Where Are All the Autonomous Vehicles?
Three reasons why you shouldn’t sell your car just yet
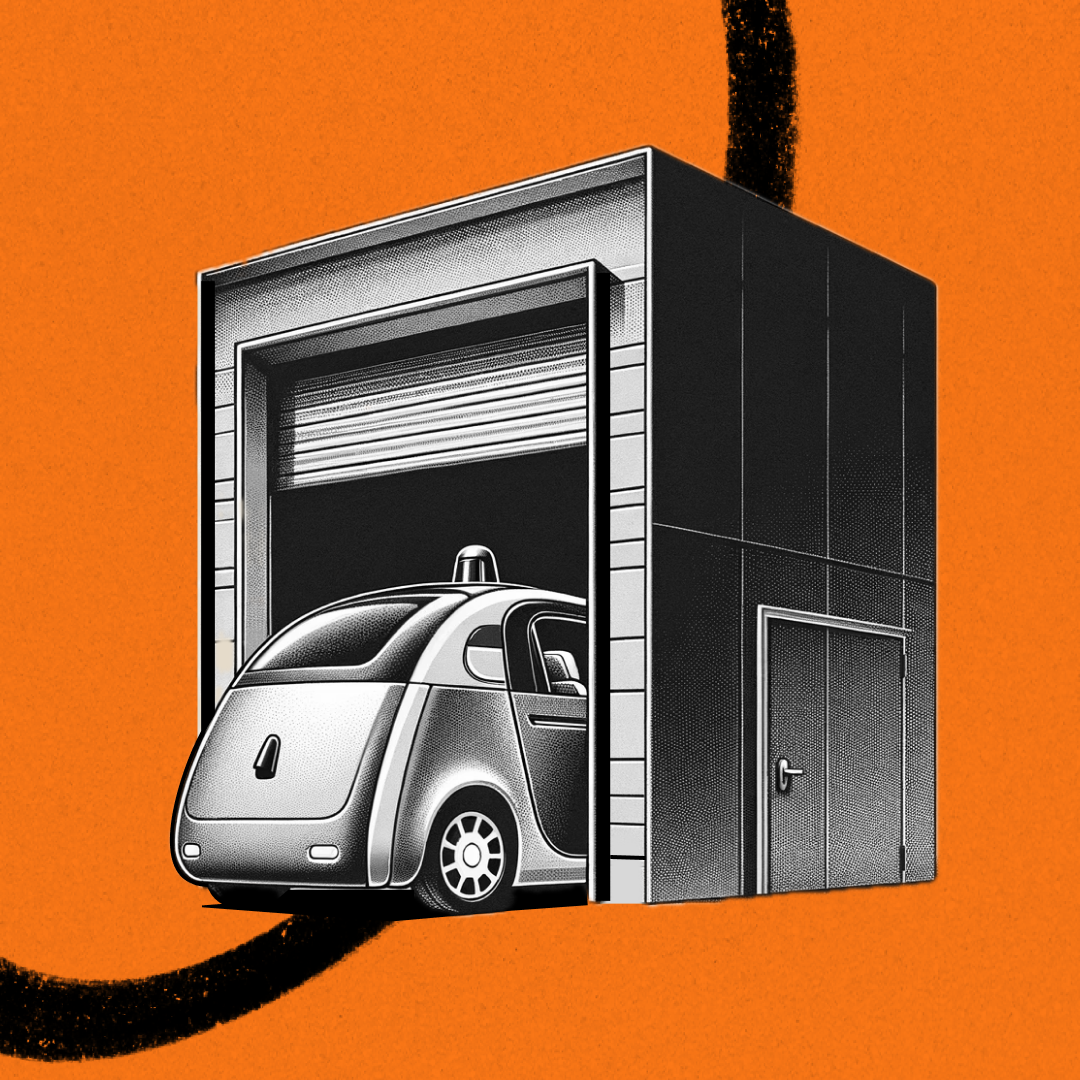
I love publishing views that strongly disagree with my own. The Every team thinks that autonomous cars are much closer to working than most people realize, but there are very smart and informed people who feel differently. As such, I’m proud to publish this original piece by Dimi Kellari, in which he argues the bear case to my personally held bull. It made me reexamine my views, and I encourage you to read it with an open mind.—Evan Armstrong
It was easy to spot Waymo’s employees. Their hoodies and T-shirts were adorned with an unmistakable blue-and-green “W.” I was working as a strategist at X, Alphabet’s self-styled moonshot factory, which shared an office with Waymo. We built potentially world-changing products, such as novel sensors, wildfire prediction models, and learning robots. While the work was fulfilling, some of our products never saw the light of day, let alone hit the market.
