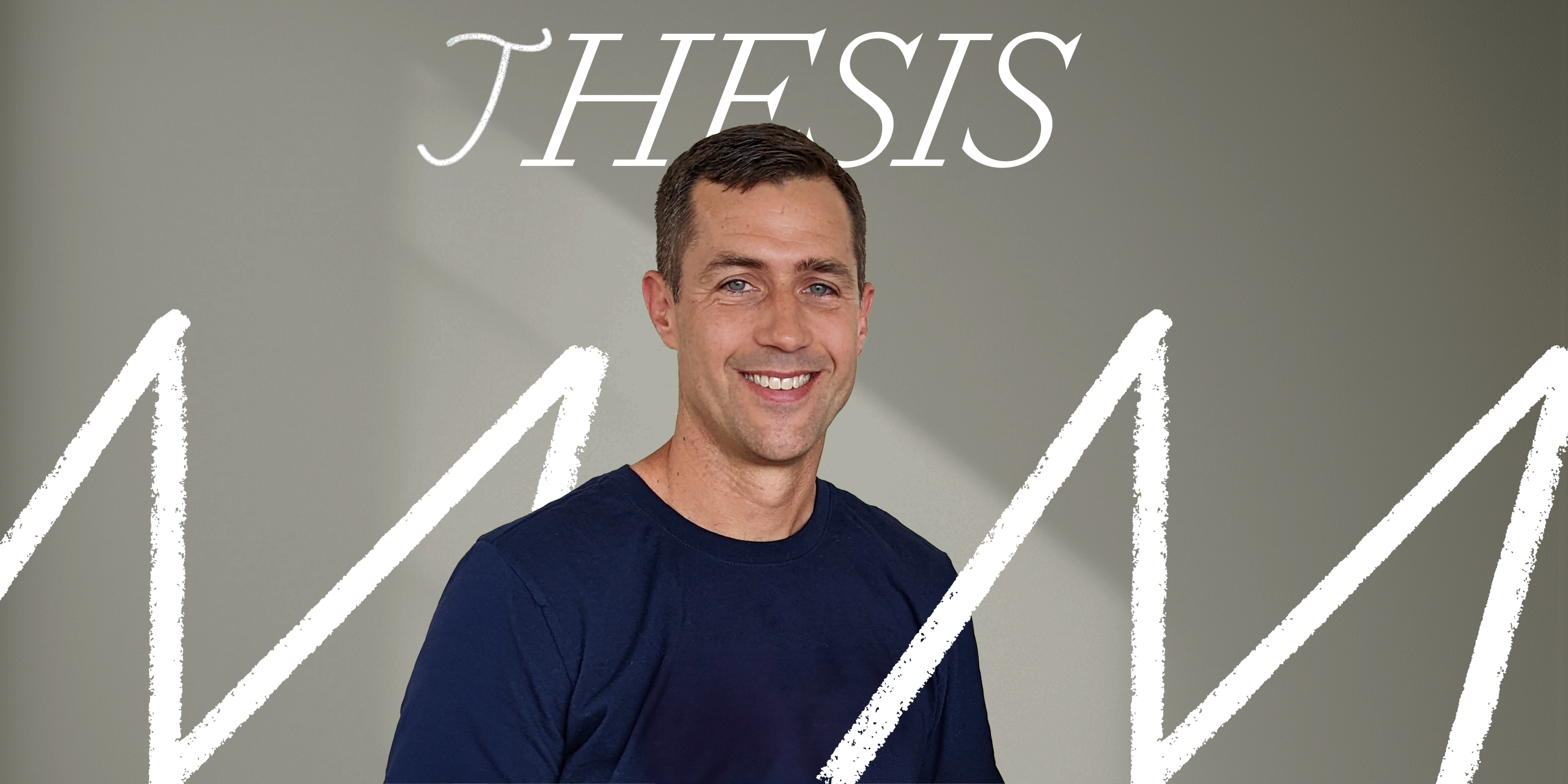
Silicon Valley prognosticators often make bold pronouncements about the point at which artificial intelligence (also known as artificial general intelligence or AGI) surpasses human cognitive ability. But while many tech leaders talk at length about their ambitions or fears regarding building AGI, that’s a distraction from something more important, argues venture capitalist Phin Barnes in this week’s Thesis: building good businesses.—Kate Lee
“I think the race to AGI is somewhat overblown,” an engineer at my firm recently wrote to me. “As we get closer to AGI, the definition itself will diffuse and fragment—not into five or 10 variations, but into thousands of ‘not really AGI’ solutions.”
