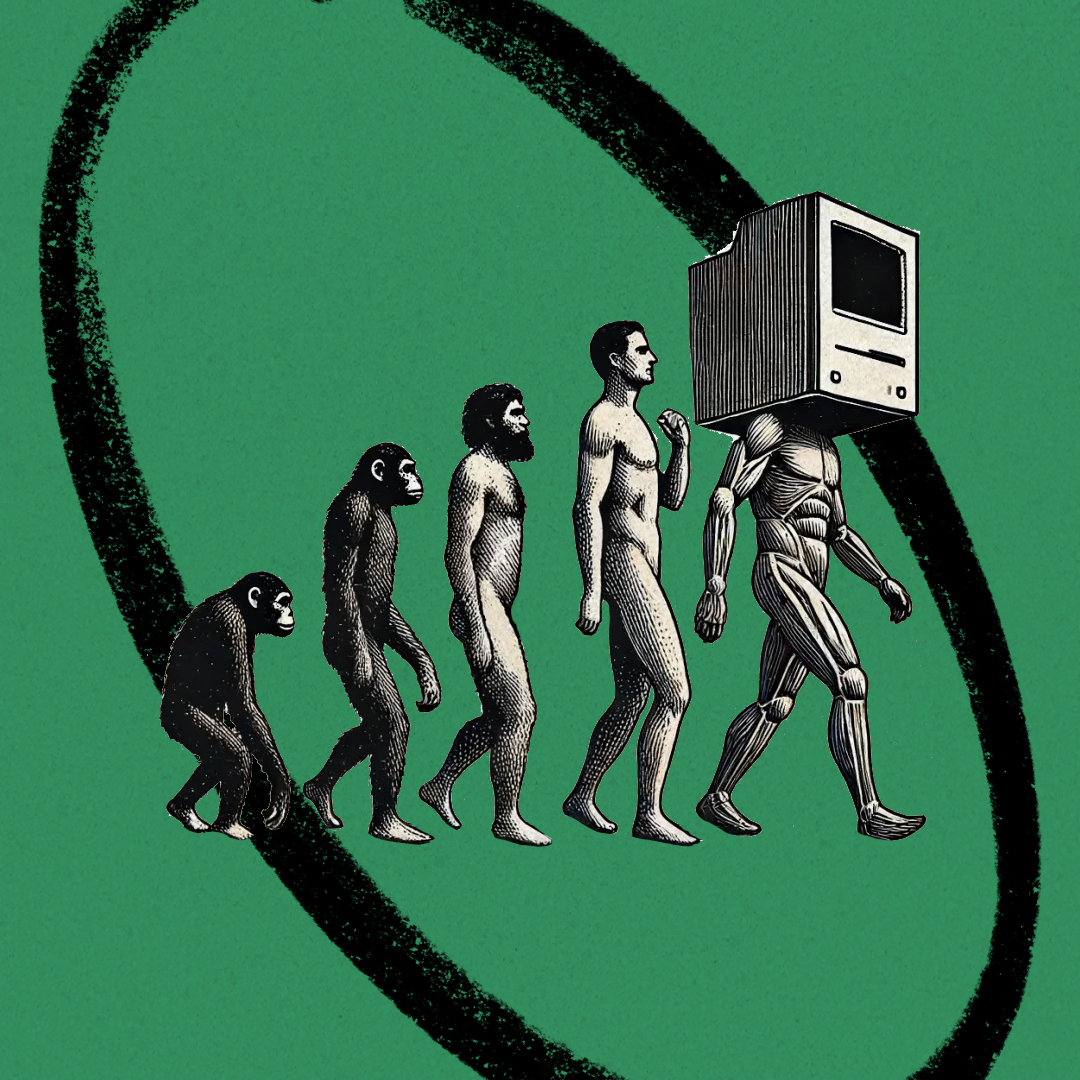
Was this newsletter forwarded to you? Sign up to get it in your inbox.