In the cafeteria, I struck up conversation with Waymo employees, peppering them with questions: When will driverless cars be everywhere? Are they actually safe? How safe do they need to be? How will they ever make money? Waymo’s cars seemed futuristic, just like the products we worked on at X, but they were already deployed for testing in the real world.
But I needed to know more. After a year at X, I found myself obsessed with the driverless future, mulling over philosophical questions about human risk and practical questions about the kind of infrastructure needed to make this a reality. Each year, more than 40,000 people die on the roads in the U.S., and many more people suffer debilitating injuries. I was drawn to the possibility of bringing something straight from science fiction into the real world—and making our roads safer for everyone.
By the end of 2019, I departed X to incubate a startup called Cavnue, which builds infrastructure, like dedicated lanes and road sensor technologies, for automated vehicles. (Until recently, I was the company’s head of systems and technology, and am still an advisor.) In the process, I spoke to nearly every autonomous vehicle (AV) startup and large automaker about their obstacles to success.
In the course of these conversations, I noticed something strange. Many companies had roadmaps that showed them, as they put it, achieving scale—running cost-effective operations in multiple locales—within the next few years. And many were struggling to operate commercially in even a single metropolitan area. It didn’t quite add up.
Five years later, I don’t believe that AV makers were disingenuous with their projections. Getting autonomous vehicles to scale isn’t as straightforward as one might think. Many misunderstood the bar of what it takes to be—and be seen as—“safe enough,” as well as the financial difficulties that come with rapidly growing these complex systems.
In the past two years, Ford has shut down the self-driving car venture Argo.ai. Meanwhile, the autonomous trucking company TuSimple exited the U.S. market, its peer Embark Trucks dropped from a $5 billion valuation and got acquired for $70 million, and General Motors’s autonomous subsidiary Cruise temporarily pulled all of its vehicles off the street. That’s not to mention the fires: Teslas have burst into flames, and a group of people in San Francisco set a Waymo vehicle on fire.
When the original investments were made in companies like Cruise, Argo, Zoox, Waymo, and others nearly a decade ago, the AV industry believed it would have been further ahead commercially by now. But the markets for these products have not yet materialized, in part because the products are not ready. For all the hoopla, autonomous cars are far from operating with true autonomy—humans are still very much involved.
What is holding us back from our driverless future? What problems still need to be solved? And when will we—as humans—feel like these cars are safe enough to drive us around?
Three reasons why driverless cars are so delayed
Self-driving technologies were supposed to usher in an era where anyone, anywhere, had access to reliable, affordable, safe, and low-emission transportation. An era with the experience of concierge cars, convenience of ride-hailing, affordability of public transit, and far fewer fatalities on the road. That may sound like a utopian vision, but that’s what we were promised by bright-eyed evangelists in Silicon Valley and Detroit.
Companies are building out autonomous vehicles in one of two ways. Either they’re building driver-out from the get-go—they’ll launch their vehicles with high levels of autonomy—or they’re building incrementally toward self-driving. Startups such as Aurora, Cruise, Kodiak, and Waymo are in the former camp while incumbent automakers and Tesla are in the latter.
While we’re a long way from autonomous vehicle ubiquity, you can experience self-driving in the fleets of autonomous taxis, or robotaxis, deployed by companies like Waymo in select cities. If you’re lucky enough to get off the waitlist to use its app to hail one, you’ll find yourself paying a similar price to an Uber or Lyft ride. When you are in the vehicle it can feel like magic, but it typically takes far longer to get to your destination than alternatives. There are three reasons why AVs are not yet widespread:
1. The bar for safety is higher than we thought.
2. Scaling driverless cars is harder than we thought.
3. The economics of driverless cars are shaky.
Understanding these three obstacles is the key to understanding why we’re still a ways away from autonomous vehicles being a part of everyday life.
