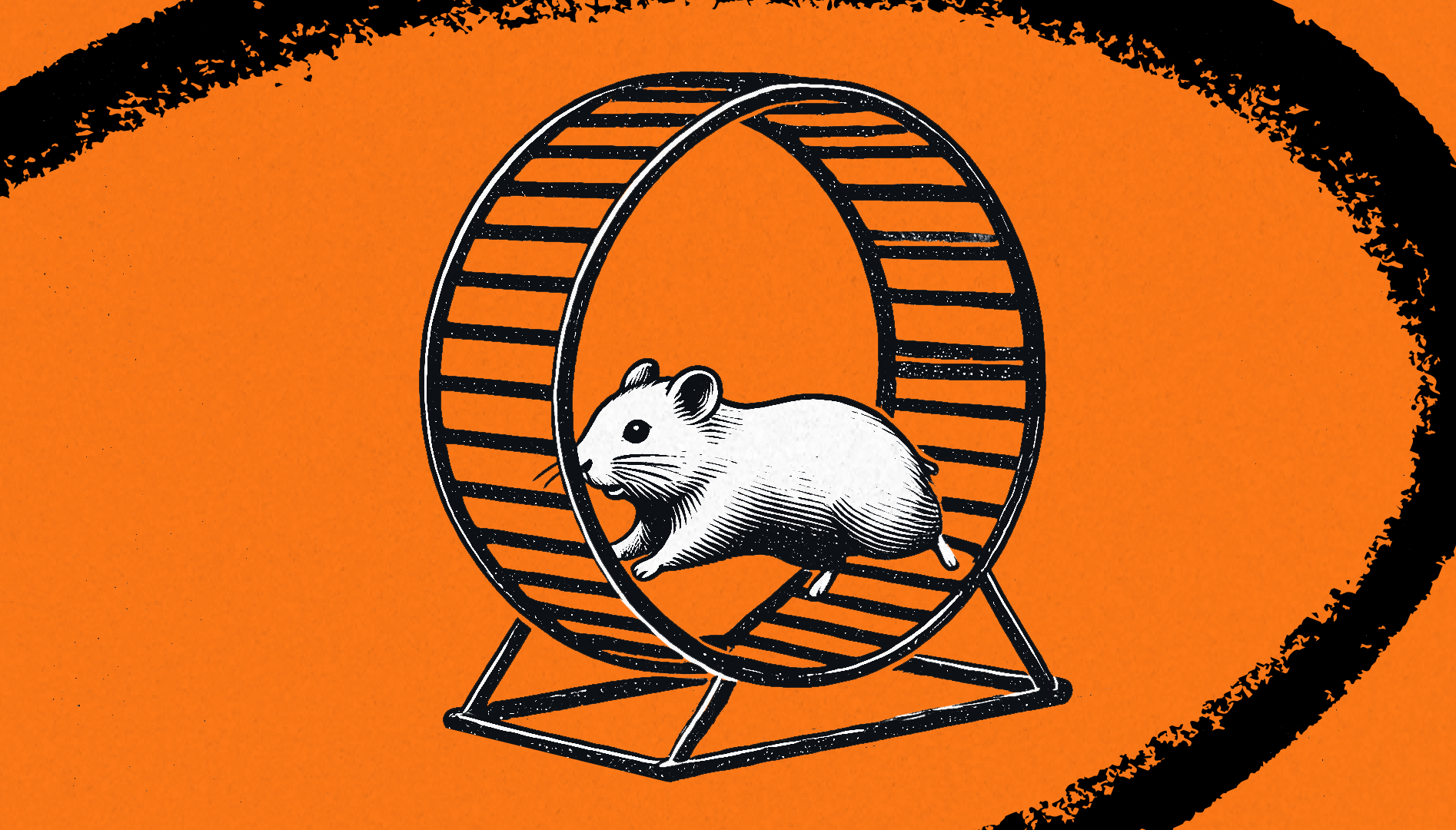
In Michael Taylor’s work as a prompt engineer, he’s found that many of the issues he encounters in managing AI tools—such as their inconsistency, tendency to make things up, and lack of creativity—are ones he used to struggle with when he ran a marketing agency. It’s all about giving these tools the right context to do the job, just like with humans. In the latest piece in his series Also True for Humans, about managing LLMs like you'd manage people, Michael explores self-consistency sampling, where you generate multiple responses from the LLM to arrive at the right answer. Every company with more than one person with the same job title does this to some extent—so start hiring more than one AI worker at a time. Plus: Learn how to best position yourself to compete against AI coworkers in the future.—Kate Lee
In my former life as the founder of a 50-person marketing agency that spent over $10 million on Facebook ads per year, I employed one trick to ensure that I always delivered for clients: I hired multiple people to do the same task. If I needed fresh creative designs and copy for Facebook advertising campaigns, I’d give three different designers the same brief. My thinking was that it was better to pay $200 each to three designers than risk losing a $20,000-a-month client. The chances were high that at least one of the three would deliver something the client liked.