This sentiment captured something I’d struggled to articulate in conversations with founders and fellow investors. Artificial general intelligence (AGI) is typically defined as a form of AI with the cognitive ability to learn, reason, adapt, and perform any intellectual task on par with humans. But the market for AGI—potentially the biggest shift in human-computer interaction in our lifetime—is surprisingly small. The current narrative around AGI has become too simplified, overly corporate in its winner-take-all framing. While AGI’s impact on humanity will surely be profound, the real economic opportunity lies in narrow, cost-efficient models that can handle specialized tasks.
The global AI market is already worth billions, soon to be trillions—so why would the tremendous promise of AGI create incredible social value but capture significantly less economic value? Consider the actual needs of industry: A manufacturing company doesn't need its AI to write poetry while controlling robot arms. A pharmaceutical firm doesn't need its drug discovery model to also handle customer service.
The trajectory for AGI, then, seems clear. As AI models get better, they hit a point of being “good enough,” and then pivot toward specialized applications. This specialization—not general intelligence—is where most of the economics of AI will be captured. Down the line, the best founders will likely not be comparing foundation model capabilities, but rather the “build versus buy” decision to create scaffolding around large models (open or closed) for specific needs. The ability to point a model at a specific use case will become the most important problem in the value chain of AI, so foundation model companies will compete to be the easiest to use and integrate.
As AGI hype collides with reality, understanding the constraints of today’s technology—power, scale, and data—will give builders a strategic edge. Let’s dive into the three biggest opportunity areas I see for builders in AI, as well as a strategic playbook for founders.
Sponsored by: Every
Tools for a new generation of builders
When you write a lot about AI like we do, it’s hard not to see opportunities. We build tools for our team to become faster and better. When they work well, we bring them to our readers, too. We have a hunch: If you like reading Every, you’ll like what we’ve made.
The gap between AGI hype and market realities
Why wouldn't everyone adopt AGI for everything once it's available? Simple: economics. Absent a more efficient breakthrough architecture, AGI will be inherently more power-hungry and expensive than specialized models with a narrower focus. And that expense goes beyond just electricity costs for inference, the process by which an AI model applies its trained knowledge to analyze new data and generate outputs. Will AGI run efficiently on your phone? What happens when it demands 100 times more RAM? What if every response comes with noticeable latency?
These aren't theoretical concerns—they're practical barriers pushing real companies toward narrow use case workflows, infrastructure, and system architecture. This said, builders who understand the constraints are finding ways to turn them into opportunities. Here are the three biggest opportunity areas I’m seeing in play.
The power wall
Training GPT-4 reportedly consumed around 500 megawatts during peak runs—roughly equivalent to powering 40,000 U.S. homes. If scaling predictions hold true, a hypothetical AGI model could require three to four gigawatts, which is more than a third of New York City's peak power demand. For context, Google's entire global operation, including all of its data centers, currently uses about 15.4 gigawatts. When a single AI training run starts demanding enough electricity to dim the lights in Manhattan, we've got a problem.
But perhaps it's more of an opportunity (and not just for nuclear power startups). For over 50 years, hardware and software have performed an intricate dance of efficiency: Hardware engineers push the boundaries of what's possible per watt, and software developers dream up new ways to use that power.
We've seen this pattern before. When gaming demands outpaced central processing units (CPUs), the industry pivoted and created graphics processing units (GPUs). Now, we're seeing the next wave in software demands, and the hardware market is responding. Google's tensor processing units (TPUs, in development since 2014), startups like Etch and Groq, and Apple's M-series chips are all reimagining chip architecture from different angles. Meanwhile, software techniques like LoRA are revolutionizing how we fine-tune models, dramatically reducing computational needs.
It’s the cycle of tech at its best. When we hit a wall, we build a door.
The physics of scale
The quest for more compute is forcing companies to distribute training across data centers. Physics becomes our enemy here. Light takes about three milliseconds to travel 1,000 kilometers through fiber-optic cables. For distributed training to work effectively, we need sub-millisecond latency between nodes, and that requires geographic proximity. To satisfy this need, our data centers can't be more than a few hundred kilometers apart, severely limiting where we can build them.
Distributed systems challenges aren’t unique to AI; they're the latest variation of problems that Google, Microsoft, and Amazon have worked around for decades. In 2012, Google's Spanner project (a globally distributed database) solved essentially the same problem: synchronizing vast amounts of data across global data centers with microsecond precision. It may be a different payload, but the same fundamental challenge: How do you orchestrate massive computational tasks across distributed systems with minimal latency? Opportunities are baked into answering that question.