The bar for safety is higher than we thought
Fully driverless vehicles need to pass safety cases if they are to drive in the real world. In the AV world, a safety case is self-regulated by the AV company—it’s how a company gains its own conviction about safety so as to not face liability issues with an unsafe vehicle.
Traditionally, safety evaluations have focused on identifying and fixing design faults associated with vehicle components and overall structure—airbags, brakes, and seat belts—while human drivers have been responsible for the safety of operations. This division of labor has kept the scope of safety manageable.
However, as driving tasks start becoming automated, the number of things that can go wrong is no longer bound by these design faults. There are nearly infinite scenarios to test for, many of which are uncommon but important for safety, otherwise known as edge cases. The countless nature of these events is often referred to as "the long tail" problem of autonomy.
One way to reduce the number of possible scenarios is to introduce constraints on the operational design domain (ODD)—basically, the context in which the vehicle can safely operate—and only allow it to function under certain conditions, like on certain roads, at certain times, under certain weather conditions. Within this narrow space, you can test the probability, severity, and controllability of events.
Unfortunately, the complexity of getting a vehicle to drive itself in this narrower ODD remains high. Let’s illustrate this with some simple math: Assume there is a particular event that can result in an injury or fatality, say, someone in a wheelchair chased a dog across a road. You cannot estimate how often this event happens without data: It could be once every 10,000 miles, giving it a 0.0001 percent chance of occurring on any given mile. In order to gather whether that is a fair estimate, you’ll have to travel at least 10,000 miles. For the sake of this example, let’s say you do encounter the case within 10,000 miles. If a vehicle drives eight hours a day at an average of 30 miles an hour, it would take about 42 days to encounter this edge case. Once you encounter it, you build and implement an algorithm that can safely navigate this situation. You test it in simulation and on the test track. The next step is to try it out in the real world. Statistically, it might take another 42 days to encounter the situation. It could also take less time or much, much more.
If you find that your algorithm does not work, you’ll have to try something else. And you’ll have to do this for all conceivable edge cases, typically paying one or two drivers per vehicle to gather data and carry out tests. You’ll likely also have a large fleet to parallelize the process. While you don’t always need humans and can leverage computer simulations for some of the testing, the time and costs can quickly add up—and so can the questions.
What if there are important scenarios that you do not happen to encounter during the period you are gathering data? You should probably gather more to be sure. For example—rare events that could have caused serious negative outcomes, like vehicle interactions with cyclists or pedestrians, otherwise known as vulnerable road users? You’ll need to find a solution for each of these. Do you need to gather data on every single road, or are some roads similar enough that it doesn't matter? How much data is enough data?
The situation is not quite as linear in reality. If you’re a company operating vehicles in the real world, you’re constantly gathering data about events. After you’ve driven millions of miles, you start to pick up on those one-in-a-million events. After tens of millions of miles, you grow more confident about the probabilities of these events. The more miles your cars have driven, the more confident you can be about event probabilities, which is why autonomous vehicle companies flaunt the number of miles they’ve driven.
These statistics might show that a vehicle is safer than a human driver within the ODD. If that’s the case, would that make it acceptable to deploy these vehicles at scale?
Safety is measured by the absence of unreasonable risk (AUR). Unreasonable risk encompasses a threshold that is determined to be acceptable by “societal moral concepts.” Each autonomous vehicle company is responsible for self-certification—they decide what this threshold should be. But it is difficult to determine this threshold for an autonomous vehicle. Does it simply need to be better than the average human driver? Better than the best human driver? Or something else? While research has shown that vehicles perform safer than human drivers within a specific ODD, it is still not clear that they pass the AUR threshold. The public’s reaction to various autonomous driving events has made it seem that the threshold might be zero deaths, zero injuries, and even zero traffic incidents—which is next to impossible. Where do we go from here?
Source: San Francisco Fire Department.Scaling driverless cars is harder than we thought
In 2018, former Waymo CEO John Krafcik said: “[Self-driving] is really, really hard. You don’t know what you don’t know until you’re really in there and trying to do things.” Scaling requires more than merely producing more vehicles and expanding geographically. You need to consistently develop the technology and supporting infrastructure.
