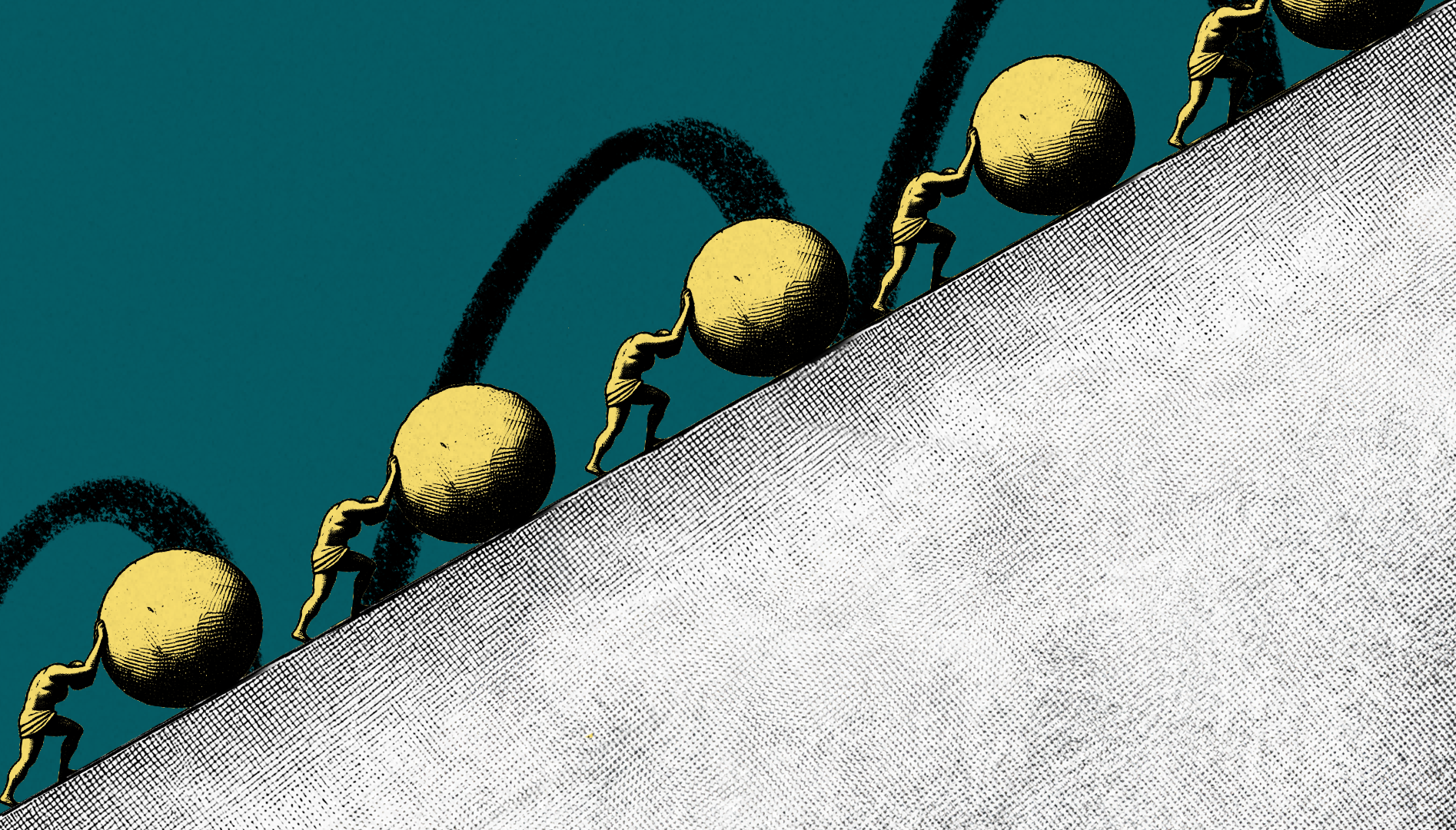
Was this newsletter forwarded to you? Sign up to get it in your inbox.
Contrary to popular belief, this generation of artificial intelligence technology is not going to replace every single job. It’s not going to lead employers to fire every knowledge worker. It’s not going to obviate the need for human writing. It’s not going to destroy the world. We don’t have to strafe the data centers or storm Silicon Valley’s top labs.
The current generation of AI technology doesn’t live up to the AGI hype in that it can’t figure out problems that it hasn’t encountered, in some way, during its training. Neither does it learn from experience. It struggles with modus ponens. It is not a god.
