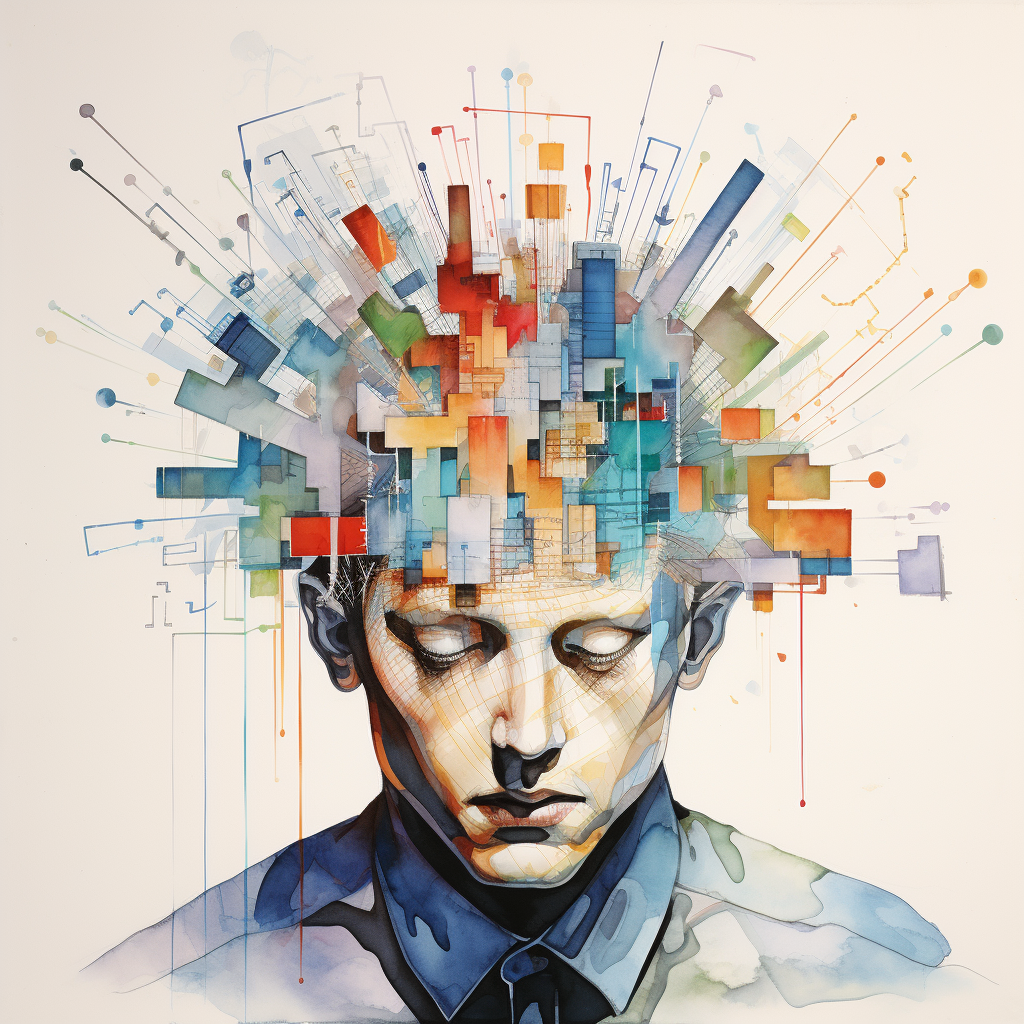
If there’s one question I’ve been obsessed with for the past six months, it’s this:
How might AI change the way scientific progress happens? In particular, how might it help us make progress in areas of science where progress has historically been slow, like psychology or other fields of social science?
I’m not the only one thinking about this. Demis Hassabis, the founder of DeepMind who is currently leading AI at Google, is famous for saying, “Just as mathematics turned out to be the right description language for physics, we think AI will prove to be the right method for understanding biology.”
