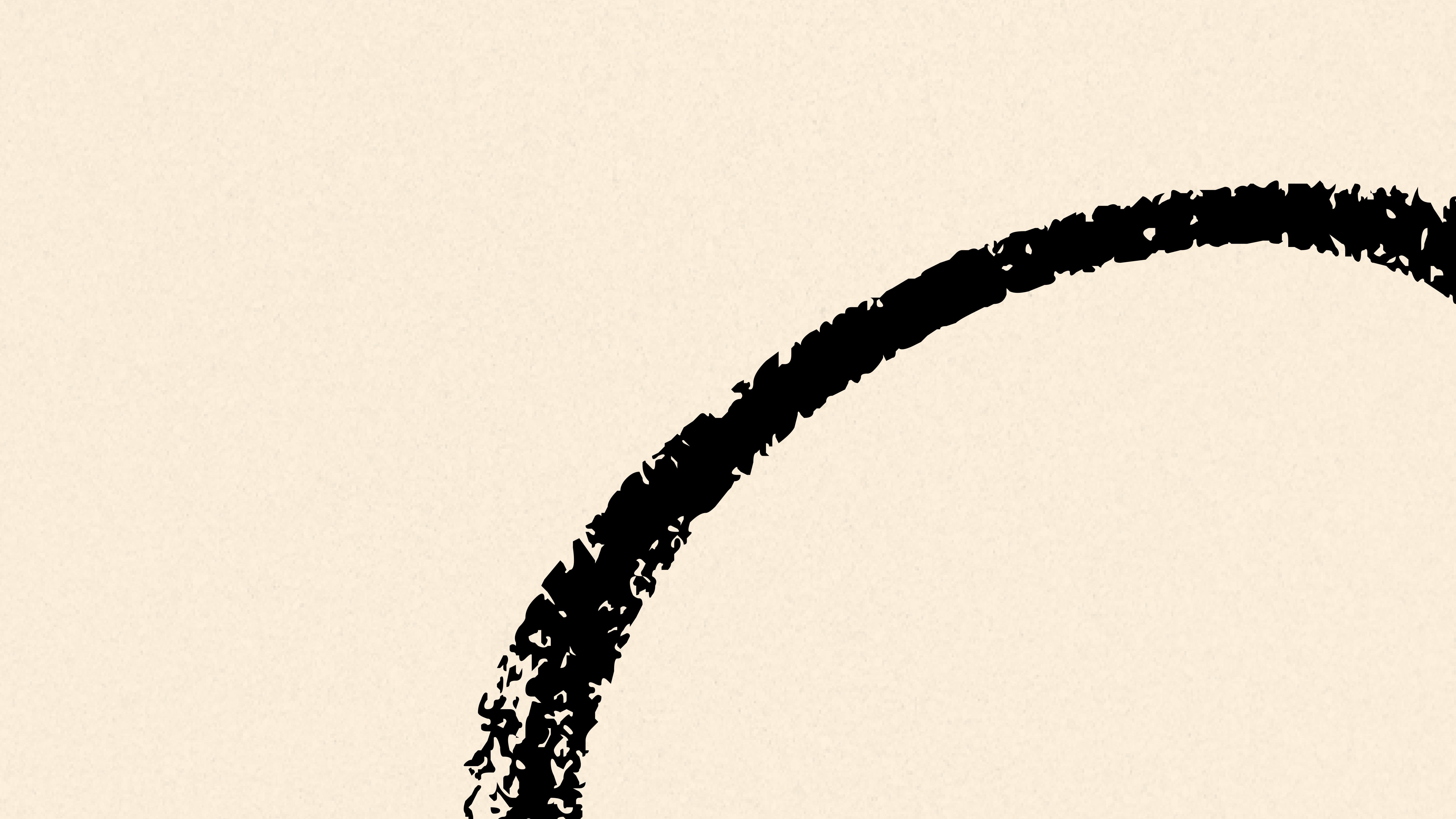
The transcript of AI & I with Alice Albrecht is below. Watch on X or YouTube, or listen on Spotify or Apple Podcasts.
Timestamps
- Introduction: 00:00:59
- Everything Alice learned about growing an AI startup: 00:04:50
- Alice’s thesis about how AI can augment human intelligence: 00:09:08
- Whether chat is the best way for humans to interface with AI: 00:12:47
- Ideas to build an AI model that predicts OCD symptoms: 00:23:55
- Why Alice thinks LLMs aren’t the right models to do predictive work: 00:37:12
- How AI is broadening the horizons of science: 00:38:39
- The new format in which science will be released: 00:40:14
- Why AI makes N-of-1 studies more relevant: 00:45:39
- The power of separating data from interpretations: 00:50:42
Transcript
Dan Shipper (00:00:59)
Alice, welcome to the show.
Alice Albrecht (00:01:01)
Thanks for having me.
Dan Shipper (00:01:02)
So for people who don't know, we've been friends for a long time. You are the founder and CEO of re:collect, which you recently sold to— What company bought you?
Alice Albrecht (00:01:14)
SmartNews.
Dan Shipper (00:01:15)
SmartNews and I'm excited to have you on here because you're just one of those people in AI that's actually been doing it for a really, really long time. And you've seen many different AI summers and winters. And I just find you to be incredibly smart and thoughtful and have built a business in the space that that you sold— You said it was an acquihire, so I just want to get into. what happened in the business you learned about running a company in this era of AI and kind of what's on your mind now. And I'll say for people listening I really liked re:collect as a product and we have not caught up since the acquisition. So, we're going to just do the conversation that we would normally do just hanging out together. So welcome to the show, give people a little bit of an introduction to the product and the acquisition process. And then we'll take it from there.
