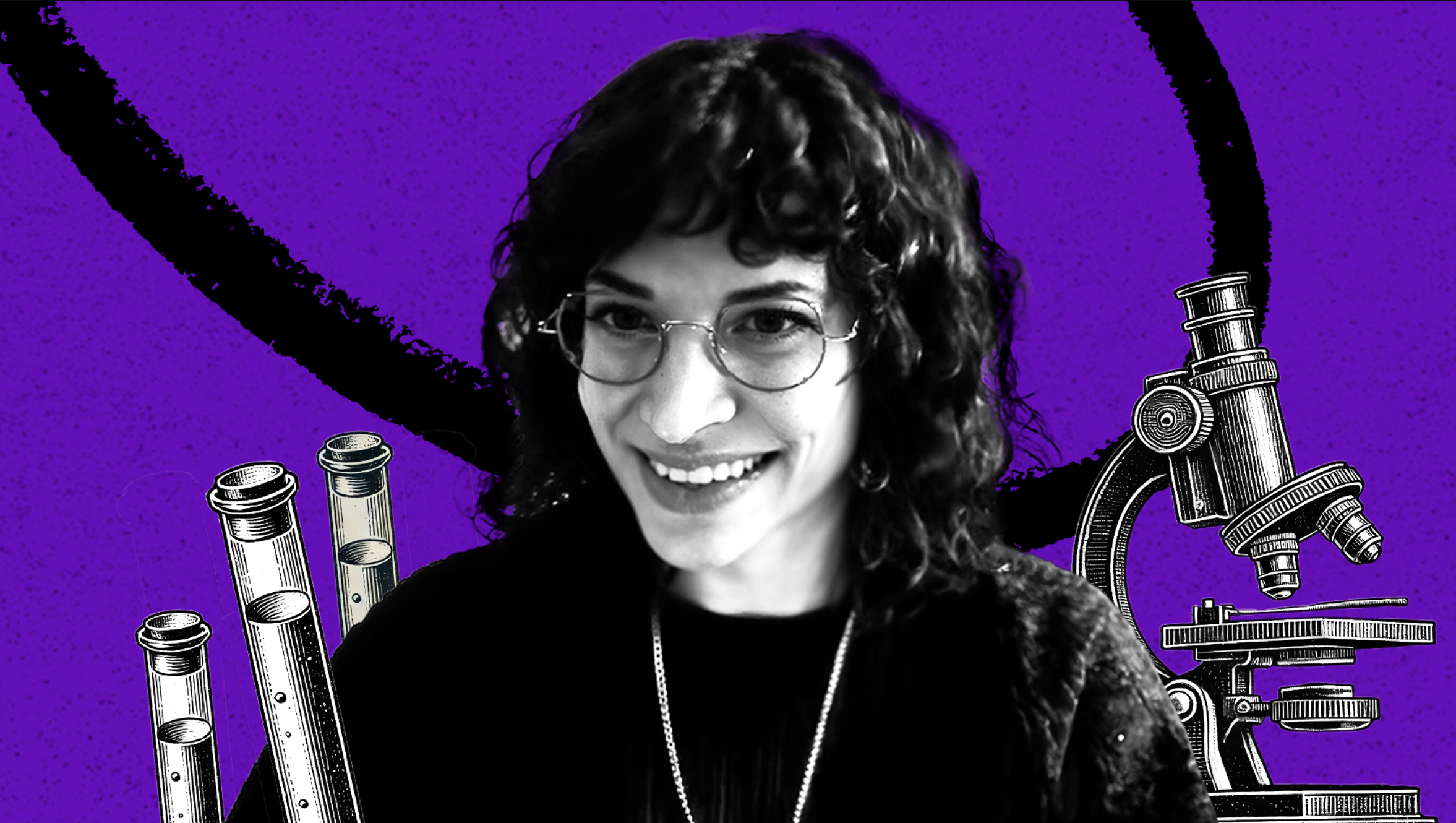
TL;DR: Today weâre releasing a new episode of our podcast AI & I. I go in depth with Alice Albrecht, the senior director of AI product at SmartNews, an app that curates the news for you. Watch on X or YouTube, or listen on Spotify or Apple Podcasts. Also ICYMI: Yesterday, we started onboarding people for Cora, a product that offers you an entirely new way to manage your inbox with AI. Join the waitlist to experience the most human way to do email.Â
I believe AI is going to change science forever.
