deliberately designs the experience to be tantalizing and horny and frustrating all at once. When users are hooked, they are asked to get a subscription to boost their profile and (supposedly) improve their outcomes. Other companies like Netflix, Substack, and The New York Times are compelled to paywall their most hookable content, so when you want the service most you have to pull your credit card out to continue.
Importantly, all of these systems can be construed as having “bad” outcomes. You can also make an equally compelling argument that these monetization schemes are good! Ads make information free, sales don’t burden people with repeat bills, subscriptions foster long-term consumer/creator relationships. Unfortunately, it isn’t as obvious as ads suck.
To make it even more complicated, almost all companies will offer some combination of all three of these methods. Shoot, this very publication has a subscription tier, sells ad slots, and offers educational courses you can buy. I am not immune from criticism along any of these dimensions. My interaction with recommendation engines outside of the occasional tweet or inbox sorting is minimal, but I do think about it a lot!
What actually matters is that all three of these monetization methods are competing in the exact same marketplace—the internet. The winner of a respective market is competing on which method best suits their competitive landscape, not what suits stakeholder good. When we are trying to say whether X or Y system is relatively good, what negative and positive behavior is incentivized must be considered.
Evaluations
Imagine the perfect recommendation engine.
Everyone who uses it is magically inspired to maximize their utility. Suddenly the world is full of people with washboard abs, PhDs, and ever-present smiles. People change their actions because they got the information they needed at the exact time they needed it. This is clearly a fantasy! It will never happen. The gulf between what we say versus what we actually do is huge, colossal, enormous. There is a difference between people’s stated versus revealed preferences.
I just don’t think it is possible to get a recommendation system that makes everyone happy; there are too many stakeholders with directly contradictory needs.
The answer could potentially be that companies should empower users to control the recommendation engines they interact with. This sounds tempting, but I have concerns. We don’t interact with one or two of these engines a day—there are dozens. They are all around us, everpresent, always watching. How likely is it that users are able to correctly individually tune each of these? Real control over these systems would require a technical knowledge of neural networks, weights, and data sets 99.99% of consumers don’t comprehend. Shoot, the very companies that built these things don’t truly understand what happens inside them! Why should users be expected to figure it out? To say that user-empowerment fixes this issue feels like token empowerment versus true individual freedom.
The other supposedly obvious answer would be to just ban them. However, it isn’t that simple. There is simply too much data, too much information on the internet, for us to abolish the techniques. To make the internet usable, we have to have recommendation engines. A world without them is a world with less choice, worse information, and boring entertainment.
Frankly, right now, I don’t know the best answer. The whole point of this essay is to show that there is not a pithy solution to this issue. It requires serious study and hard work for all of us, as technologists, to figure out. I hope that my three frameworks below can allow for us to examine this topic with a more discerning eye:
- Algorithms are editorial
- Design determines outcomes
- Monetization doesn’t care about your feelings
Over the next month, I’ll be examining this topic in detail. It really, really matters. The internet has given me my career, introduced me to my wife, and given me empathy for people like Jake and Megan. I want it to thrive and improve and continue to be one of humanity’s crowning achievements. To keep it and to protect it, this next decade of builders will have to do better.
This essay is the first in a series about AI and recommendation systems that I’ll be writing over the next few weeks. It will cover:
- The AI math and techniques that make this all possible
- The history and development of business models that make this technology profitable
- How AI content generation breaks the whole system
To follow along, just click the follow button below. I can’t wait.
Find Out What
Comes Next in Tech.
Start your free trial.
New ideas to help you build the future—in your inbox, every day. Trusted by over 75,000 readers.
SubscribeAlready have an account? Sign in
What's included?
-
Unlimited access to our daily essays by Dan Shipper, Evan Armstrong, and a roster of the best tech writers on the internet
-
Full access to an archive of hundreds of in-depth articles
-
-
Priority access and subscriber-only discounts to courses, events, and more
-
Ad-free experience
-
Access to our Discord community
Thanks to our Sponsor: Brilliant
For professionals and lifelong learners alike, Brilliant is the best way to learn. Start your free 30-day trial now and master math, data, and computer science in minutes a day.
Comments
Don't have an account? Sign up!