Alice Albrecht (00:02:10)
Yeah well, I'm excited to be doing this. We have not gotten to catch up, so this is good. So yeah, re:collect was—which is interesting to talk about it in the past tense now, but it feels, bittersweet. I'm proud of what we did and I'm excited about it, but yeah, it's hard just because I sat with it for so long, which I'll go through sort of the journey of building in this time of AI and how everything feels really new. I don't know if time feels like it's accelerating faster than it would have if I built this company at another time. If it was a software company five years ago with no AI, I feel like it would have been a different experience or a different journey. In any case, the re:collect was really focused on knowledge workers. You got to try out different versions of it over the years, but our main goal was, can we take all the stuff that you're consuming, could we connect it for you in this really personal way that your mind would? And then how do we make use of that for knowledge workers? So, we started out with this tool and we had a few different interfaces over the years we worked on it, but you could recall what you were thinking if you were doing writing or research. This is sort of pre-ChatGPT times. And then earlier this year, wow, yeah, this calendar year we had shifted into, don't tell us what you're thinking about—tell us what you want to accomplish. And then, we'll bring all the materials to you. We'll do the synthesis for you as needed, or we'll sort of alter those materials in a way that's useful to you as a knowledge worker. And everything we were doing was really aimed at how do we make all this stuff that we have access to accessible and useful for you? With the goal of enabling human intelligence really rather than the machine intelligence piece of it.
Dan Shipper (00:03:56)
I just resonate so much with that mission or that goal. That’s one of the things that got me so psyched because I'm a little bit more of a latecomer to AI than you. And so one of the things that got me psyched about it is, I feel like I've been such a nerd for tools for thought or technologies that expand the way we think or the way we see ourselves or the way that we create things and what we understand ourselves, all that kind of stuff. And it just feels like there’s so much there and you were playing in that space and, I'm kind of curious, what did you learn about trying to build a business there that you didn't know before?
Alice Albrecht (00:04:50)
Yeah, I think— A couple of different things. When I started— I left my full-time gig to work on this in 2019. So things were really different at that point. BERT and ELMo, these early models that came out, it’s all opportunity in the ideation phase. Okay, we have a capability, what do we build with it? What's useful in the world of this capability? What's possible now that wasn't. That was an interesting process. It took, I'd say, a year and change probably with Covid to be stuck in there and all of the chaos that surrounded that. But the journey of building a business for it, I think was, it had these separate phases. One of them was, I would say, we have this really nascent technology. Nobody really understands it. I'm trying to explain what's possible to do with it, but the models really weren't quite—this is GPT-2 maybe—they weren't amazing yet. I could see potential, but that was hard. I think once the models got a bit better and especially after ChatGPT launched, everybody woke up and was like, wow, this is really cool.
And then we had this middle phase as a company where I still was a bit skeptical about, okay, do we shove this into a product and have it generate things? Do we trust the models to do that in a safe way in the same way? And I thought, no, and so we had this middle phase of company building where we were launching a consumer product. We wanted it to be something that was useful for people, but also navigating all of the, I guess, oh, we could do that, but is that really in line with what we want to do? Or is that just because something new came out with a model and we could veer in that direction? That was interesting to navigate. I think, on the whole, now having gone through the whole cycle, if I had to do it over again, or had the opportunity to do it over again, I would have launched the product much earlier. I would have let the messiness be okay with customers and just see what happens with that. And I think we did a bit of trying to protect that and say, okay, we want to have this integrity. We want it to feel like it's a really seamless experience. We don't want them to really feel the weirdness of using these AI tools. And then the third phase is really as these tools are way more widely adopted, they're in everything. There's this huge explosion in this space trying to think about, okay, what is the actual application other than retrieval? Because retrieval was such an easy one. And we were doing a lot of retrieval stuff in the beginning, but then what happens after that? Once you retrieve a bunch of things, what is useful to do with those things? Yeah, so it was an interesting tri-part journey, I'd say.
Dan Shipper (00:07:38)
That's interesting. I definitely think that's such a common thing and it's so hard. It's easy to say and hard to actually internalize. It's just, ship something faster that you're not as proud of and just seeing what happens, can be very scary because obviously you want to make the best product possible. And it's a weird thing. Sometimes making the best product possible is starting with doing the messy thing or whatever. It's just weird. It's a weird thing to get into your brain. I think one of the things I'm interested in is, when you started it was like BERT and maybe GPT-2-ish days, what made you be, okay, this is happening now?
Alice Albrecht (00:08:33)
I think I willed it to happen, honestly, even going out, we did the typical VC fundraising process, too, and so, there was no moment where I was like, okay we finally hit it. I was like, no, no, this is going to happen. Come hell or high water. Even if I have to make this true—so yeah, I feel like it was really convincing other people that no, no, no, these embeddings I'm talking about are really, really important. And that was hard.
Dan Shipper (00:09:03)
Okay. And what do you think about— You wrote an article in Every a while ago and I read it and edited it a really long time ago, so I only have some of it in my brain, but basically I just feel like you have a really strong and clear thesis for how humans and AI can work together and what that should look like or could look like. And it's, it's a sort of cyborg hypothesis and I wonder if you could lay that out because I think it's a big question on people's minds. Where are we going if we're going to use this as tools? How does it work? And also keep us being humans and employed. And then I'd love to talk about how that has shifted for you over the years building this company.
Alice Albrecht (00:09:55)
Yeah, and it certainly has shifted, which has been interesting to kind of catch myself in between you saying these statements in the world and then something changes and you're like, oh, you'll have to readdress or reassess the whole situation.
So the cyber piece I'm still pretty hell-bent on. I think this has been my schtick for a long time now, coming from human studies to working with machines. I have this belief that humans are really kind of amazing creatures and that, if we're going to use the technology, it should be to augment them rather than to just build an AGI. The goal for me is not some sort of super intelligence that works without us. How do we start to connect this technology to humans? I'm really finding those touch points. I think knowledge is one of those. So if we think about knowledge work in general, a lot of it is consuming, distilling information, understanding and really kind of propagating that information out to other people, other knowledge workers in those systems.