Small scale studies will give way to large scale open data gathering efforts. Weâll shift from seeking broad general theories to making contextual predictions in individual cases. The traditional research paper will change fundamentally.
Thatâs why I had Alice Albrecht on the show. Few people straddle the worlds of science and AI like she does: She holds a Ph.D. in cognitive neuroscience from Yale and is a machine learning researcher with almost a decade of experience. In 2021, she founded re:collect, an app that aimed to augment human intelligence with AI. It was acqui-hired by news curation app SmartNews in September of this year, and she is now the senior director of AI product.Â
We discuss the contours of this new paradigm of science: the growing importance of data in scientific discovery, how AI makes N-of-1 studies imperativeâwhen theyâre normally seen as unscientific, the case for big tech to open-source their data for research, and the power of unbundling data from interpretations, in both science and media. Here is a link to the episode transcript. Â
Sponsored by: Yandex
GPU training just got faster
Yandex has engineered a solution for one of machine learning's most persistent challenges. YaFSDP, their open-source framework, tackles the complex world of large language model training by revolutionizing how GPUs communicate and process computational tasks. It can:Â
- Accelerate model development by up to 26%Â
- Potentially save $500,000+ in monthly GPU resources
- Optimize training with zero complex configuration
- Supports dynamic graphs and custom optimizers
No more bottlenecks in GPU communication. No more runaway training costs. This is AI efficiency reimagined.
In January of this year, we published Aliceâs thesis about how augmenting human intelligence with AI is more effective than attempting to achieve super intelligence through standalone AI systems, and in a happy coincidence, sheâs our last podcast guest of 2024. Thank you for listening, and weâll see you in the new year.
In the meantime, this is a must-watch for anyone interested in how AI is changing the future of scientific research.
Watch on X or YouTube, or listen on Spotify or Apple Podcasts.
If you want a quick summary, hereâs a taste for paying subscribers:
Reimagine scientific discovery with AI
For Alice, the pressing question around AI research isnât how to build AGI thatâll replace humans, but how to use the technology to augment our intelligence. âThe goal for me is not some sort of super intelligence that works without us. It's: How do we start to connect this technology to humans?â In that vein, this is how Alice believes AI is broadening the horizons of human scientific ability:
- The role of humans in scientific inquiry. Human scientists will continue to play a key role in scientific discovery because they are responsible for shaping the direction of research. Alice explains, âHumans [will] still do a lot of the hypothesis generationâŠand still think critically about [questions like:] âwhat kind of data are we trying to get to understand this?ââÂ
- Information processing at light speed. Alice believes that AI has made it possible for scientists to sift through large chunks of information faster than ever before. She says that âbeing able to comb through all that information and pull things outâ has saved âgraduate students and post-docs countless years.âÂ
- Better ways to explore the space of possibilities. According to Alice, AIâs ability to simulate data from an existing dataset can help scientists map out the constellation of possibilities within complex problems. â[Humans] can make predictions, we can synthesize data, but keeping in mind all of these different kinds of possible future states is really, really, really hard.â
My pet theory about the future of science is that our focus will shift from searching for explanations to making predictions. This is especially relevant in domains like psychology, where causal explanations are hard to pin down. Instead of getting stuck trying to figure out why an individual behaves a certain way, we can use an AI model trained on a high volume of accurate data to make predictions about their behavior.Â
In this data-driven approach to scientific discovery, the traditional research paperâwith its focus on explaining underlying mechanismsâmay be outdated. I wonder if the future of scientific inquiry is to gather high-quality data about problems we care about and allow any scientist to build models on them, instead of writing papers about them. The shift in scientific research from research papers to data gathering and model building mirrors the shift in the late twentieth century from symbolic AIâwhere models learned from explicit rules and logicâto sub-symbolic AIâwhere models learn by identifying patterns in data. As a former scientist herself, this is Aliceâs take on these ideas:Â
- Build in public. According to Alice, publishing papers will remain useful, but she hopes that the focus shifts from publishing only successful studies to including those that failed. âWe are only getting a very weird and skewed slice of science as it were...we might need more papers, and if we have a tool to help us sift through those, maybe it doesn't matter.â
- Open data drives progress. Alice agrees that making datasets from different domains generally available could unlock huge benefits for science, comparing it to how valuable training LLMs on all the text on the internet is. âAs we get more access to data and compute, scientists will become more computational by nature, even if they never were before.â
While acknowledging the benefit of making data accessible, Alice points out that âthe way you chose to collect that data is incredibly importantâŠand so making [the data] transferable is hard.â
Aliceâs argument reminds me of another idea I have about the future of science, particularly in fields like psychology. Traditionally, scienceâperhaps influenced by the development of physicsâaims to draw broadly applicable conclusions. But in fields like psychology, where context is everything, what if we focused instead on solving problems for individuals within their specific contexts, with generalization as a secondary goal?
