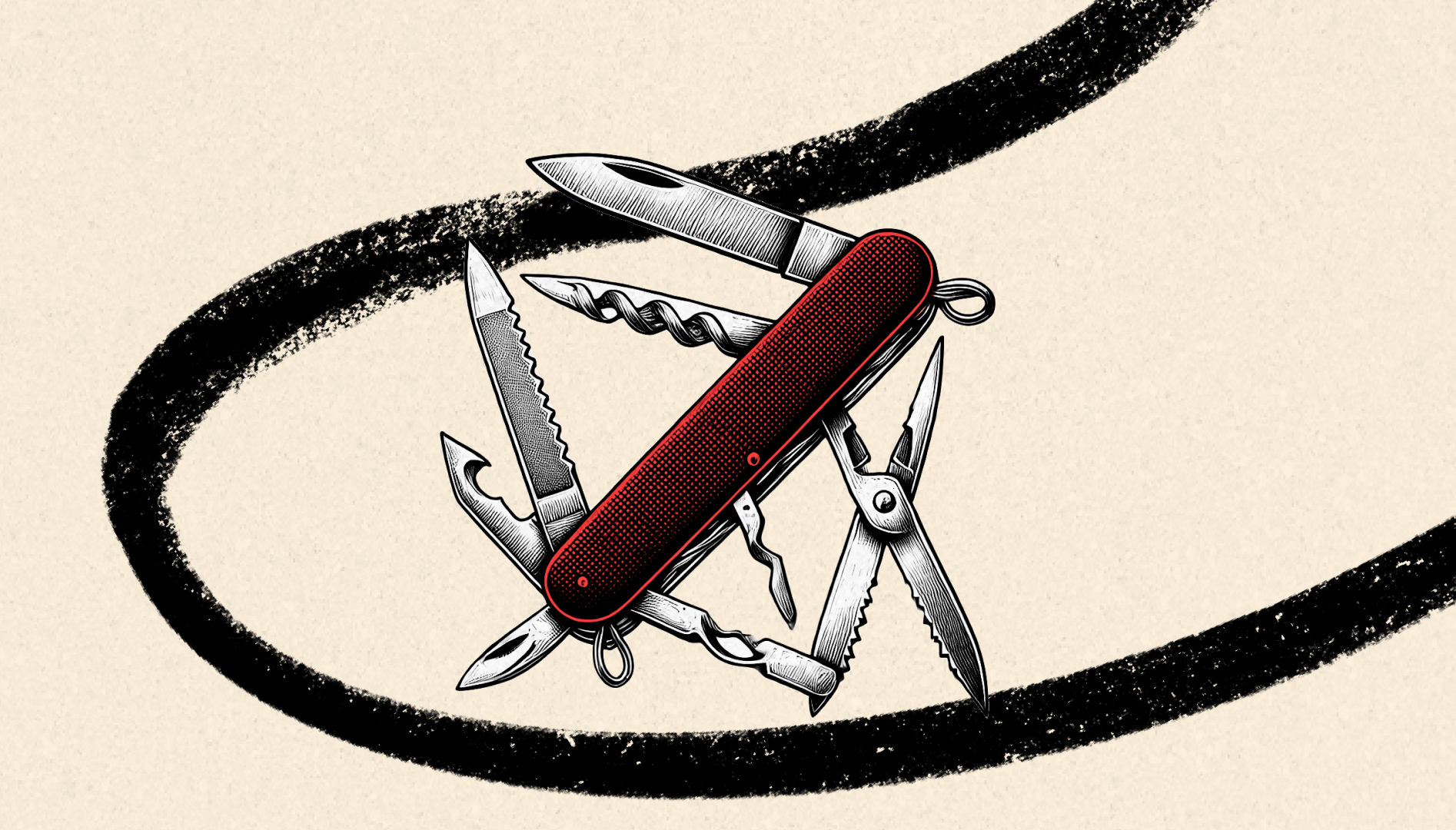
Was this newsletter forwarded to you? Sign up to get it in your inbox.
A common refrain I hear is that in the age of AI, you don’t want to be a “jack of all trades and a master of none.”