On the development side, let’s revisit the process described earlier to pass a safety case in a given ODD. If you have trained and tested in San Francisco, do you need to carry out the same process in San Jose? What about Detroit or Miami? How can you be sure that the vehicle behaves the same across geographies? It might take decades to scale across the country.
And while some things do transfer—like what to do at stop signs or when you see brake lights—there are still many things that are more difficult to be replicated between cities: vehicle and pedestrian behaviors, road configurations, environmental conditions.
On the infrastructure side, there are many requirements to launch a fleet of functional robotaxis in a given geography:
- High-definition (HD) mapping: HD maps are used by many autonomous vehicles to understand where they are in the world, a function known as localization. Since the world changes daily (for example, road construction), every fleet of vehicles needs to update HD maps regularly.
- Remote support: All vehicles are remotely supported by operations centers with “tele-assist” teams. These teams are often used to handle the edge cases that the vehicle cannot navigate itself. Teams of people sit behind monitors and help guide these vehicles when they encounter any issue. While these teams have existed for a while, they caused a stir when their presence was thrust into the public eye.
- In-field support: Vehicle recovery operations teams attend to and rescue vehicles that encounter issues requiring human intervention, such as loss of connectivity, equipment failure, inability to navigate an edge case, or even a door that is left ajar. Without drivers, you either need to staff a dedicated team or train the local AAA-equivalent to deal with these incidents.
- Charging infrastructure: Most autonomous vehicle companies are working with electric vehicles (EVs). EV charging infrastructure is needed to support a fleet of hundreds of vehicles, which will require considerable work. Also, one of the biggest issues is interconnection with an electric grid that often requires upgrades to allow for the increased electric load of EVs. For this reason, EV ride-sharing company Revel has encountered many obstacles in its growth in New York City.
- Maintenance facilities: An autonomous vehicle has a variety of components that have not been previously deployed, such as Lidar sensors, computer graphics chips that are used to run algorithms, and control actuators that are used to physically move things like the steering wheel, brakes, and gas. When things go wrong, specially trained engineers must carry out repairs. And, as Kodiak’s director of external affairs, Dan Goff, explained, “[M]ost of those maintenance processes are proprietary, just because the technology is a little bit different from developer to developer.”
- Spare parts: As with maintenance staff, a supply chain of spare parts is required to be available across the country. This supply chain exists for regular vehicles, but it’ll take time to build out for autonomous vehicles.
- Cleaning facilities: Passengers can be messy. Vehicles require daily cleaning, and sensors that get dirty require immediate cleaning. To address the former requires special planning, as these vehicles cannot autonomously drive through and pay for a regular car wash today. To address the latter, many companies have built self-cleaning sensors, but they aren't always 100 percent effective.
- Insurance: As with all vehicles, cars require insurance in order to legally drive on public roads. Waymo and the insurance company Swiss Re have partnered on a study that shows that in a limited ODD, the Waymo AV is indeed safer than human drivers. Even so, insurance premiums are typically based on vast amounts of actuarial data. It is still uncertain how the insurance market will solve for AVs, although self-insurance is an option in some locations.
Some of these requirements—such as HD mapping, support teams, and EV charging infrastructure—pose serious challenges to rapid scale. The skills required to support the ecosystem are rare, and training this workforce will take time.
In the early days of autonomous vehicle development, the AV companies believed that scale would come easily once they cracked the code of self-driving in a single location. This assumption has since been proven wrong, as there are non-trivial technology and infrastructure requirements involved. Scaling a fleet of vehicles involves much more than just, well, vehicles. It takes significant capital, human resources, and time.
The economics of driverless cars are shaky
When the AV companies originally set forth their assumptions about robotaxi businesses, they jumped straight to the end state: largely “autonomous” vehicles that wouldn’t need the added costs of human drivers. It’s understandable: On the revenue side of the equation, the numbers look amazing. Using models that mimic ride-sharing companies like Uber and Lyft, we can assume an average of $50 of revenue per utilized hour in the U.S. (derived from Uber’s breakdown of driver pay in large cities). We can also estimate an average of 16 utilized hours per day (accounting for time to find and pick up passengers, and clean and charge cars), across 350 yearly working days (with two weeks for maintenance and repairs).
