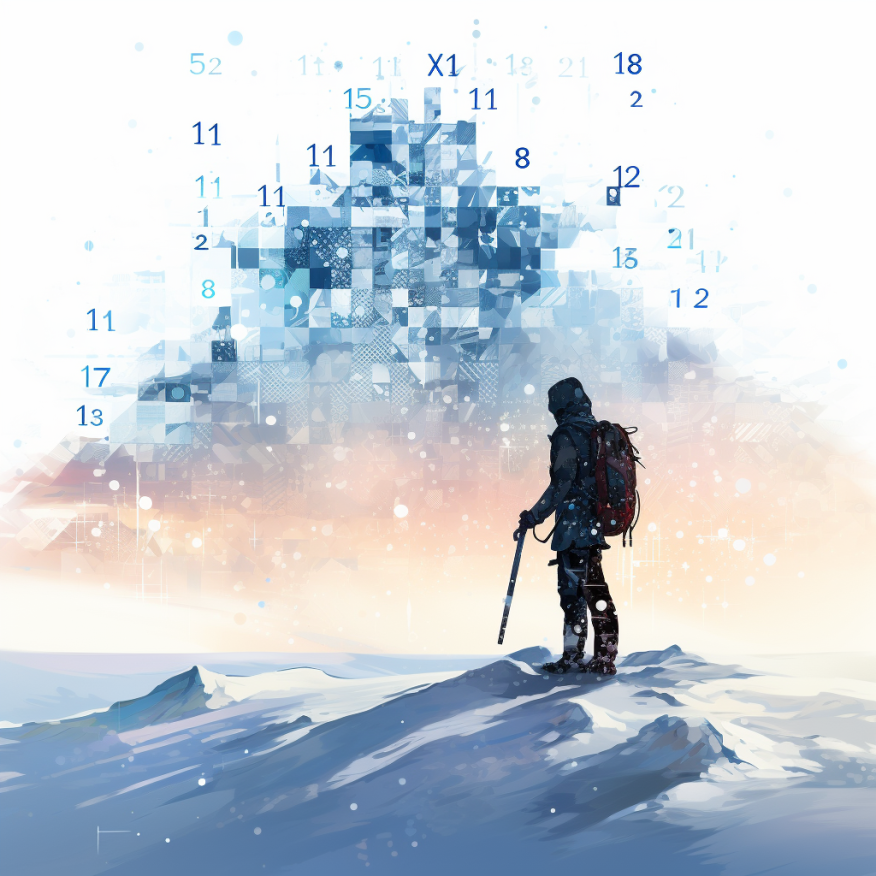
Sponsored By: Fraction
This essay is brought to you by Fraction. The best developers already have jobs, so why not work with them fractionally? If you are looking to scale your startup without breaking the bank, Fraction is here to help. We connect you with fully vetted, US-based senior developers at a fraction of the cost.
Legend has it that in the winter of 1913, explorer Ernest Shackleton put out an ad for sailors to join him on an expedition to Antarctica:
Men wanted for hazardous journey. Low wages, bitter cold, long hours of complete darkness. Safe return doubtful. Honour and recognition in event of success.
Today, in September of 2023, I’d like to propose a similarly adventurous job ad for the age of AI:
Talented engineers, designers, and copywriters wanted for a new agency. All work will be recorded, labeled, and organized for AI training. Your role will be progressively phased out. Salary paid and profits shared to you indefinitely. Failure likely. In the event of success: the last job you’ll ever have—or need.
Here’s the idea: I think there’s an opportunity to start an agency that recruits extremely talented people to train AI by promising them it will be the last job they’ll ever have.
Agency employees will do client work, like engineering, design, or copywriting. They will also record and label the entire process from start to finish as input to model fine-tuning. The agency’s goal is to progressively phase out each employee by training the model on their work. And the employees are in on it! Everyone wants to be replaced because—if it works—they get to keep their salary and upside in the form of dividends, for the life of the business.
A professional services firm that’s structured in this way might have a margin profile that looks a lot more like software (good) than consulting (bad). It could become what The General Partnership investor Ben Cmejla described to me recently as a “mullet consultancy”: from the front it looks like a services business with salespeople and account managers, and from the back, it looks like a software business because most of the actual work is done by the AI.
If a model like this works, it will have broad implications for the types of professional services businesses that can be built, how much they can scale, and how employment agreements should be structured.