To Aliceâs point about publishing research papers even when the hypothesis isnât successful, I think sharing quality datasets instead of papers is more valuable. It lets others build models and draw their own conclusions from it, separating data collection from interpretation and opening up more opportunities for scientific progress. Moreover, much of the data is already available to big tech companies, which I believe should establish data trusts to make this data accessible to qualified researchers.Â
While agreeing that âpushing everything toward a general space doesnât really workâ and âestablishing a data trust makes a ton of sense,â Alice argues that the greatest value lies in a combination of datasets and research papers, not one or the other. In her new role at Smart News, sheâs exploring ways to help people deeply understand information from news articles, suggesting that a similar approach could be applied to academic papers.
The unbundling of data from interpretations is underway
A recurring theme in my conversation with Alice, both in scientific research and media, is the importance of separating data from the stories, or interpretations around the data. By doing so, others can draw their own independent conclusions, potentially accelerating our progress as a society. âYou as the writer, you as a narrator, you as a scientistâŠyou have an interpretation of a thing, and if you provide the interpretation and the storyâthe pieces that you went through that got you there, they could be other articlesâŠthey could be conversations you had with other peopleâall that information, then somebody could then generate their own separate story from it,â Alice says.Â
At Every, weâve been playing with this theme through a couple of our recent experiments: TLDR, an AI tool that generates 3-5 minute podcasts from meetings you missed at your company, and Extendable Articles, an essay format where readers can interact with the underlying source material for an article. As Alice and I discussed these ideas, she wondered how to maintain the essence of a story across different formats and lengths. She also has a keen eye on how AI is democratizing the means to create new forms of media like podcasts.Â
You can check out the episode on X, Spotify, Apple Podcasts, or YouTube. Links and timestamps are below:
- Watch on X
- Watch on YouTube
- Listen on Spotify (make sure to follow to help us rank!)
- Listen on Apple Podcasts
Timestamps:
- Introduction: 00:00:59
- Everything Alice learned about growing an AI startup: 00:04:50
- Aliceâs thesis about how AI can augment human intelligence: 00:09:08
- Whether chat is the best way for humans to interface with AI: 00:12:47
- Ideas to build an AI model that predicts OCD symptoms: 00:23:55Â
- Why Alice thinks LLMs arenât the right models to do predictive work: 00:37:12
- How AI is broadening the horizons of science: 00:38:39
- The new format in which science will be released: 00:40:14
- Why AI makes N-of-1 studies more relevant: 00:45:39
- The power of separating data from interpretations: 00:50:42
What do you use AI for? Have you found any interesting or surprising use cases? We want to hear from youâand we might even interview you. Reply here to talk to me!
Miss an episode? Catch up on my recent conversations with star podcaster Dwarkesh Patel, LinkedIn cofounder Reid Hoffman, a16z Podcast host Steph Smith, economist Tyler Cowen, writer and entrepreneur David Perell, founder and newsletter operator Ben Tossell, and others, and learn how they use AI to think, create, and relate.
If youâre enjoying my work, here are a few things I recommend:
- Subscribe to Every
- Follow me on X
- Subscribe to Everyâs YouTube channel
Thanks to Rhea Purohit for editorial support.
Dan Shipper is the cofounder and CEO of Every, where he writes the Chain of Thought column and hosts the podcast AI & I. You can follow him on X at @danshipper and on LinkedIn, and Every on X at @every and on LinkedIn.
We also build AI tools for readers like you. Automate repeat writing with Spiral. Organize files automatically with Sparkle. Write something great with Lex.
Get paid for sharing Every with your friends. Join our referral program.
Find Out What
Comes Next in Tech.
Start your free trial.
New ideas to help you build the futureâin your inbox, every day. Trusted by over 75,000 readers.
SubscribeAlready have an account? Sign in
What's included?
-
Unlimited access to our daily essays by Dan Shipper, Evan Armstrong, and a roster of the best tech writers on the internet
-
Full access to an archive of hundreds of in-depth articles
-
-
Priority access and subscriber-only discounts to courses, events, and more
-
Ad-free experience
-
Access to our Discord community
Comments
Don't have an account? Sign up!