In this very rosy picture, the revenue is about $800 per vehicle per day and approximately $280,000 per year. These revenue numbers are much better than using a financial model with human drivers today. However, on the cost side of the equation, the numbers have proven to be closer to regular fleets than assumed. The cost for a fleet of robotaxis is not as simple as subtracting labor from that of a fleet of regular taxis, as additional technology and infrastructure adds up to a non-insignificant sum:
- Software platform: Ride-sharing and robotaxi businesses both require software platforms to interface with and engage customers. These platforms likely have similar cost structures.
- Capital: Robotaxis are expected to cost between $150,000 to $200,000 due to sensing and compute needs; a typical vehicle, such as a Toyota Prius, costs about $30,000.
- Maintenance: Robotaxi equipment has high maintenance costs stemming from its equipment, including sensors and high-performance chips.
- Labor support: This is a tough one. The support labor costs for robotaxis today are higher than ride-sharing: The ride-sharing vehicle today has a driver, who carries the burden of the support function, while the robotaxi does not. However, the human-to-vehicle ratio for remote and in-field operations for Cruise was 1.5 humans to one robotaxi (1.5:1). Someone who is supporting a robotaxi requires more training and might command a higher wage than a ride-sharing driver. This ratio will improve as we gather more data, but the question is by how much—and when.
- HD mapping: Ride-sharing today does not require HD mapping. Using back-of-the-envelope math, based on daily updates, mapping rates, sizes of areas served, and number of vehicles in a fleet, I’d put the cost for this somewhere between $5 to $20 per vehicle per day—$1,800 to $7,300 per year per vehicle.
- Technology upgrades: Over time, robotaxis will require updates and upgrades to their technology. Software and hardware upgrades will allow them to drive more comfortably, navigate more areas, and travel at higher speeds than AVs today. Will these upgrades be free of charge for robotaxi operators? Somehow, I don’t think so.
- Other: A fleet of robotaxis will require a depot from which to operate, where they can charge and be cleaned. In ride-sharing human drivers do much of this work, and it has not been automated yet for robotaxis.
The assumptions that have led to billions of dollars poured into this space rely on a future state in which these vehicles have passed the safety threshold and have scaled efficiently. We are not in that world yet, and we won’t be for a while. There are many stages along the way, which all involve adding more humans as guardrails—such as tele-assist or remote operations teams—to ensure smooth and safe operations. The original end game may not even be possible anymore. Will a human always need to bail out these vehicles when something goes wrong?
The funding problem for autonomous vehicles
Compare this scenario to the history of Uber, which was founded in 2009 and did not turn a profit for 14 years. The push into autonomous trucking was an attempt to establish a more compelling near-term business model. Trucks have a higher price-per-ride, as well as a more immediate need—there is a shortage of truck drivers because the job is so grueling. Unfortunately, even this path has faced challenges, such as the vast number of miles required to drive in order to train the system, the complexity of first- and last-mile operations, and the organized labor pools that are vehemently against this type of automation. None of this bodes well for profitability.
This situation is not uncommon. Startups use venture capital dollars to develop products and scale, with plans to generate profits down the line. According to a McKinsey survey, the path to get to scale will take another $5 billion for robotaxis and another $4 billion for autonomous trucks. I believe that these numbers are too low—Cruise spent $1.9 billion last year alone and is yet to achieve significant scale. In fact, Cruise is back in the data collection and training phase, albeit in a different ODD, with its coming relaunch in Phoenix after it pulled all its vehicles from San Francisco.
Investors have yet to demonstrate a sustained appetite to pour cash into thesecompanies, particularly in this macroeconomic environment. If recent news is anything to go by, it would seem that they are shying away. Take, for instance, autonomous vehicle company Motional, which in March announced a bridge loan that will tide it over while it tries to find longer-term sources of funding. Its major backer Aptiv announced that it would no longer fund the company, citing issues with unit economics.
But what about Tesla?
Many Tesla fans claim that the company has managed to scale self-driving everywhere. As of today, they would be wrong. Tesla states as much on its Autopilot webpage: “The currently enabled autopilot and full self-driving features require active driver supervision and do not make the vehicle autonomous.”
Such is also the case with Ford, GM, Audi, BMW, Volvo, and others, all of whom have developed similar driving automation technologies. These systems are known as "hands-off" systems. They require the driver to constantly monitor the road and intervene quickly in case something goes wrong—known as “human-in-the-loop,” in which a human is required to be part of the control loop of the system.