There are two meaningful divides among technologists in AI. The first is that the technology is an iterative, genuinely useful improvement that will enable new use cases of software. AI is the next cloud, the next smartphone. I’ve covered this perspective extensively over the last few months with the latest Google, Microsoft, and Apple AI product roadmaps.
The other side is more…extreme. It believes that artificial intelligence will someday become artificial general intelligence (AGI) and, from there, self-improve to the point of being 10 times smarter than us. When that happens is debated. Whether that kills or empowers us is debated. How the technology will be able to self-improve is debated. But it is such a strong belief that every single AI research group has at least a few adherents.
Even if you, like most investors and founders today, dismiss the second scenario out of hand, it is worth considering its validity. Because the biggest companies in the world are acting like it is true. Elon Musk believes that we will have AGI in less than two years. Microsoft is drawing up plans for a $100 billion supercomputer to build models big enough for AGI that it intends to finish in about six years. The world’s most important AI companies—OpenAI, Google DeepMind, Anthropic—have AGI as the explicit mission of their organizations.
I’m covering this topic today because of this chart, pulled from a 165-page series of essays from Leopold Aschenbrenner, who was recently fired from OpenAI’s Superalignment research team. The series—entitled "Situational Awareness"—is his argument for why this second group is right and what we should do as a result.
Source: Situational Awareness.The basic thesis of this chart is this: Previous generations of LLMs have had forecastable logarithmic growth in their capabilities. Each time we increase the investment into training by 10x, we get a predictably large leap in LLMs’ capabilities. Aschebrenner believes that we are about two to three orders of magnitudes (OOM) of investment away from AI being able to conduct AI research, making it recursively self-improving. He says that it will be able to do so by making a “hundred million autonomous machine learning researchers.” Sure! Why not?
Aschenbrenner’s choice to publish these essays with a podcast interview and a bunch of Twitter threads is embedded with his own incentives. To me, this writing reads as a spurned lover trying to reclaim what was lost—his position of authority in the AI community after getting the boot from OpenAI. He is doubly incentivized to make a splash with them because he “recently founded an investment firm focused on AGI, with anchor investments from Patrick Collison, John Collison, Nat Friedman, and Daniel Gross.” (Online PDFs are a surprisingly effective way to raise interest in investment vehicles.)
He is so confident in his thesis that he threw the gauntlet at technology equity analysts like me, saying, “Virtually nobody is pricing in what's coming in AI.” First off, rude. Second, maybe he’s right. If superhuman intelligence really is about to be achieved in three years, maybe those five-year financial models I’ve been making are bunk. If nothing else, his writing is a useful framing tool to examine the AGI thesis. At its core, his argument is built on a series of three assumptions.
The three levers of AGI
Let’s start with the Y axis of the chart: “Effective Compute.” This label summarizes the improvements and investments in LLMs in three different ways:
- Compute: The size of the “computer used to train these models,” aka how many GPUs were used
- Algorithmic efficiencies: The quality improvement in techniques that make training these models more efficient and powerful
- “Unhobbling” gains: The tools and techniques that we have developed to give the LLMs additional powers (like browse the internet)
You’ll note that only one of these three variables is actually, like, based on numbers and neatly trackable in a spreadsheet—compute, or how many GPUs are humming. Algorithmic efficiency and “unhobbling” are bets on continuous scientific breakthroughs, not on capital expenditures.
That does not disqualify Aschenbrenner’s calculations! There is precedence for this sort of continuous breakthrough. The oft-cited Moore’s Law—the observation that the number of transistors on a microchip doubles approximately every two years—relies on both the explicitly quantifiable investment in sophisticated chip fabricators and wholly unquantifiable scientific breakthroughs in transistor technology. Aschenbrenner is arguing for a variation on Moore’s Law, in which OOM gains in GPU chip computers and scientific breakthroughs enable a requisite increase in AI capabilities.