Creative work is unpredictable by nature, and even great designers have off days or miss deadlines. I was comfortable with the trade-off: higher cost for greater reliability.
Now AI is doing some of that work, making it easier than ever to apply the principle of repetition. While your colleagues might be upset to learn you had given the same task to multiple people (“Don’t you trust me!?”), your AI coworkers don’t know, and don’t care. It’s trivial work to generate three or more responses with the same prompt and compare them to see which one you like best. This technique is called “self-consistency sampling,” and you can use it to make the trade-off between cost and reliability with AI.
Generative AI models are non-deterministic—they give you a different answer every time—but if you run the same prompt multiple times, you can take the most common answer as the final answer. I do this all the time in my professional work as a prompt engineer building AI applications, and as a user of AI tools. The simplest implementation of this concept is to hit the “Try again” button multiple times in ChatGPT to see how often I get the same answer. My co-author James Phoenix does the same thing by programming scripts that call ChatGPT’s API hundreds of times, counting the most common answer to major decisions. By urging the model to “Try again”—five or 500 times—you can get a better sense of the range of potential answers.
Source: Screenshot from ChatGPT.The realization that you can throw more AI at a problem and increase your chances of success is deceptively powerful, even if any one attempt is likely to be wrong. Let’s walk through how to take advantage of this technique—as well as the implications for your own work.
AI never tires of your asking for something
While my human employees would get frustrated if I asked them to do the same task multiple times, AI never pushes back. You can keep asking, and it costs you basically nothing to run the same prompt.
When I prompt GPT-4o to tell me the better name for a fake product I made up—a pair of shoes that fits any foot size—it usually returns the name “A) Fitshapes.” One in five times returns the name “B) Whimsoles” (one in twenty times it also breaks format completely and returns a preamble of why it chose that name).
Source: OpenAI Playground. Screenshot from the author.If the model gets the “wrong” answer of "A) Whimsoles" one in five times, you have a 20 percent chance of failure, or a one in five chance of being unlucky if you only run the prompt once. However, if you always run the same prompt five times and take the majority answer from those five attempts, you’ll only get it wrong about 5.7 percent of the time (i.e., about one in 20 times A) Whimsoles will be the majority instead of B) FitShapes). The downside for this extra reliability is that running the same prompt five times is that it takes five times as long.
In one of my most difficult projects, I was generating assessment reports for a team of psychologists based on a proprietary personality assessment quiz. To evaluate each report in real time, I trained an LLM judge (i.e., another prompt for GPT-4o) that ran after every report was generated, evaluating how many errors or issues the report had based on past assessments and feedback I put in the prompt. Now I could generate three versions of the same report and pick the one with the fewest errors, and it only cost me about $12 in OpenAI API fees instead of $4 while avoiding costly mistakes (that otherwise had to be manually corrected by the team).