And so, for me, I think a lot about how we can use AI or machine learning or these other technologies to make that process easier for humans so they can do the creative piece. And I think that's where we get to how do we all stay employed? I'm a big proponent of lowering the bar, raising the ceiling, I guess. So, what's possible we don't even truly understand quite yet. But if we can find the touch points where it makes a lot it makes that human work a lot easier. And I don't think we're quite there. I don't think chat is the right interface for this. I don't think the models have the right context for it. I say this again and again, I still think we're going to need some sort of biometric feedback piece in there to really make this work. And in that case, it's about finding where humans are not deficient, but where they kind of struggle from their evolutionary constraints, where technology can come together. And it's not creating artificial eyes. That's pretty cool, but that's not exactly my thing.
Dan Shipper (00:12:05)
Yeah, that makes sense. I was laughing earlier because I feel like we have this recurring sitcom or soap opera or something that every time New York Tech Week comes around, we're on a panel together. And the first year it was me and you and it was right after ChatGPT launched it and it was a couple of other people. And, yeah. I won't say who or whatever, but there was one person on the panel where me and you were just looking at each other the whole time. You're like, what is he—? What did he say?
Alice Albrecht (00:12:32)
What is happening here?
Dan Shipper (00:12:35)
Yeah, that was really fun. And then the next year we were on another panel about AI and creativity or something like that. And we were on different sides of the debate because we're talking about chat interfaces and whether or not chat interfaces are the future of interactions. And like you said just now, you think the answer is no. I was defending chat and I'm curious how that position has evolved for you and, if you could refresh my memory, why do you think chat is not the right interface?
Alice Albrecht (00:13:10)
Yeah, I’m trying to remember the argument I made on this panel. I probably won't be able to recall it exactly and I would also love to hear how your position may have changed over time. But, I think, language is a super powerful tool. We're using it right now to communicate with each other. We consume it in this way. I don't think that it is the most natural way to access things like this. I think we've actually moved farther in the search and probably since that panel too, with all of this agent stuff happening, where we have this kind of thing that does work in the background for us, but it intuits what work to do a little bit more. And it accomplishes that without us saying hello, robot, please, blah, blah, blah, blah, blah and I think the only way I've come maybe a little closer to the chat piece is I've used it more. I find myself in new spaces where I'm like, okay, I just started a new company, I'm in a new area where I'm thinking through things. It has become a little bit more useful for me to say, okay, I'm thinking about this, what are the pros and cons? And right now I have colleagues that are in Japan. We're 13–14 hours removed and so it's helpful for me in that sense. It's still not my main interface, though, so I think I'm doing more coding too. And so I think the main use for me right now is code. And that's sometimes chat.
Dan Shipper (00:14:45)
Are you using Copilot, Windsurf, or Cursor? Or like what are you doing?
Alice Albrecht (00:14:50)
So I'm coming back to Cursor. I had used it really early on. It was really buggy. And I was like, this is ruining my get-up. I can't use this. This is terrible. I'm coming back to that. So I use VS Code. I have Copilot for all the regular things. I do some chat. I really like the Claude Artifacts. That has been a really big game changer for me. And this is where maybe my chat argument does hold up. So it generates these—basically—little maps of things. You can have it do these mermaid diagrams as one of the artifacts. And I think really visually I draw things out a lot. I'm famous for having this remarkable thing with me everywhere I go and sharing these—I think I put these in one of my articles I published. This is how I think. I don't really think in this chat back and forth situation, so generating that has been interesting for me, too, as a way to collaborate in a non-text way.
Dan Shipper (00:15:47)
That is interesting. So for me, when I talk about chat, I would include voice, video and the sort of back and forth. But maybe it's more properly limited to just text and I think probably the reason I like chat as interfaces is I'm just so verbal. So that just makes a lot of sense. But if I try to turn my own personality into a general truth about what's good, which I think is typically how things work if I try to do that consciously, I think the reason that I that I like chat as an interface is it allows you to push forward along many different dimensions simultaneously where a lot of other software interfaces are either on or off. Or it's along one axis at a time or something like that, which is quite useful. Sometimes for refining something or for processes that you're doing where the dimensions that you're improving along are really well known and really well understood and it's sort of repetitive but then there are a lot of other processes, particularly creative processes, where you're trying to explore space along a bunch of different dimensions and you don't really know what the dimensions are beforehand. And I find that to be quite good for that exploration process and for the refinement process of, okay, you’re producing this thing, now I want you to push it in this way or define what that was before. But now I see it. Now I know.
So a really good example is we've been incubating products inside of Every, which is really cool. I would love to tell you about it. And one of the products that we incubated is this product called Spiral and it helps to automate a lot of the repetitive creative tasks that you do if you're running a company or you're a marketer or you're a creator. So an example would be, for this podcast, I have to take this podcast and turn it into a tweet to get the episode out. And that's a very repetitive process because I kind of have a format that I know works. I have a good first line and then I have a couple of bullet points about things like, what are the key topics that we discussed that I think are interesting or whatever. And I realized that Claude is really good at doing this. If you give it a few short prompts and you give it a bunch of examples of podcast transcripts tha turned into tweets, then it can do that over and over again. And it gets you like 80 percent of the way there. So we built Spiral, where it's basically a few-shot prompt builder. So you can make a Spiral for turning podcast transcripts into tweets, or you can make one for turning blog posts into LinkedIn posts or turning whatever release notes into a product announcement or whatever and I'm giving you this very, very long-winded explanation because one of the things that we found is in the in the Spiral interface, when you have a Spiral—let's say we take my podcast transcripts to tweet example—you just paste your transcript and you press run and then it gives you a bunch of example tweets that you can try. And one of our biggest pieces of feedback from people wanting an improvement is, I just want to chat so that I can say, this example was good—do more of that. You didn't really think that that would be the case. We had ideas for, maybe we could do sliders or really what you should do is go back into the Spiral creation flow and modify the prompt a little bit and make the prompt a little better or something like that.