For example, my good friend (and former Every writer) Nat Eliason recently argued:
“Trying to be a generalist is the worst professional mistake you can make right now. Everyone in the world is getting access to basic competence in every white-collar skill. Your ‘skill stack’ will cost $30/month for anyone to use in 3-5 years.”
He makes a reasonable point. If we think of a generalist as someone with broad, basic competence in a wide variety of domains, then in the age of AI, being a generalist is a risky career move. A language model is going to beat your shallow expertise any day of the week.
But I think knowing a little bit about a lot is only a small part of what it means to be a generalist. And that if you look at who generalists are—and at the kind of mindset that drives a person who knows a lot about a little—you’ll come to a very different conclusion: In the age of AI, generalists own the future.
What generalists are
Generalists are usually curious people who like to hop around from domain to domain. They enjoy figuring things out, especially in areas that are uncertain or new. They’re good at solving problems that domain experts struggle with, because they’re able to bring bits of knowledge from diverse fields together.
As Nat notes, because of their propensity to hop domains, generalists tend to possess a wide set of shallow skills. But measuring them against their rudimentary coding abilities or their working knowledge of French baking technique misses their true advantage: the ability to adapt to new situations, and the desire to do so.
Where generalists thrive
In Range: How Generalists Triumph in a Specialized World, David Epstein argues that generalists are especially good in what he calls “wicked” environments: “In wicked domains, the rules of the game are often unclear or incomplete, there may or may not be repetitive patterns and they may not be obvious, and feedback is often delayed, inaccurate, or both.”
According to Epstein, this is where generalists thrive. They are able to use their diverse experiences to attack problems in unique ways and see solutions that no one else can see.
He contrasts wicked environments with what he calls “kind” environments, where feedback is immediate and there are clear, repetitive patterns that lead to success. These are the domains where the experts tend to shine. They can apply their specific expertise to solving problems over and over again, because they’ve seen those problems in some form before.
Kind environments, interestingly enough, are also where LLMs thrive. A few weeks ago, I likened large language models like GPT-4o and Claude Sonnet 3.5 to having “10,000 Ph.D.’s available at your fingertips.” They are quite proficient at most areas of specialist knowledge in the world. But they are still not very good at figuring out entirely novel problems.
This view of LLMs suggests the reverse of Nat’s thesis: trouble for experts and opportunities for generalists. LLMs are weak in wicked domains and strong in kind ones where experts thrive. If you’re an expert navigating a novel problem, an LLM won’t imagine a new solution for you. But they become a gift for generalists, who can use them to get up to speed in new domains much more quickly, and resurface and apply knowledge from other fields easily. Generalists can use their adaptability and imagination to work through any “wickedness” that a language model can’t handle on its own.
In an allocation economy, where you’re compensated not based on what you know, but on your ability to deploy intelligence, language models aren’t a threat to generalists—they are a potent weapon.
The past and future of generalists
Generalists have been out of style since at least the time of Adam Smith, who popularized the notion of specialization and division of labor as a driver of economic growth in the 18th century. In fact, we probably have to go back to ancient Greece to find an example of a society where generalists were the norm instead of the exception.
Ancient Athens was a direct democracy. Citizens, as a rule, participated in all aspects of civic life, from politics to warfare. Any citizen could be judge, jury member, senator, and soldier. In The Greeks, the classicist H.D.F. Kitto writes that Athenian society was driven by the ideal that in Athens, “a man owed it to himself, as well as to the [city], to be everything in turn.” This ethos, Kitto argues, implied “a respect for the wholeness or the oneness of life, and a consequent dislike of specialization.” In short, Athens was a society of well-rounded individuals—or generalists. (Of course, it must be noted that citizenship was limited to adult males. Athens was not a utopia.)
But as time passed, and life in Athens became more economically, socially, politically, and militarily advanced, this society of generalists began to fissure. Progress equals complexity, and complexity requires specialization. As Kitto explains it, “If one man in his time is to play all the parts, these parts must not be too difficult for the ordinary man to learn. And this is where the polis broke down.“
As AI becomes more capable of specialized tasks, we might see a return to the Greek ideal of the well-rounded citizen. This time, though, we can do it in the context of an advanced and complex economy because a citizen armed with AI will be far more capable of playing many roles than one without.