Source: Screenshot from Jupyter Notebook.In Michael Taylor’s work as a prompt engineer, he’s found that many of the issues he encounters in managing AI tools—such as their inconsistency, tendency to make things up, and lack of creativity—are ones he used to struggle with when he ran a marketing agency. It’s all about giving these tools the right context to do the job, just like with humans. In the latest piece in his series Also True for Humans, about managing LLMs like you'd manage people, Michael explores self-consistency sampling, where you generate multiple responses from the LLM to arrive at the right answer. Every company with more than one person with the same job title does this to some extent—so start hiring more than one AI worker at a time. Plus: Learn how to best position yourself to compete against AI coworkers in the future.—Kate Lee
In my former life as the founder of a 50-person marketing agency that spent over $10 million on Facebook ads per year, I employed one trick to ensure that I always delivered for clients: I hired multiple people to do the same task. If I needed fresh creative designs and copy for Facebook advertising campaigns, I’d give three different designers the same brief. My thinking was that it was better to pay $200 each to three designers than risk losing a $20,000-a-month client. The chances were high that at least one of the three would deliver something the client liked.
Creative work is unpredictable by nature, and even great designers have off days or miss deadlines. I was comfortable with the trade-off: higher cost for greater reliability.
Now AI is doing some of that work, making it easier than ever to apply the principle of repetition. While your colleagues might be upset to learn you had given the same task to multiple people (“Don’t you trust me!?”), your AI coworkers don’t know, and don’t care. It’s trivial work to generate three or more responses with the same prompt and compare them to see which one you like best. This technique is called “self-consistency sampling,” and you can use it to make the trade-off between cost and reliability with AI.
Generative AI models are non-deterministic—they give you a different answer every time—but if you run the same prompt multiple times, you can take the most common answer as the final answer. I do this all the time in my professional work as a prompt engineer building AI applications, and as a user of AI tools. The simplest implementation of this concept is to hit the “Try again” button multiple times in ChatGPT to see how often I get the same answer. My co-author James Phoenix does the same thing by programming scripts that call ChatGPT’s API hundreds of times, counting the most common answer to major decisions. By urging the model to “Try again”—five or 500 times—you can get a better sense of the range of potential answers.
Source: Screenshot from ChatGPT.The realization that you can throw more AI at a problem and increase your chances of success is deceptively powerful, even if any one attempt is likely to be wrong. Let’s walk through how to take advantage of this technique—as well as the implications for your own work.
AI never tires of your asking for something
While my human employees would get frustrated if I asked them to do the same task multiple times, AI never pushes back. You can keep asking, and it costs you basically nothing to run the same prompt.
When I prompt GPT-4o to tell me the better name for a fake product I made up—a pair of shoes that fits any foot size—it usually returns the name “A) Fitshapes.” One in five times returns the name “B) Whimsoles” (one in twenty times it also breaks format completely and returns a preamble of why it chose that name).
Source: OpenAI Playground. Screenshot from the author.If the model gets the “wrong” answer of "A) Whimsoles" one in five times, you have a 20 percent chance of failure, or a one in five chance of being unlucky if you only run the prompt once. However, if you always run the same prompt five times and take the majority answer from those five attempts, you’ll only get it wrong about 5.7 percent of the time (i.e., about one in 20 times A) Whimsoles will be the majority instead of B) FitShapes). The downside for this extra reliability is that running the same prompt five times is that it takes five times as long.
In one of my most difficult projects, I was generating assessment reports for a team of psychologists based on a proprietary personality assessment quiz. To evaluate each report in real time, I trained an LLM judge (i.e., another prompt for GPT-4o) that ran after every report was generated, evaluating how many errors or issues the report had based on past assessments and feedback I put in the prompt. Now I could generate three versions of the same report and pick the one with the fewest errors, and it only cost me about $12 in OpenAI API fees instead of $4 while avoiding costly mistakes (that otherwise had to be manually corrected by the team).