And what we're finding is the natural thing to do is sort of you run a company, it's sort of when someone that's reporting to you comes to you and it's like, I did the thing that you asked for and you're like, okay, this is great, but here's a couple of things I need you to do. That's a very natural way to kind of push something in a sort of multidimensional, somewhat unknown space. Does that make sense?
Alice Albrecht (00:20:25)
It totally makes sense. And I think that using AI today is really good for this. Here's the first draft. Be it creating the tweet for me, writing some code for me, whatever, any kind of production thing. In that process though, I think there's this very interesting teacher-student relationship, which shows up. I think there's some new stuff around this, around training tiny models, based on big models, and those are really cool.
But I think it's interesting—there's two interesting pieces there. One is the human in the loop. And this, I think we do usually agree on, which is the human creative piece of this where you have the judgment and saying, nah, this one's not quite right, but here, change this one. Or, I can choose from this list. Awesome. The Claude model or whatever using it doesn't have enough information or intuition or something that seems a little bit fuzzier in there to choose. Okay. This is the best one. Just roll with it. I think that's going to continue to be the case.
And then the other piece in there, though, is this knowledge sharing. So in a sense, when Claude or whatever model you're using outputs, these sweeps for you get a little bit of a peek into how it's, quote unquote, thinking. It generated these things. You can see these, it got it kind of right. These are kind of wrong. You could give it feedback, but you're getting insight into that. And when you give it data, it's not learning in real time with the few-shot pieces. But maybe at some point it could and I think the more that you get a system that knows a little more about what and how you— I don't even want to call it preferences because it's so squishy. You can't learn these preferences easily but the more that you and the model can get to a shared understanding of what the other thing knows and can fill in the blanks. You can say, oh yeah, you wrote that one, but you forgot, or you didn't know, or, by the way, actually add this piece because this is critical in there. So I think this way of interacting and that even to me, I think the original like chat interface of ChatGPT where people are like I can talk to the thing and everyone likes chatbots. This is already beyond that when it creates artifacts. It's not a conversation. It's not like, hey, I think you should generally do. It's like, no, no, here's your thing. Here is your output and that's gotten really interesting.
Dan Shipper (00:22:49)
Yeah, that is, that is interesting. I was arguing against chat interfaces and then the product that I built was like a chatless interface, which is, I think it's your point, that reducing that down is actually— It can be helpful. And then we need to bring it back in some form, but there's interesting trade-offs to that. And the form that we're bringing it back is definitely not a totally general interface. And that's the only way that it can compete with Claude. You can do the same thing in Claude. It just has to be very specific to your purposes. I've been thinking about doing something. And something about the shape of it makes me feel like you would be into it or have interesting thoughts on it and whether it would work and what I should think about. So, I'd love to talk about it. You said something earlier that reminded me of it, but I forgot what it was. So let me just lay it out for you. I'm pretty curious.
Okay, I don't know whether we've talked about this before but I have OCD. One of the things I've been thinking about or lightly trying is I wanted to see if it was possible to wear a WHOOP, so to take my WHOOP data and be able to label from the graphs whether or not I was experiencing OCD on a particular day.
And so far, my answer to that is kind of, maybe. But, it actually probably needs more context to know. Because what a spike means on a stress draft and a WHOOP and a WHOOP can mean a lot of different things depending on what's going on in the background. And what's really cool about these models is now they know enough to be able to take the context and use that. And so, I'm not far enough along yet like another thing I'm going to try is I'm starting to do daily, two-minute video journals and there's this emotion-labeling AI called Hume and I'm going to see that it can label it. So, I don't know tha, there's some interesting things there that I think about. I feel pretty confident there's some combination of data where I can get from data to label to be, yes, he's having OCD symptoms today or no, he's not. And when I'm there, the big question to me is, will it be possible to predict, let's say a day in advance, whether or not I will start to experience OCD or whether or not I'm in a sort of OCD phase, whether it'll go away because I think once you can predict it opens up lots of interesting things and so my thought for how to do this is to basically do a bounty. And do $10,000 if you can predict my OCD, here's a data set and let anybody—because everyone you can have—you can use o1-Pro for $200. Anyone can do this basically now in a way that they couldn't before. And a) see if that works and then b), maybe build that into a sort of Kaggle but we're now anyone's a data scientist. And there's probably a lot of things that you would be into about this, but or have thoughts on but the thing that I'm kind of interested in— I think making predictions about and maybe we talked about this a bit, but I think making predictions about whether or not I'll have OCD is a form of science, but it's a form of science that like a scientist would never do because it's an end-of-one experiment and you're not actually looking for a causal explanation. You're just predicting so it's completely taboo to the establishment of research, but it's completely incredibly useful. And I think now doable where I think we should be doing a lot more of these end of one things and pursuing more predictions over underlying scientific cost explanations. And I just thought that that would get your brain going and you'd have interesting things to say.
Alice Albrecht (00:27:13)
Totally. And I appreciate you reigning it in too, because I have all of these pieces I can talk through. Yeah, I think the end of one thing is interesting because, if you came to me and you're like, I am in great pain from this. You were like, I am suffering. And as my friend, I would be like, gosh, how do I help you? And if I thought I could slap together a model, you got your WHOOP data—great. It'll take me whatever amount of time, but I could just do this. I wouldn't actually go to one of the large language models straight away. My brain would say, we need a predictive model. We need to understand the data sources. So I think it would be an interesting combination of looking at the actual research. So there is research out there on OCD—I'm not an OCD researcher.
Dan Shipper (00:28:01)
Not physiological, as far as I can tell. There's no physiological marker research.
Alice Albrecht (00:28:06)
Fascinating. That is actually the most interesting.
Dan Shipper (00:28:09)
And I did buy an at-home EEG to see if I could do stuff. It's all like MRI stuff. So it's not usable by me.