It does, however, very much live up to the hype in that it’s broadly useful for a dizzying variety of tasks, performing at an expert level for many of them. In a sense, it’s like having 10,000 Ph.D.’s available at your fingertips.
The joke about Ph.D.’s is that any given academic tends to know more and more about less and less. They can talk fluently about their own area of study—maybe, the mating habits of giant isopods, or 16th-century Flemish lace-making techniques. But if you put them to work in an entirely new domain that requires the flexibility to learn a different kind of skill—say, filling in as a maître d' during dinner rush at a fancy Manhattan bistro—they’ll tend to flounder.
That’s a little like what language models are. Imagine a group of Ph.D.’s versed in all of human knowledge—everything from the most bizarre academic topics to the finer points of making a peanut butter and jelly sandwich. Now imagine tying all of the Ph.D.s together with a rope and hoisting a metal sign above them that says, “Answers questions for $0.0002,” with a little slot to insert your question. By routing the question to the appropriate Ph.D., this group would know a lot about a lot, but they still might fail at a task sufficiently new to the recorded sum of human knowledge.
This is in line with University of Washington linguistics professor Dr. Emily Bender’s idea of the “stochastic parrot”—that language models are just regurgitating sequences of characters probabilistically based on what they’ve seen in their training data, but without really knowing the “meaning” of the characters themselves.
It’s also in line with observations made by Yann LeCun, chief AI scientist at Meta, who has repeatedly said that large language models can’t answer questions or solve problems that they haven’t been trained on.
There’s room to quibble with whether both of their takes truly represent the current state of the technology. But even if you grant their point, what both Bender and LeCun misunderstand is that they think the powers of the current generation of AI technology is a letdown. They say, in a pejorative sense, that language models are only answering questions they’ve seen in some form in their training data.
I think we should get rid of the “only.” Language models are answering questions they’ve seen before in their training data. HOLY SHIT! That is amazing. What a crazy and important innovation.
LLMs allow us to tap into a vast reservoir of human knowledge and expertise with unprecedented ease and speed. We’re no longer limited by our individual experiences or education. Instead, we can leverage the collective wisdom of humanity to tackle challenges and explore new frontiers.
For anyone trying to figure out what to use AI for, or what kinds of products to build with the current generation of technology, it implies a simple idea: Don’t repeat yourself.
Was this newsletter forwarded to you? Sign up to get it in your inbox.
Contrary to popular belief, this generation of artificial intelligence technology is not going to replace every single job. It’s not going to lead employers to fire every knowledge worker. It’s not going to obviate the need for human writing. It’s not going to destroy the world. We don’t have to strafe the data centers or storm Silicon Valley’s top labs.
The current generation of AI technology doesn’t live up to the AGI hype in that it can’t figure out problems that it hasn’t encountered, in some way, during its training. Neither does it learn from experience. It struggles with modus ponens. It is not a god.
It does, however, very much live up to the hype in that it’s broadly useful for a dizzying variety of tasks, performing at an expert level for many of them. In a sense, it’s like having 10,000 Ph.D.’s available at your fingertips.
The joke about Ph.D.’s is that any given academic tends to know more and more about less and less. They can talk fluently about their own area of study—maybe, the mating habits of giant isopods, or 16th-century Flemish lace-making techniques. But if you put them to work in an entirely new domain that requires the flexibility to learn a different kind of skill—say, filling in as a maître d' during dinner rush at a fancy Manhattan bistro—they’ll tend to flounder.
That’s a little like what language models are. Imagine a group of Ph.D.’s versed in all of human knowledge—everything from the most bizarre academic topics to the finer points of making a peanut butter and jelly sandwich. Now imagine tying all of the Ph.D.s together with a rope and hoisting a metal sign above them that says, “Answers questions for $0.0002,” with a little slot to insert your question. By routing the question to the appropriate Ph.D., this group would know a lot about a lot, but they still might fail at a task sufficiently new to the recorded sum of human knowledge.
This is in line with University of Washington linguistics professor Dr. Emily Bender’s idea of the “stochastic parrot”—that language models are just regurgitating sequences of characters probabilistically based on what they’ve seen in their training data, but without really knowing the “meaning” of the characters themselves.
It’s also in line with observations made by Yann LeCun, chief AI scientist at Meta, who has repeatedly said that large language models can’t answer questions or solve problems that they haven’t been trained on.
There’s room to quibble with whether both of their takes truly represent the current state of the technology. But even if you grant their point, what both Bender and LeCun misunderstand is that they think the powers of the current generation of AI technology is a letdown. They say, in a pejorative sense, that language models are only answering questions they’ve seen in some form in their training data.