Being a generalist gives us something that language models don’t have: the capacity to learn quickly, and to see and solve novel problems in new domains.
In an allocation economy, the person who wins isn’t the expert who knows the exact answer to a question.
It’s the one who knows which questions to ask in the first place.
Dan Shipper is the cofounder and CEO of Every, where he writes the Chain of Thought column and hosts the podcast AI & I. You can follow him on X at @danshipper and on LinkedIn, and Every on X at @every and on LinkedIn.
Find Out What
Comes Next in Tech.
Start your free trial.
New ideas to help you build the future—in your inbox, every day. Trusted by over 75,000 readers.
SubscribeAlready have an account? Sign in
What's included?
-
Unlimited access to our daily essays by Dan Shipper, Evan Armstrong, and a roster of the best tech writers on the internet
-
Full access to an archive of hundreds of in-depth articles
-
-
Priority access and subscriber-only discounts to courses, events, and more
-
Ad-free experience
-
Access to our Discord community
Comments
Don't have an account? Sign up!
Totally agree. I also think that having a specialist area (one you've dived deep into in the past) helps you know what questions to ask about the new thing you want to learn about. To be able to do both might be where the super power is at.
@richoheg Hi, this is Kate Lee, Every's EIC. We'd like to include your feedback in an upcoming edition of our Sunday newsletter. Would you let me know your job function? Feel free to respond here or email me at [email protected]. Thank you!
"A jack of all trades is a master of none, but oftentimes is better than a master of one."
The second part is often forgotten or unknown.
The book "The Neo-Generalist" revises the _of none_ part: _None_ doesnt mean zero, it means not 100% depth. Imho a jack of all trades (@85+% in several disciplines) will always manage better in complex situations.
Its just a pity that most education systems still produce workforce for the 19th century factory.
Long term versatility will beat depth, because most fundamental problems man produces come from a lacking holistic approach and adaptability. I totally agree with your conclusion.
I really love this article - thank you! Of course I am very biased, being a generalist myself but have found this a good way to progress my career as well as aiding personal development and building skills outside of my immediate career. It has also given me confidence to tackle most situations; obviously not open-heart surgery, but instances in the corporate world.
This was one of the first articles that I read of yours Dan and it hit me so hard and just that realization of this is who I am and how this age of AI has really given me my power back as this generalist who can see what others don't see and knows how to connect the dots and can adapt, and now has the ability to leverage the deep dive and the expertise of AI and it makes the future so exciting for me. Great job on articulating this.
Although I agree with the article, the real situation for generalists is that it is hard to convince others that you have the ability to solve more cross-field problems with your diverse insights (unless you have already accomplished something extraordinary).
Since not everyone dreams of becoming an entrepreneur, proving past experience to secure employment is still important.
@doremi31618
Unless you are occupying a supervisory/managerial role in an establishment, I think a generalist only functions best as an entrepreneur.
And it's a good thing IMO. Once Artificial General Intelligence (AGI) comes, even managerial roles will be taken over. Entrepreneurs are owners and allocators of capital. They cannot be replaced.
**"It's the one who knows which questions to ask in the first place."**
I've found that the questions we ask often come from our domain experience and curiosity. As Kitto implied, there's "a respect for the wholeness or the oneness of life, and a consequent dislike of specialization." What's true for economies is also true for nature.
When environmental changes occur, generalists survive while highly specialized species struggle to adapt. And why should they adapt? They've developed features, diets, and hunting methods specifically designed to thrive in stable, unchanging environments—tools honed over a great length of time in non-hostile conditions. But when change happens—a change that levels the playing field—it becomes about who can utilize it to thrive and who cannot.
By tapping into our understanding of areas we're familiar with and drawing from diverse experiences, we're able to explore more effectively and solve problems outside our usual domains—things that were once not possible.
AI is a force multiplier. Applied correctly, it yields significant results. But it's also a threat. Because it creates a division between those who can use it and those who cannot.
Consider leveraging AI at the very beginning of your problem-solving process. Even if you don't know the answers to questions outside your fields of expertise, AI can multiply our efforts and help us ask the right questions. Turning you specialist domain expertise into general domain competence.
Love it