Source: Screenshot from Jupyter Notebook.Mario Filho, a Kaggle grandmaster—a winner of data science competitions—does entity recognition in his work optimizing large-scale blog content to rank on Google. He identified the topics of a blog post using smaller, cheaper models like GPT-4o-mini and Google’s Gemini Flash 1.5 to process thousands of posts. The topic tags he was getting back weren’t consistent, so his solution was to run the prompt for each blog post three or more times, and merge the tags he got back into a single unique list that was consistent every time he ran it:
- Run 1: Artificial Intelligence, Healthcare, Medical Diagnosis, Patient Care, Machine Learning
- Run 2: AI in Healthcare, Healthcare Technology, Medical Diagnosis, Patient Care
- Run 3: Artificial Intelligence, Healthcare Technology, Patient Diagnosis, Machine Learning
- Merged unique list: Artificial Intelligence, Healthcare, Medical Diagnosis, Patient Care, Machine Learning, AI in Healthcare, Healthcare Technology, Healthcare Technology, Patient Diagnosis
Giving AI more time to think
OpenAI’s new o1-preview model is based on principles of chain of thought prompting, where you ask an LLM to break down its response into multiple steps before answering. Think of self-consistency sampling as a continuation of the theme of throwing more “thinking time” at a problem to improve the quality of the LLM’s response. In fact, self-consistency sampling was introduced to solve the variability that chain of thought returns, as the LLM can go off in a random direction if it gets one of the steps in logic wrong.
Source: Datadrifters, Medium.While it might get prohibitively expensive to improve models by investing more computing power in pre-training, the o1 model opens up a new scaling law: Spend more tokens on “thinking” through a problem, and you get predictably better results. Self-consistency sampling is part of that paradigm, spending more tokens horizontally (multiple responses) instead of vertically (longer responses). The trick is that while individual reasoning attempts may contain errors, the correct answer is likely to appear consistently across multiple attempts. After generating a diversity of paths, the technique aggregates the results, typically by selecting the most frequent answer.
Source: Arxiv.This approach leverages the language model's ability to approach problems from different angles, potentially overcoming biases or errors present in any single reasoning attempt. Self-consistency sampling is particularly effective for complex reasoning tasks such as mathematical problem-solving or multi-step logical deductions, where it can significantly improve accuracy without requiring any additional training of the underlying model. By embracing variability in the model's outputs, this technique offers a robust way to enhance the reliability and performance of language models in reasoning tasks.
Researchers have demonstrated that self-consistency significantly improves performance across a range of arithmetic and common-sense reasoning tasks. They have shown substantial gains on commonly accepted reasoning benchmarks from +3.9 percent on identifying the next image in a sequence to +17.9 percent on grade-school math word problems. Even better, self-consistency requires no additional training or fine-tuning and works across all language models, making it a simple yet effective method for enhancing reasoning capabilities in LLMs.
Avoid doing jobs that are easy to compare
While most people don’t hire three designers to unknowingly compete on a single task, companies do compare the output of their employees all the time. It might not be done in parallel as one might do using an LLM, but every worker’s results are in some way compared to their peers. Customer service calls are monitored for quality control and training (as you may hear on one of these calls yourself), whose real purpose is that companies can more easily evaluate which agents do a better job.
Some of the best career advice I ever got was, “Never do the main job the company does.” For instance, an accountant at an accounting firm will be more closely judged than an accountant at a marketing firm (and the reverse is true). If most of your colleagues do the same thing as you, the company will be good at evaluating your performance, whereas if you’re the only person doing that job, you are less easily replaceable and can potentially be paid more for less. Today this advice is even more important—your output isn’t only being compared to that of your coworkers, but also to what the latest AI models can do.
Given that LLMs are so much more amenable to evaluation than human workers, the companies I work with are investing a lot of time into perfecting ways to evaluate them. But once you get better at measuring how good AIs are at a task, you can’t help but compare them to humans. It’s no surprise that the first sector in which jobs are being lost to AI are in highly measurable roles like customer service agents, two-thirds of which Klarna now handles with AI. AI might not be better than you right now, but being able to ask it to do a task 1,000 different ways without pushback means it will get better more quickly. We should modify that career advice: “Never do a job an LLM can do.”