Scale Your Startup with Experienced Fractional Developers
With Fraction, you can tap into a pool of fully vetted US-based senior developers at a fraction of the cost. We bring you the best talent without breaking the bank.
Our developers are MIT-vetted and experienced in AI and LLM. They're ready to help your business grow, whether you need assistance with coding, software development, or project management.
Forget offshore outsourcing - work with top-notch developers right here in the US. With Fraction, you can accelerate your startup's growth and stay ahead of the competition.
Ready to take your startup to new heights?
Professional services businesses with software margins
The professional services industry is a gigantic part of the economy—generating $2 trillion in revenue in 2019. It’s a ripe playing ground for first-time entrepreneurs looking to get a business off the ground. Tiny, Andrew Wilkinson’s now-public software-holding company, started as a consultancy. So did Jason Fried’s 37 Signals, makers of Basecamp. If you’re a talented and motivated individual, it’s usually a lot easier to sell your time for money than it is to make a product.
But this comes with a tradeoff: consultancies are maddeningly hard to scale. Despite decades of technological advancements, professional services have been more or less immune to the productivity gains that software promises. You’re trading your time for money, and each employee at a consultancy can only handle so many clients.
Even if you do manage to get to scale, the business you can build isn’t great by software standards. For example, Accenture, one of the largest consulting firms in the world, has more than 700,000 employees and did $61 billion in revenue in 2022. That’s a revenue per employee of around $87,000. Meta, on the other hand, has 70,000 employees and $116 billion in revenue. That’s a revenue per employee of $1.6 million—almost 20x Accenture.
It will probably be a long time before firms as large as Accenture are automated. However, there are probably hundreds of thousands of smaller professional services firms in the U.S. that could use AI to significantly change their revenue-to-employee ratio—and their margins. Over time, certain categories of professional services may end up having a margin profile that’s more similar to software than it is to consulting.
If AI does create room for these kinds of consultancies, how do you make one that produces great work?
Better training data means better quality outputs
Improvements in foundational models may mean that anyone will be able to get high-quality professional services like engineering, design, or copywriting work done without engaging an outside firm. But I’d bet coaxing models to produce differentiated high-quality output will remain a professionalized skill that involves talented humans in some way for a long time.
The question is: if you’re running a consultancy, how do you get excellent-quality, differentiated-model outputs that allow you to offload as much of the process of creating work as possible to an LLM? I bet that recruiting people of exceptional talent and incentivizing them to spend part of their time training a model might work.
If you can collect a large enough corpus of properly labeled demonstrations of different processes that occur as part of delivery to a client, you can probably get a model to reproduce those demonstrations with some combination of prompting and fine-tuning. If the demonstrations are of extremely high quality from smart people, you’ll probably get significantly better results.
You could start by getting the models to learn small pieces of the workflow that take a significant amount of time—maybe crafting a proposal, or coming up with a good headline—and over time move to more complex processes. This is already a common idea in AI circles, called factored cognition.
What's more, many professional services firms already attempt to do something like this today by “productizing” the work they do: reducing complex tasks into simple, repeatable processes that can be done by less experienced employees. But this always sacrifices quality and flexibility. AI might allow firms to productize without those trade-offs.
That brings us to a new question. How do you get talented people to want to automate themselves?
Employment contracts will have to change
Employment contracts are currently based on a fundamental proposition: in order to keep getting my work, you have to keep paying me. That may no longer be true in an AI-first world. When future work product can be produced with enough up-front demonstration data, what’s fair?
Probably the best thing to do is to pay people in an ongoing way for the value that their data generates. If the goal is to get people to do enough model training to replace themselves, then ideally, at the point at which their work is automated, they continue to get paid their existing salary. Because the model they’ve trained will be able to work with many times more clients than they would’ve been able to themselves, they should also participate in the upside.
In this world, agency employees look a little bit more like startup investors. The job of an investor is to identify an interesting opportunity, deploy capital, and help where they’re needed. An investor puts up capital and, in exchange, gets paid when that capital produces more capital.
Instead of contributing money, agency employees contribute data and ongoing expertise. In exchange, as the models go on to do more and better work, the employees get paid. This could create an entire economy of people who—instead of exchanging their time for money—exchange their data for money to fledgling firms looking to add specific skills to their repertoire.
Of course, there are many ways this might go wrong—or not happen at all.
What are the potential pitfalls?
There are a few ways this might go wrong.