The data demand
The third and perhaps most interesting constraint is data. As The Information recently reported, we're approaching a data wall that's both quantitative and qualitative. The entire internet’s text content is estimated at about 100 petabytes, of which GPT-4 is already trained on a significant fraction. Where do we go from here?
Synthetic data might seem like a solution, but it's a circular dependency: We're using AI to create training data for better AI. At some point, you're creating an echo chamber of artificial knowledge, and new models may resemble old ones.
The real opportunity lies in the vast ocean of untapped, real-world data:
- The low-hanging fruit. This is data that exists but needs muscle to extract. Imagine combining every broadcast, podcast, and live stream with satellite imagery and real-time weather data. During a hurricane, you're not just seeing news footage—you're correlating ground conditions with atmospheric patterns and historical data. That's not just more data; it's better data on account of how it’s joined.
- The hidden treasures. There are vast troves of offline and semi-private information. Every city has land ownership records, site conditions, and tax data. Most of it isn’t online. Some of it isn’t even digitized. Add to this semi-private transaction data, targeting insights, and private social platforms. It's messy, fragmented, and extremely valuable.
- The experiential holy grail. This is the data that captures what it means to be human: the ambient conversation in a busy coffee shop. The controlled chaos of a city at rush hour. The subtle dynamics of an office meeting. Whoever captures human interactions in ways that create genuine understanding will be able to build AI that understands humanity—and redefine our relationship with computers in the process.
The path to value with AGI
For founders, these constraints—energy, scalability, and data—are the makings of a clear strategic playbook:
- Build knowing that what’s expensive and impractical today will be commodity computing tomorrow. Powerful models will become cheap and abundant. Those models will change how we live and work with computers. Knowing how to apply them effectively will become rare and valuable. The next $10-plus billion AI company won't be built on having the best model but on having the freedom to use the right model for the right job, at the right time.
- Choose your problem’s scale carefully. Rather than chasing “magic” that works sometimes, build reliable solutions with “good enough” AI that focuses on a specific problem. You will create more value than a less dependable, more sophisticated general solution.
- Maintain model independence. As foundation model companies realize AGI is a shrinking piece of the pie, they will try to lock you into their ecosystem. Developer tools, infrastructure, and support systems are traps with sharp teeth. Build without getting locked in, and you’ll maintain a competitive advantage.
- Most importantly, start by building the expensive version. Today's state-of-the-art models give us an unprecedented preview of what’s possible. But they’re expensive—and in some cases, too slow—to build a business around. For now. So when you use o1 or GPT-4o, use them to prototype. Even if current costs make them impractical for production, you’ll get a clear sense of the capabilities that will become practical and profitable as models get cheaper in the coming years.
Don’t let those high API costs discourage you. They’re not barriers to entry—they’re your R&D. As these capabilities march down the cost curve through improved infrastructure, optimized architectures, and open-source alternatives, the experiences you've designed and tested will become increasingly viable. Sometimes, the best tool isn't the most powerful one—it's the one that cuts exactly as deep as you need it to. That's where the real market—and the real opportunity—lies.
Thanks to Alec Flett for editorial support.
Phin Barnes is a cofounder and managing partner at The General Partnership (TheGP), a venture capital firm designed to deliver “sweat equity” agreements in addition to term sheets. Previously, he was a managing partner at First Round Capital. He is on X at @phineasb and occasionally publishes writing in his newsletter.
To read more essays like this, subscribe to Every, and follow us on X at @every and on LinkedIn.
We also build AI tools for readers like you. Automate repeat writing with Spiral. Organize files automatically with Sparkle. Write something great with Lex.
Ideas and Apps to
Thrive in the AI Age
The essential toolkit for those shaping the future
"This might be the best value you
can get from an AI subscription."
- Jay S.
Join 100,000+ leaders, builders, and innovators
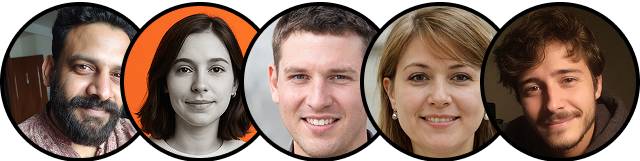
Email address
Already have an account? Sign in
What is included in a subscription?
Daily insights from AI pioneers + early access to powerful AI tools
Comments
Don't have an account? Sign up!
I love to know more about what you mean by 3. "The experiential holy grail" and if you see any companies building in this space?