Alice Albrecht (00:28:18)
That's so interesting. That's a whole other podcast in and of itself. Why do we not have biological correlates for a thing that is fairly common in the population and probably has at least a heart rate difference? Something has to change.
Dan Shipper (00:28:31)
That's true for pretty much all mental mental illness—there are no biomedical markers, biophysical markers. It goes from being psychiatry to being neurology. So certain things used to be mental illnesses that are now just neurological issues. So yeah, it's, it's very— All of psychology is basically built on self-report as far as I can tell.
Alice Albrecht (00:29:03)
I know, I know. It's true. Yes. I could make some easy predictions off the bat and go, okay, heart rate data, probably important as a predictive measure of this. If you can give me labels, it's a question of how many labels do you need to make this work? Is it 700? And then you're suffering for a really extended period of time. I don't know how long these episodes might last and so I think if I were going to start from this, the way I would use these models though, is to help comb through literature and say, okay, I'm looking for this, pretty specific information. How do you help me jump into a field that it's not mine? But it's adjacent to when I know enough, but then also help me build the model—help me think about how to build this predictive model. Here's the data that I've got, or the type of data that helps me. Don’t build an LLM, but build a model that has predictive power that is well beyond what the LLM would be able to do. Because the LLM isn't really trained to be a predictive model. It's trained to predict language and it has reasoning capabilities from that. But there's lots of other models out there that would take all this data, normalize it, put it together and say, okay, now we can build a basic predictive model, which would be a great Kaggle exercise in and of itself. I don't think it exists.
But so I think the piece that's interesting is translating if you have a video daily check-in or something translating that into something that is useful for this kind of model. Fei Fei Li did some really interesting work a really long time ago. She's sort of the mother of AI, I guess we can call her. I don't know if she would care about labels but she had a fascinating paper and it's really a long time ago now, but it was taking different data inputs. So it was video data, people talking and they could predict depression or the onset of a depressive episode and it was the facial expression, it was the cadence of their voice, the tone, all of this data lives in this. And I don't know that there's anything correlated for OCD but I think you could use these models to transduce that information into some meaningful signal for a different model.
Dan Shipper (00:31:18)
Yeah, I think you're right. So basically the cool thing about Hume is it turns your video into the equivalent of emotion embeddings, which is pretty cool. And so each frame is for your voice and your facial movements and whatever it outputs an embedding that represents where it thinks you are in the emotional world—embedding space. And so yes, that can be used and fed into some sort of predictive model, but I don't know.
Alice Albrecht (00:31:50)
And if you had it over time. So if this model ran frequently enough that you get time series data that's meaningful, even if it was once every 10 minutes or something, and you could aggregate over your group data, you could bring it— I could totally see how you could put these pieces together and then say, okay, I can label for you when I'm having these episodes. I can label for you when I feel like you have a sense when they're starting to come on vs. fully in the middle of this vs. it's at the tail end. I think it's totally possible.
Dan Shipper (00:32:22)
I built this little app for myself where I do retrospective assessments. So in the morning I'm retrospective: How was I yesterday? What were my symptoms if I had any? And then I upload a screenshot of my WHOOP graph and then I do a two minute check-in. I don't say how I'm feeling, but I just talk into the camera about what I'm going to do that day or something like that. Because I don't want to give away the label so you're saying maybe I should be doing more momentary-type data gathering if I'm in the middle of something to try to catch it if I can.
Alice Albrecht (00:32:55)
Yeah without aggravating the symptoms, obviously. Because I can see how that could spiral. No pun intended, but I don't really want to get into a weird thing. What if I can keep doing this thing.
Dan Shipper (00:33:07)
Well, one of the funny things is the treatment for OCD—one of the big treatments—is exposure. It's for science. It's exposure for science.
Alice Albrecht (00:33:18)
Exposure therapy. Oh gosh. So I think if I was going to model any kind of, I don't know, psychological thing that was happening periodically, I would want the data from right before. In the middle, so you've confirmed it's true. And then at the end and see, okay what are the shifts and changes that happen? And then I think what's interesting with the models also is you could get other contextual data that you don't have. location is an obvious one where are you generally in the world? Your calendar. Who are you talking to? What are you doing? What is your workload? We’ve got all these sleep predictors. We'd like the WHOOP to be good for saying, did you sleep well the night before? It does all these other analyses.
Dan Shipper (00:34:04)
How would I get that into a non-LLM though? Let’s say I have a calendar. Let me tell you what I've been doing. Because you're probably gonna laugh because it's so dumb, but, what I've been doing so far is just taking the images and the labels and just throwing them into Claude and -o1 and being like: Here are labeled images. I want you to come up with a set of rules to classify whether or not I'm having OCD or not. And it's currently not very good.
Alice Albrecht (00:34:36)
I wouldn't expect it to be. I would be pretty shocked if it was. You might have opened up a portal into some other whole other research field. It would actually be fascinating if it worked. So what I would say is what you need for a predictive model. If you need to get all of these signals as features. And then for something that has temporal dynamics to it. I think you can get it off the Whoop I think I tried at some point.
Dan Shipper (00:35:01)
The daily summary, but not the momentary statistics. I think I created a data pipeline. Because I can just take a screenshot and then feed it to Claude and Claude will be like, here's what they make an SVG that matches the line of the WHOOP graph and then turn that into a time series or something. I think it's all time series.
Alice Albrecht (00:35:20)
Yeah, that's actually really cool. I didn't think of that. So that's very clever as a way to get this data. So what I would say is, you need temporal data that's fairly time-locked, and then you need to convert all of these pieces into features. So you have your video that you're taking a certain time. And so you would need to do some processing on the video to pull out and you can use human sounds to do that. But pull that out, have the timestamp associated with it, have the actual time series of the WHOOP data, and have as much queued up to these timepieces. And then those are features that go into a basic classifier and it depends on the test that you can. There's lots of different ways to classify data, but all you're doing then is providing information to the model, a non-LLM model to say this or this. And you kind of have a binary classification, unless you predict, oh, it's oncoming. It's happened. It's fading. And that's also not a terribly difficult classification task. So my bet is that if you asked Claude, if you said hey, I've got this data turning into a time series for me, here's my video data with timestamps, translate that into features and then write—probably in Python—a model for me, a predictive model, a basic one, and help me plug these features in, I think you might be able to get somewhere with that. I think the problem is asking an LLM to do the predictive work because it's not really set up to do that. It doesn't execute other models.