His evidence is that, to date, models have gotten “predictably, reliably” better with each OOM increase in effective compute. This has been the case in video generation:
Source: Situational Awareness.Similar jumps in improvement have occurred in the GPT series of models, which can now outperform the vast majority of humans on standardized tests.
Source: Situational Awareness.Models are already so powerful that most benchmarks aren’t useful. They’re simply too smart for many of our tests. With the amount of talent and capital currently devoted to AI, it’s reasonable to expect model improvements for at least for another few years. At the very least, if Nvidia’s $3 trillion market capitalization is any indication, we know that compute will continue to scale.
So far, so good. We might be on a breakneck path to AGI. However, I have major quibbles with Aschenbrenner’s third category: “unhobbling.”
Where the levers break
Aschenbrenner’s first two categories are mostly focused on the improvements in what I would call “making the model smarter.” Each OOM of change from GPT2 to GPT4 increased the accuracy and intelligence of the LLM’s answers. By contrast, he views “unhobbling” differently, writing:
“Critically, don’t just imagine an incredibly smart ChatGPT: unhobbling gains should mean that this looks more like a drop-in remote worker, an incredibly smart agent that can reason and plan and error-correct and knows everything about you and your company and can work on a problem independently for weeks.”
These capabilities are fundamentally different from the capabilities that come from making the models bigger and more effective. Many AI researchers believe that planning is a function of algorithm design, not simply of making the models larger. LLMs’ ability to think on long-term horizons has not yet emerged when you scale models. You have to actually build the models differently, not just make them bigger, which will require a new type of scientific breakthrough—and there’s no quantitative way to measure when that will be. There is a wide range of opinions on the timelines for planning capabilities, all from people who have more degrees than I do. However, this division among scientists is exactly my point. Aschenbrenner’s argument of make-GPU-bigger could work, but it is a major assumption to state as a positive fact.
In addition, “knows everything about you” is not a function of scaling up the model! It is a function of the operating system that the model is given access to and the model’s ability to gather information about you. That’s why I was so excited about Apple’s progress earlier this week in putting LLMs on your iPhone. An additional OOM or two in chips or algorithmic efficiency will have no bearing on whether a model can know everything about you.
“Knows everything about you” also opens up a question of data ownership. I have an unfortunately mind-boggling amount of knowledge about enterprise software. I can promise, in the name of all that is good and holy, that the blocker to an LLM “knowing everything about you” won’t be science. It will be an enterprise procurement process. You can have the most magical software in the world, but if it requires complete access to a corporation’s data warehouses, you’ll be arguing with IT managers for at least 2-3 years.
Everyone from Microsoft CTO Kevin Scott to the myriad AI researchers I’ve spoken to shares a strong confidence that bigger models will solve many of the problems that make the current generation of models feel dumb. However, for them to become independently capable of AI research requires scientific advances outside the scope of the current LLM paradigm. I’m not saying they’re impossible, and hell, they may even happen faster than what “Situational Awareness” proposes, but I think it is far more challenging than what the majority of the AI research community believes.
We’ve also done a disservice to ourselves by characterizing each successive version of GPT models as “more intelligent.” Look at the labels on the right hand of the graph, which compare them to the intelligence of children and teenagers.
Source: Situational Awareness.
I don’t think these characterizations are at all accurate of the model’s capability. Preschool-level GPT2 wasn’t very good at answering questions, but it was strangely haunting and beautiful. Here is a response it gave to the prompt: My 10 favorite animals are: 1,
“My ten favorite animals are:
1. Zebras with a white scar on the back
2. Insiduous spiders and octopus
3. Frog with large leaves, hopefully black
4. Cockatiel with scales
5. Razorbill with wings hanging about 4 inches from one’s face and a heart tattoo on a frog
3. Cockatric interlocking tetrabods that can be blind, cut, and eaten raw:
4. Black and white desert crocodiles living in sunlight
5. Zebra and many other pea bugs”
The numbers are wrong and the answers are nonsensical, but the writing has soul (I love the cockatric one). When I ask GPT4o the same question, the output is a grab bag of animals—no art, no soul.