The Society of Automotive Engineers has developed the most commonly used framework for driving automation, known as the levels of automated driving. It ranges from Level 0, which is a traditional car with no automation, to Level 5, which has full automation and no human control.
Source: Philip Koopman.Currently, there is only one driving automation system on the market that is certified at Level 3: the Mercedes Drive Pilot. Level 3 features can carry out all of the driving tasks in their ODD but still rely on humans to intervene if something goes wrong. With Drive Pilot, you can drive on major freeways in parts of California and Nevada. Great! Until you realize that it is only certified for speeds under 40 miles per hour in daytime and in clear weather, on roads with clear markings, and with no construction present. That’s a lot of constraints!
Source: Mercedes.So, no. We don’t yet have true self-driving everywhere.
What’s next for autonomous vehicles?
We will not have widespread self-driving vehicles for a while; rather, there will be incremental improvements that will largely be unnoticeable. The first generation of self-driving companies will continue to develop their technologies and scale slowly, gradually expanding to new ODDs—assuming no major safety incidents lead to public uproar. They will try to make their business models work, but will still require significant external funding to support scaling. Turning a profit is many, many years away—at least a decade, based on the progress so far. And while self-driving trucking companies will likely find more commercial success, it will be in limited ODDs. Short but profitable autonomous trucking routes will become popular over the next five years as infrastructure is built out. For example, Aurora is planning a full commercial launch in 2024 within a limited ODD.
Meanwhile, automakers will continue to introduce new Level 2 features like better hands-free lane-keeping assistance, but they will be limited in their scope and value because of safety issues. GM's recent cancellation of its prominent Ultra Cruise program illustrates the difficulties in improving the current automated driving features. I suspect the company will follow Mercedes in introducing very limited Level 3 features, where the driver does not need to be alert and monitoring the road at all times. This will likely take three to five years for most of them to develop.
Other paths are possible, too. A new generation of autonomous vehicle companies, like Waabi and Wayve, is applying end-to-end algorithms that allow vehicles to operate without explicit human programming and complex HD mapping. Most of the older generation of self-driving companies require engineers to build discrete algorithms: perception ones that recognize things in the world, localization ones that pinpoint where the vehicle itself is situated in the world, motion-planning ones that calculate the right vehicle trajectory, and control ones to apply the right steering wheel angle or brake pressure. End-to-end algorithms do this in one fell swoop—no need to program these separate functions. The new generation of self-driving companies operate with the theory that advanced computer simulation can take on more of the burden of testing AVs, and in so doing, they will require far smaller operations teams. Tesla has recently adopted a similar end-to-end neural network approach and is slowly releasing it in new Full Self Driving system updates. However, it remains a Level 2 system—partial automation—for now, and is subject to all the obstacles mentioned earlier.
All of these companies show promise, but they have their challenges and a long way to go. While they may solve scalability problems, the safety bar remains extremely high. It is yet to be proven that we can meet this bar without the infrastructure and support that first-generation AV companies require.
The vision for autonomous vehicles is a noble one. They can potentially save lives and prevent injuries that we’ve come to accept as a natural and unavoidable part of human driving. Still, I worry that we are setting these vehicles up for failure if we don’t understand how they actually work. Without the right knowledge and long-term thinking, there could very well be an incident that sets us back—ushering in an autonomous vehicle winter. And the potential benefits of this world-changing technology may unfortunately slip out of reach.
Dimi Kellari is an engineer, tech entrepreneur, and angel investor who writes about deep tech and the evolution of science and technology. Most recently, he led engineering and technology strategy teams at Cavnue; previously he worked at X, the Moonshot Factory (formerly Google X), Airbus, CERN, and McKinsey & Company.
To read more essays like this, subscribe to Every, and follow us on X at @every and on LinkedIn.
Comments
Don't have an account? Sign up!
Excellent piece. I was expecting something a bit more optimistic - seems the best argument is that human drivers are much less safe, but that is not enough.
As a person who lives in a rust belt city with wildly variable road infrastructure, I can’t imagine how autonomous happens beyond very limited applications - no snow, quality roadways and logical traffic patterns. This is a long way away.