Dan Shipper (00:36:48)
That's interesting. I mean, you can use an LLM, as a basic classifier model. So you're just saying that it's classifying a different kind of sequence and so the features that it's going to pay attention to in a text sequence are just going to be different from the features it pays attention to in a OCD time sequence.
Alice Albrecht (00:37:12)
Yeah, and depending on— I know there's multimodal aspects to the models now. I actually don't know that LLMs are the best for classifying certain things. And so even now I'm still saying, okay, BERTopic is a different kind of model. It's a large model, but it's not OpenAI or Anthropic models. Models that are specific to pulling out tags or categories or things like that from text data are actually still a little better than just throwing it into the big model. They're more specialized, so that might change as these models get better. But I don't think that these are in and of themselves better than other machine learning models that are really meant to classify, especially time series data.
Dan Shipper (00:38:04)
That's very helpful. Basically, what I feel like I'm doing right now, which I didn't even realize I'm doing. I'm trying to kill a mosquito with a rocket launcher or something like that.
Alice Albrecht (00:38:14)
Yeah, totally. But it can help you build the right ones, which is cool.
Dan Shipper (00:38:19)
Yeah. That's interesting. Well, I guess I want to pull us out of the specific details of this particular engineering problem and more to the higher level of— I'm thinking about how this might change science and how this might change how we do science. And I'm curious about your thoughts there.
Alice Albrecht (00:38:39)
Yeah, I think it's already probably fundamentally changed how we do science from a knowledge standpoint. Being able to comb through all of that information and pull things out has saved graduate students and postdocs and these people countless years of their lives. I don't know, but that part feels incredible to me. So that’s one way we change science. The second way will be simulating. So the data is huge to train these models. We have all the hardware for these things and they're getting good enough at simulating data. If you have a solid existing data set, you can say simulate lots more data like this. And that is incredible if you're trying to understand the possibility of space as a scientist and kind of winnow that down, and it's hard for humans to do. We can make predictions. We can synthesize data, but keeping in mind all of these different kinds of possible future states is really, really, really hard. So I think it'll change science in that way. I don't think we're going to lose scientists. I don't think we'll really have AI scientists. I think there is a problem around— I think humans still do a lot of the hypothesis generation, which is a lot of the science and still thinking critically about, okay, what kind of data are we even trying to get to understand this? I’d like to even get started on this space or this hypothesis.
Dan Shipper (00:40:14)
That's interesting. I mean, that's sort of what I've been thinking about. Okay, in a world where the data is actually the really important and rare thing, and you want to get really good data, is the substrate of a scientific paper actually like that? Is the substrate of a scientific paper actually the right format to release science in. For example, there's a lot of open-data type type pushes right now and is the idea of doing a 16-person study actually at all interesting or is should we be basically should the project of science be to gather as much as much good data sets about problems that we care about as we can and to aggregate them and then allow any scientist to build models on them rather than writing papers about them. I mean, papers are fine, but, in general, there's a huge overproduction of papers and huge underproduction of usable, good data and then if it moves to production of good data and basically building good predictive models, then, for me, it feels like— And I've been on this soapbox for a little while. For me I feel like we should be actually going for predictions first before we do the causal explanation stuff, especially for things like psychology or psychiatry where the causal explanations are really, really, really, really, really, really complicated.
Because if you can avoid high depression or predict my OCD or predict what intervention is going to work, it's life-changing. And if you have a good enough predictor, maybe the explanations are in there somewhere so that's kind of where I've been thinking about okay, science may change and it may have to change in this particular way where it looks a lot more like engineering. It looks a lot more like data gathering and model building and maybe a lot more like this. I think you'll have opinions on, which I would really like—maybe a lot more, like the shift that we went through from symbolic AI to subsymbolic AI. Maybe that shift where we were trying to find rules and logic—This is not for you, it's for people listening—to rules and logical formulas like defining what intelligence or intelligence decisions were. And then we were like, actually, why don't we just throw a bunch of data at a model? And it'll figure out that shift. Maybe we need to apply that to the rest of science and a lot of other areas of science and the world. And that would actually be really, really helpful.
Alice Albrecht (00:42:58)
I like the analogy that you’re drawing between sort of the production of papers right now as being the more symbolic approach where we've got this really specific thing that somebody is asking a question about. They gather small data. Usually, it really depends. Science is such a broad field. So I also don't want to shoot myself in the foot with, oh, there's so many kinds of science. My space that I used to work in is small-end studies. Maybe 10–20 people. It's not huge drawing conclusions from that is hard and it's hard to replicate. But if we say, okay, the more symbolic pieces of this are rules-based and we're saying we've learned something really, really specific about this very narrow thing, and now we can create a rule around that. And then the next person comes along and says, I follow this rule because you published a paper and moved on. And so I think a couple of things in there. I do think we should be publishing a lot more data. I think that the data asymmetry between what people can use to train models, let's say the large language models. All this text on the internet. That was a huge unlock. Now that we have the compute power to deal with that amount of data, we have the compute power though. So if people release datasets in other domains, it would be potentially this very synergistic and maybe sort of almost not a linear, but maybe a log scale improvement and things. The more we get this competent combinatorial power of lots of different pieces of this broader data set that everybody gets a chance to see. And so I do think like as time goes on, as we get more access to data and compute, scientists do become more computational by nature, even if they never were before, they have access to the combinatorial tools in their toolbox to answer questions and then I still think it's useful to publish papers.