Source: Author’s screenshot.The hedge for the rest of us
Aschenbrenner’s boldest claim comes on page 41: “We are on course for AGI by 2027. These AI systems will basically be able to automate basically all cognitive jobs (think: all jobs that could be done remotely).” He clarified in his tweet on the topic, “That doesn’t require believing in sci-fi; it just requires believing in straight lines on a graph.”
I will concede that we may have the necessary chip and data infrastructures in place for his AGI timeline. However, the scientific progress required is not just a function of capital allocations; it is a function of genuine innovation, an area of progress that is frustratingly hard to predict. It is presumptuous to say that those gains are certain. And “straight lines on a graph” that 1) is logarithmic and 2) one that you made up isn’t very strong logic. Major capability gains are not just a matter of more compute.
Still, “AGI coming soon” is a commonly held belief system (if not timeline). It seems like the CEOs of these model companies are hedging their bets. While their research teams may believe in AGI by 2027, the CEOs believe in distribution advantages and judicious revenue growth. It’s why the hiring pages of these companies list a much larger number of sales and go-to-market positions than those for researchers. Commercialize today to hedge for AGI tomorrow.
I'm not saying AGI is impossible or that we shouldn't be crazy-excited about the progress in AI. But we need to pump the brakes on the hype train and engage with the full complexity of the challenge. The future of AI is going to be messy, and anyone who tells you otherwise is probably trying to sell you something (or angling for some of that sweet VC money). It’s important to be cautious in our assumptions because if you aren’t—if you just believe in the straight line—you can end up in some hyperbolic situations.
All of the arguments I’ve discussed today only constitute 73 pages of the 165 pages of Aschenbrenner’s writing. The rest of the essay is devoted to what happens in the lead-up to superintelligence. Aschenbrenner’s big concern turns to statecraft, with sections on why China must not gain AGI first, where the energy for training these models will come from (he thinks natural gas in Texas), and how AI will end up being a new Manhattan Project. Again, he may not be wrong. These are important things to consider! But it is worth asking why this obviously brilliant person is devoting their time to this slim-chance hypothetical and why he’s distributing his ideas in inflammatory PDFs and tweets.
The smartest thing we can do is focus on the groundwork: basic research, thoughtful experimentation, and a whole lot of elbow grease—not just drawing straight lines on a chart and calling it a day. AGI isn't destiny, it's a choice. And it's on us to make sure we're putting in the hard work to get it right.
Evan Armstrong is the lead writer for Every, where he writes the Napkin Math column. You can follow him on X at @itsurboyevan and on LinkedIn, and Every on X at @every and on LinkedIn.
Ideas and Apps to
Thrive in the AI Age
The essential toolkit for those shaping the future
"This might be the best value you
can get from an AI subscription."
- Jay S.
Join 100,000+ leaders, builders, and innovators
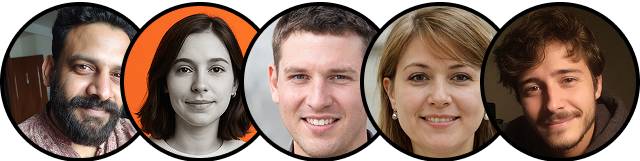
Email address
Already have an account? Sign in
What is included in a subscription?
Daily insights from AI pioneers + early access to powerful AI tools
Comments
Don't have an account? Sign up!
Wonderful article , opens up new thinking
As always from you Ethan, some of the best, balanced and most nuanced thinking on a topic that most authors represent from a tightly held perspective at either end of a belief spectrum. Thank you for all of your great work, it’s always appreciated.
I share your sentiment. I personally don't think current model architectures (even those of recent "enhanced" transformers) can produce an AI capable of discovering new things. Note that "new" here refers to anything humans haven't already put on paper.