AI progress might not happen fast enough
There are many likely in-between worlds where humans are consistently managing AI rather than allowing it to operate fully autonomously. In a world like this, consultancies get more efficient but don’t become fully autonomous. That’s a likely scenario for the foreseeable future—and it’s probably desirable. Moving too quickly to autonomy won’t give employees and governments enough time to adjust.
AI progress may not depend on better training data from humans
It’s possible that human or superhuman performance on professional services-like tasks doesn’t get materially better with specialized data from skilled humans. When I brought this up with AI researcher and Notion AI lead Linus Lee, he raised two important points: 1) there may not be enough data generated by a small consultancy to train a model (and good data might be easier to get through other means), and 2) automating an agency is “a very complex, multi-objective task that is difficult to directly optimize the model to perform with either RLHF or prompting.” Basically, you can’t just train a model on “doing good work.” You’ll have to do a lot of work to break up the tasks into small demonstrable processes and collect end-to-end labeled data of all of the steps—which is hard but doable. Linus was optimistic about using AI to automate specific portions of the workflow for these kinds of tasks, though.
Employment structures might not actually change
The legal structures of capitalism favor investors over employees and are made for a world where employees trade their time for money. Therefore, companies may be able to extract training data from employees in ways that don’t compensate them in an ongoing way.
This question is already being brought up by writers who are concerned about the use of their work at the foundational model layer of the stack—and are suing OpenAI over it. It’s pretty clear we don’t yet have social or legal norms for how to deal with this new world, but rethinking how we structure employment contracts is going to be a big deal in the coming years.
Who’s going to do this first?
These days, it’s a hot thing on creator Twitter to start consultancies. Everyone with a YouTube account or newsletter has their own editing firm or ghostwriting agency. These kinds of companies are an excellent way to monetize hard-won skills and reputation.
A big business opportunity is to think about how to augment and scale the work of these agencies with AI. It’s incredibly important—and potentially lucrative—to find ways to do this that compensate talent appropriately for what they contribute to the AI that comes out of their work.
It’s possible, and desirable, to create win-win scenarios for agencies and employees to build creative, useful AI models.
And if they succeed, to make that the last job they’ll ever have—and will ever need.
Find Out What
Comes Next in Tech.
Start your free trial.
New ideas to help you build the future—in your inbox, every day. Trusted by over 75,000 readers.
SubscribeAlready have an account? Sign in
What's included?
-
Unlimited access to our daily essays by Dan Shipper, Evan Armstrong, and a roster of the best tech writers on the internet
-
Full access to an archive of hundreds of in-depth articles
-
-
Priority access and subscriber-only discounts to courses, events, and more
-
Ad-free experience
-
Access to our Discord community
Thanks to our Sponsor: Fraction
Thanks again to our sponsor Fraction. Forget expensive offshore outsourcing. With Fraction, you can tap into a pool of talented developers right here in the US for a fraction of the cost. Our team specializes in AI, LLM, and more, ensuring you get the expertise you need to succeed.
Scale your business quickly and efficiently while saving on costs. Join the ranks of successful startups who have already leveraged the power of Fraction.
Comments
Don't have an account? Sign up!
Another winning piece from Dan! I love the context you see around the issues you choose. Here's why I think we are a long, long, long way off from AI doing our job. When I turned 60 I thought now is the time to release to the world those 13 filing cabinets full of content that everyone has been wanted to steal from me. Because I no longer need the money and this is a good time to leave a legacy, I'll just give it away and see what thousands of other professionals do with it. As it turned out, all said "We don't want to work as hard as you do. Plus most of this is handwritten or printouts of digital files no longer accessible.
As a copywriter more than happy to obsolete myself if I get paid to do it, count me in
This is THE Idea.
One I am willing to to take the leap on.
If there are further details or updates - Please count me in!
Agree! As a designer, i’m more happy working with AI / system than working directly with human and their biases haha (i love human but for the sake of efficiency at work). For the most part of design can be automated since it’s only happened in digital world. Where’s the CTA button to get in? 🖐️
I love this concept and often find myself wondering why the Accenture-like organization I work for isn’t building massive labs to do just what you’re describing. The only issue you overlooked is the race to the bottom. The early firms will reap the margins and enjoy the near endless scalability for a period of time. But as more firms copy this model and build the infrastructure to deliver quality outcomes, the service becomes commoditized, and these once profitable businesses are forced to lower their prices over and over again. And one day, you’ll be able to buy a messaging framework, positioning strategy, branding package, UX/UI design, and development for your app for the price of an iPhone SE.