It's useful to get this information out there. The problem has always been, we only publish certain things. And so we're not getting any information around what's failed or what's been tried. And so we really are only getting a very weird and skewed slice of science as it were so I don't think we need fewer papers. We might need more papers. And if we have a tool to help us sift through those, maybe it doesn't matter but also, if you do make data available, there are people that are able to use it for lots of different things that you may never have thought of the hard part at speaking as a former scientist comes in that the way that you chose to collect that data is incredibly important. What was the setting you were in? What was all the way down to the refresh rate of the monitor often and so making this transferable is hard.
Dan Shipper (00:45:39)
The thing is, I feel like there's this big push to generalization. That's the whole object of science as we've construed it, basically because of Newton. And in general, I think what we find in cognitive science or psychology or whatever it's so contextual that every time people have claimed to have something really, really generalized, not every time, but a lot of the time, it's much weaker than we thought. And it's not as reliable as we thought and rthen people get angry at scientists and it's like, well, maybe we're setting a super, almost impossible task and we're asking the wrong question. And that's why I think the end of one thing is more interesting because, sure, the context really matters. Because I'm one person, please— I'm in my context, solve for my context, and worry about the realization later.
And the other thing that this made me think of I was talking about papers and data sets is one of the nice things about shifting away from a paper as as the thing that you publish, is that you don't publish papers where the hypothesis doesn't bear out, but if you're just publishing the data set, as long as the data is quality, you'll publish it. And then the model is you unbundle the model, which is the kind of conclusion apart from— Which I think is kind of interesting and then it also, if the thing that's really important is the data, it's really stupid that researchers have to get grants to study five undergrads when Facebook has all of the fucking data that you would ever need. And this is a family show, so we'll bleep that out. But I think big tech companies should establish data trusts where they will donate their data for qualified researchers to, ask questions and find out the answers—it's all sitting there. We just need to use it.
Alice Albrecht (00:47:51)
I think to be fair, some of this does happen. And this is the big argument around keeping open-source models. Academics can use these open-source models and they can build on them and they can do awesome things with them. The more you close those down, the more we mess with academics on this point. But I think, yeah, I think establishing a data trust makes a ton of sense. I think scientists can ask the right questions, pushing everything towards a general space doesn't really work. I don't think it works with AI either, to bring it back to this sort of modeling that we're talking about now. And I think the power is not choosing open data sets over papers, but the combination. So for me, something that I am thinking about a lot right now is from news articles, how do we deeply understand that information? And then how do we build things on top of that? I think with academic papers, it's similar. How do I deeply understand not the conclusions, maybe not the methods. They’re important, but if I were to take all of this information out of this paper. If I had access to the data in combination with that it would be really another level of understanding and even alternative hypothesis generation and testing. I could possibly do depending on the data set.
Dan Shipper (00:49:06)
Have I shown you Extendable Articles?
Alice Albrecht (00:49:08)
Oh, I think I saw it. It came out this week?
Dan Shipper (00:49:12)
It came out last week.
Alice Albrecht (00:49:13)
Last week. Okay. I started poking around with it. It looks super cool.
Dan Shipper (00:49:16)
Isn’t it cool? It's super cool. For people who haven't seen it, we built this little tool where we publish an article on Every that has a lot of original interviews and research. You can basically read the article. And then we also make all of the sources, all of the hours of interviews and articles and whatever that we conducted and found and read we make that available as a little chatbot so you can kind of go through and form your own opinion. Is that sort of what you're talking about?
Alice Albrecht (00:49:43)
Kind of, yeah. So if every news article came with that, it would be incredible. And there is a thread through here, actually. The stuff we were building with re:collect, where we were taking all of these pieces of information, articles you can read, whatever it was, connecting those and then generating something on top of that. We had a line back to what were the pieces that went into this? Right now on the other side of that. I'm trying to understand news articles that are coming through for the work I'm doing at SmartNews, but the deeper understanding that I get right now comes from how these articles are interconnected. And I'm creating that in the models then later, but with what you've made with this, you're giving me what is connected to this? Or, how did you get to write this article? So I feel like it comes from a couple of different angles, but the more you get those pieces, the richer the understanding becomes. And I think the more interesting things we can do with that.
Dan Shipper (00:50:40)
That is interesting. To generalize, I feel like one of the patterns that we're pulling out is— And this is something that I've written about before, but, and only in more specific context, there's all these places in machine learning, in science, in journalism, where for whatever reason, the story and the underlying data that were like used to create the story always had to be bundled, and that created a lot of problems and the solution is not to get rid of stories. The stories are also very, very important, but it is to say, we are now in a place where the underlying data is actually probably just as important as the story because it's now way more discoverable and legible than it was before without the authorial perspective and that perspective is still important, but it's important to present it with this other thing and we'll make way more progress as a society if we do that.
Alice Albrecht (00:51:47)
Yeah, I think that's absolutely right. From what you're saying, which I'm on board with you as the story creator, you as the writer, you as a narrator, you as a scientist, writing this paper, these are all ways of conveying information—it's a story in and of itself—you have an interpretation of a thing, and if you provide the interpretation and the pieces that you went through that got you there, whatever those are, they could be other articles, they could be thoughts you put together, they could be conversations you had with other people, that all that information, then somebody could then generate their own separate story from it, in a much quicker way too, than if they had to, I got to read the entire reference section of this paper and I got to comb through all of your last paper.
Dan Shipper (00:52:34)
It's no longer necessary. We don't need to do that. And that's so good because how much time have you wasted trying to understand some crazy paper that is really important, but you have to understand five background things in order to read it. And that’s not a thing anymore. It's crazy.
Alice Albrecht (00:52:50)
Yeah, it is crazy. And it's exciting. I'm thrilled.
Dan Shipper (00:52:56)
All right. We're almost at time. Is there anything else that you wanted to talk about or anything else on your mind before we end?