Source: Klarna.Solving math word problems with logical reasoning
To see self-consistency sampling in action, let’s dig into a demonstration I made for a client. First I created a bunch of math word problems that require complex reasoning and attempted to see if I could get GPT-4o to answer them. Here is one example, which you might see in a high school math quiz:
When you run this question through my prompt, which instructs GPT-4o to think step by step, the output is something like this:The reasoning chain looks legible to someone like me who doesn’t know much math, but the problem is that this answer—$930—is wrong. The correct answer is $825, by producing 50 widgets and 15 gadgets. (OpenAI’s o1-preview model gets it right the first time, but most smaller and cheaper LLMs are bad at math, and often get these sorts of tasks wrong.)Source: Screenshot from ChatGPT.However, by applying the self-consistency sampling approach, there is a chance of getting the right answer. Run the same prompt five times, and it gets the answer right twice. The rest of the time, it fails to give the right answer or follow my instructions on how to provide the answer at the end (so my script fails and provides a blank answer). Luckily for us, we got the right answer overall, because $825 was the most consistent answer—two out of five times—so we weren’t derailed by these frequent errors.
Source: Screenshot from Jupyter Notebook.Sometimes you need to pay more to ensure you get the right answer
People resent paying more to do the same task multiple times, so self-consistency sampling is underused. When I was at my agency, many of the other people on my team thought it was a waste of money or even unethical to ask more than one person to do the same job. As the founder, all I cared about was getting a consistent end result for the clients, so I overruled their protests, but I haven’t seen that many people using the same strategy. Similarly, AI engineers I talk to seem reluctant to build a system that runs the same prompt multiple times, despite the fact that it costs a couple bucks at most and saves time for a human who makes hundreds of dollars an hour.
Of course, self-consistency sampling isn’t a panacea, and there are times it doesn’t make sense. Some tasks (like discovering new scientific theorems) cannot be done by LLMs, so it doesn’t matter how many times you re-run the prompt. For many tasks, the evaluations themselves are complex, so you have no way to automatically select the best of five or 10 responses. Sometimes you need a human domain expert to evaluate each response, and it may take longer and be less desirable to review multiple LLM responses than it would for the expert to do it themselves the old-fashioned way.
The mindset you need to adopt is that you don’t have to limit yourself to one LLM response. There’s usually a way to throw more firepower at a problem and solve it by brute force—whether that’s using chain of thought for thinking time up front, self-consistency sampling for multiple answers in parallel, or a combination of both. As LLM inference costs get increasingly cheaper, throwing more thinking time at the problem will work better and better.
Michael Taylor is a freelance prompt engineer, the creator of the top prompt engineering course on Udemy, and the coauthor of Prompt Engineering for Generative AI.
To read more essays like this, subscribe to Every, and follow us on X at @every and on LinkedIn.
Ideas and Apps to
Thrive in the AI Age
The essential toolkit for those shaping the future
"This might be the best value you
can get from an AI subscription."
- Jay S.
Join 100,000+ leaders, builders, and innovators
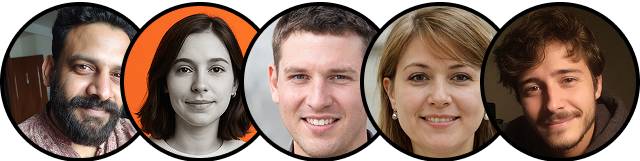
Email address
Already have an account? Sign in
What is included in a subscription?
Daily insights from AI pioneers + early access to powerful AI tools
Ideas and Apps to
Thrive in the AI Age
The essential toolkit for those shaping the future
"This might be the best value you
can get from an AI subscription."
- Jay S.
Join 100,000+ leaders, builders, and innovators
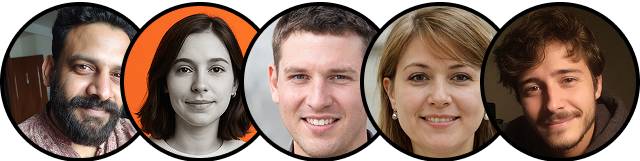
Email address
Already have an account? Sign in
What is included in a subscription?
Daily insights from AI pioneers + early access to powerful AI tools