I think we should get rid of the “only.” Language models are answering questions they’ve seen before in their training data. HOLY SHIT! That is amazing. What a crazy and important innovation.
LLMs allow us to tap into a vast reservoir of human knowledge and expertise with unprecedented ease and speed. We’re no longer limited by our individual experiences or education. Instead, we can leverage the collective wisdom of humanity to tackle challenges and explore new frontiers.
For anyone trying to figure out what to use AI for, or what kinds of products to build with the current generation of technology, it implies a simple idea: Don’t repeat yourself.
Because language models are good at doing anything they’ve seen done before, they’re good at any human task that’s repetitive. New technology changes how we see the world, and what language models reveal is that there is a lot of our day-to-day that is repetitive.
Take the humble startup founder, for example. Most people start a company because they want to build new things, discover new frontiers, and explore what can be unburdened by what has been.
The reality is that founder life is sometimes like that, but very often it consists of repeating yourself over and over in various, subtly different, contexts. You have to repeat yourself to investors when you pitch them, giving the same biographical details, and telling the same anecdotes over and over again. You do the same thing with potential customers, new hires, and journalists writing articles about you.
You have to repeat yourself with your team—to reinforce the mission, your values, and norms, and how to think about solving problems.
It’s a repetitive job, and that’s part of what makes it so hard. You have to say the same thing all the time without losing enthusiasm, and do so dozens or even hundreds of times a day.
Language models allow us to see this repetition, this drudgery, in a new way because we finally have a solution for it. Imagine Slack bots and email copilots that automatically jump in to answer repetitive questions or provide a first round of feedback by simulating the founder’s perspective in scenarios that have come up before. It would dramatically ease the burden on the founder’s time, opening them up to handle more important work.
Language models expose how much of this same drudgery occurs in every field of human endeavor, and how it might be streamlined.
This shift in how we see the world aligns with what I've previously called the allocation economy. As AI takes over these repetitive tasks, our role changes from doing the work ourselves, to deciding what work needs to be done and how to best allocate our resources to do it.
In the allocation economy, the key skill becomes knowing how to effectively leverage AI to handle these repetitive elements, freeing us up for more creative and strategic thinking.
So, if you’re wondering how your world might change over the next few years, do a little experiment today. Try to notice all of the ways you’re repeating yourself.
Soon enough, language models will be doing a lot of that stuff for you. And that will free you up to do more interesting things.
Dan Shipper is the cofounder and CEO of Every, where he writes the Chain of Thought column and hosts the podcast AI & I. You can follow him on X at @danshipper and on LinkedIn, and Every on X at @every and on LinkedIn.
Ideas and Apps to
Thrive in the AI Age
The essential toolkit for those shaping the future
"This might be the best value you
can get from an AI subscription."
- Jay S.
Join 100,000+ leaders, builders, and innovators
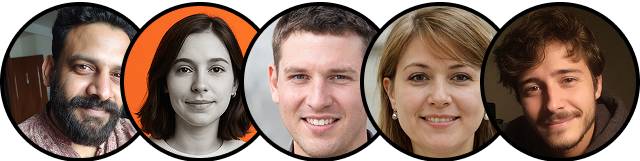
Email address
Already have an account? Sign in
What is included in a subscription?
Daily insights from AI pioneers + early access to powerful AI tools
Ideas and Apps to
Thrive in the AI Age
The essential toolkit for those shaping the future
"This might be the best value you
can get from an AI subscription."
- Jay S.
Join 100,000+ leaders, builders, and innovators
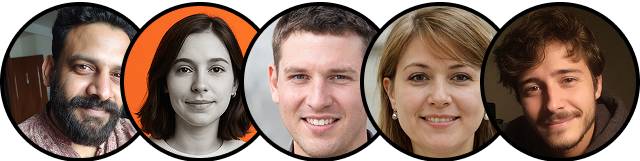
Email address
Already have an account? Sign in
What is included in a subscription?
Daily insights from AI pioneers + early access to powerful AI tools
Comments
Don't have an account? Sign up!
Ahhhhhh!!! That's the Dan Shipper I fell in love with the first time. Clear. Uncomplicated. One main point and no need to crawl through multiple screenshots. We can feel the authority--the lived experience of Dan. He didn't get this from a book or a video. He got this by doing the work and then reporting back to all the Earthlings in a simple exercise we can all do, immediately: Take the time, just for a day, to get aware of your every movement, write it down, and then, tonight or tomorrow, notice where there is repetition. Then make a good decision. Genius.
@[email protected] thanks Georgia!
Fantastic