Alice Albrecht (00:53:01)
Yeah, I feel like this last conversation is something that would be fun to do more on, but I feel like we won't get the time. How does this intersect with the stuff you're doing at Every? Now I'm thinking about this media news space, I think there's not been much change in this space, which I thought would happen with AI, but I think it might be coming, and I'm not sure.
Dan Shipper (00:53:24)
Yeah, let’s talk about it. So the question, just to make sure I understand, is how does this unbundling of story and data that we think is interesting in science and also in media, how does that affect media? How does it affect how I'm running Every? That's what you're asking about?
Alice Albrecht (00:53:43)
And I think maybe one level up from that broader question is we've got all this stuff that's happened in the last couple of years with AI. A lot of it is text heavy, story heavy. We haven't seen a fundamental shift in media. Well, we've seen lots of generated articles and stuff, and people have mixed feelings on those, but we haven't seen a huge shift, and it must be burgeoning.
Dan Shipper (00:54:07)
Yeah, I think it's coming, and obviously we're to some degree trying to invent it, so I have some specific opinions— but I don't know if I'm right. I think in general I have an opinion on where it will go and then like some specific bets that we're making. So one of those bets is this extendable article thing, which I think in its current form is not good enough. And it's really interesting conceptually, but it's not something that a lot of readers are going to use all the time, and I want to get there and try to make it a standard. Another thing, we've created a new synthetic show from Every called TLDR. TLDR is a 3–5 minute AI generated podcast about your company — so about meetings that you missed. It's done with all of the writing and taste and storytelling ability of the writers and producers at Every, but it's about your company and we take meeting recordings and turn that into podcasts so that if you miss a meeting, you know what's going on and it's the first of hopefully many shows that we’ll end up doing. TLDR is about the meetings you miss. There might be a ‘how it's made’ type show that talks about a big product launch that you did, or a Sunday strategy catch up where it's, ‘here's all the stuff that happened this week,’ as you're drinking your morning coffee stuff like that.
So that's another thing, as storytelling gets cheaper, I actually don't think it replaces other kinds of storytelling—we're still going to watch movies—but it means that we can tell stories in places where it would be too expensive to tell them otherwise. No NPR producer is going to want to make a show for most internal meetings, but now that doesn't matter. Every company has a story and you can have AI tell that story and that's, I think, really cool. So that's another place that we're kind of excited about.
Alice Albrecht (00:56:15)
Oh, that's so fun. And I've been following the NotebookLM stuff too, in terms of the podcasts they're creating. I love the idea of saying no one would bother to create this, now we can. No one's going to sit in your meetings or whatever and be like, ‘now you're up first,’ that is really, really a neat application of that. I've been thinking a lot about accessibility of stories and how do you craft a story that is maybe distilled or shorter, but doesn't lose some of the characteristics of what you're trying to actually say? But either translate that to another language or make it audio, make it video, make it something that somebody that wouldn't actually— maybe they have morning coffee, maybe they don't— but maybe they don't read. They don't want to sit and read something. So I'm pretty excited about that, I think that will be a fundamental shift in terms of the cheapness of producing that piece.
Dan Shipper (00:57:11)
I agree. There are all these classic books that can become more readable, more interesting, more visual, more audio. I've been rewriting this Platonic dialogue as narrative nonfiction, and I threw it into Sora, and it made a movie out of it and I was like, this is crazy.
Alice Albrecht (00:57:34)
Oh my god, that's really cool.
Dan Shipper (00:57:37)
Yeah, there's so much culture that is inaccessible because the only person that gets to translate it is someone who has studied that for like 50 years. That’s important, but it'd be nice if there were 50 other translations that are more accessible for a particular kind of person.
Alice Albrecht (00:57:54)
And then doing the work of creating a story, something we've been thinking about a lot recently is local news, very local things that happen, nobody does the work—you don't have a ton of journalists out there doing this, it's kind of suffering—but you still need this information. You need it in a way that’s a story. And so I think this piece, it's not the meeting piece, but kind of. If it's the stuff that's happening to you when you're little wherever, whatever sphere you're encompassing here. And then I am very excited about underserved spaces, I guess. This is the thing where I feel like we can make an actual impact. This is the thing where we get people access to information that didn't have it before. And that's huge.
Dan Shipper (00:58:40)
It goes back to what you said earlier about raising the ceiling and lowering the bar. And lowering the bar for storytelling, I think is really important.
Alice Albrecht (00:58:48)
And story consumption. So I'm excited. I think 2025 for me is hopefully going to be this year where we see the application of these things, and your products coming out, I'm excited. You're incubating these things now. That's super exciting.
Dan Shipper (00:59:03)
I love that. I hope that's true. I'm excited for this year too. And I'm really glad that we got to chat. Thank you so much for coming on.
Alice Albrecht (00:59:10)
Yeah, it was good to see you.
Thanks to Scott Nover for editorial support.
Dan Shipper is the cofounder and CEO of Every, where he writes the Chain of Thought column and hosts the podcast AI & I. You can follow him on X at @danshipper and on LinkedIn, and Every on X at @every and on LinkedIn.
We also build AI tools for readers like you. Automate repeat writing with Spiral. Organize files automatically with Sparkle. Write something great with Lex.
Get paid for sharing Every with your friends. Join our referral program.
Ideas and Apps to
Thrive in the AI Age
The essential toolkit for those shaping the future
"This might be the best value you
can get from an AI subscription."
- Jay S.
Join 100,000+ leaders, builders, and innovators
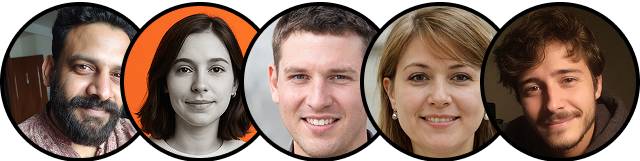
Email address
Already have an account? Sign in
What is included in a subscription?
Daily insights from AI pioneers + early access to powerful AI tools
Comments
Don't have an account? Sign up!