I love the idea of AI as a new language for describing and solving problems in the world that traditional scientific methods have had a hard time cracking, which I’ve been writing about a lot lately. AI allows us to predict phenomena in the world before we have scientific explanations for them. For example, there is no unifying scientific theory for depression. But AI and machine learning techniques might be able to predict when someone is going to experience depression, which could help with prevention and treatment. This is a significant advance because we can make progress on the disease without needing to uncover a universal underlying theory for what it is.
I’ve been looking for researchers who are going down this path—and I found Eiko Fried. Dr. Fried is an associate professor in clinical psychology at Leiden University in the Netherlands who works on how to understand, measure, model, and classify mental health problems. His current research is a five-year project called WARN-D that uses statistics and machine learning techniques to try to predict depression before it happens. Eiko and his team have followed 2,000 students living in the Netherlands for two years, using the students’ smartwatches and smartphones to gather moment-by-moment data about them. They hope that once this project is done they’ll be able to more reliably predict when depression might occur—before it does.
Dr. Fried’s research focuses on the view that depression and other mental illnesses are complex, dynamical systems, rather than clear-cut categories with simple causes.
We had a wide-ranging conversation about the role of explanations and predictions in science, why many areas of science—particularly psychology—have struggled to make progress, the role of machine learning and AI in scientific research, and how his research is advancing our ability to both predict—and explain mental illnesses.
If you want to listen to this interview as a podcast, it’s available here:
This conversation has been lightly edited for clarity.
DS: Your research is about understanding, measuring, modeling, and classifying what mental disorders are. What are mental illnesses?
EF: What is the nature of mental illness? [That’s] the holy grail in our field that many scholars have ignored actually to some degree, because it's probably very tricky to answer. I think mental health problems are emergent. So they come out of systems of things that interact with each other. And these things that interact with each other are complex systems, and the elements are biological, psychological, and social. And I think most folks would agree with it, actually, it's not necessarily a very controversial idea, but putting this into sort of research or clinical practice is quite tricky because you have all these elements and systems, and then you have all these nonlinear relationships in which these elements interact with each other. And then where do you draw the boundary around the system?
There's the person system, so to speak, with your thoughts and behaviors and feelings, and your genetic setup and so forth. But there's your partner who influences you and your family history and your folks and life events and stressors, and all of that is part of what I think to be the mental health system of a person and your current state.
DS: That makes sense. It's so interesting because I think everyone sort of agrees with that story, or not everyone, but a lot of people would say they're emergent and it's sort of bio-psychosocial. It's a combination of all these things, and the combination is probably different for different people.
If you ask me what the orbits of the moon is, I have an equation. Do you think we'll ever get to a place where we're going to get down to that level, or that there's this very high-level story you can tell and then the details for each individual person are so complicated that having an explanation is going to be hard? An explanation that's compressible is going to be hard to find, or are you looking for that explanation?
EF: Right. So I have two answers. They're quite different answers. The first answer is that there are folks who are working on formal theories of mental health or mental disorders. They don't take everything into account and they probably will never be like Newton's theory of gravitation—which also ended up to be false, by the way. So maybe that's also okay in a way. In addition I think our models or theories are probably going to be useful idealizations. I like to use the map of Rome or the tube map of London as an example, where the map is useful for the purpose that you designed [it] to be, such as navigating the Metro system in London or finding your next Starbucks in Rome. So a good model is one that sort of leaves out unimportant stuff. But, of course, then the question is, what is unimportant to leave out? But the main point is that there is work happening right now on formal theorizing. We have a paper on panic disorder led by Don Robinaugh, for example, which is basically a system of eight or nine nodes or bio-psychosocial variables that have been shown to be really relevant to panic disorder.
We worked on panic disorder first because if you draw 50 random researchers on panic disorder from around the world to the table, most of them will actually agree on the etiology and phenomenology of panic disorder, which is not the case for some other mental problems. So we started there, and the model is basically a formal theory, a formal model, and their equations. And then you can simulate data from the model. And then you can see if the data you get for a person with panic attacks, for example, corresponds to data we observe in the real world. You can see, [what] does the phenomenology of panic attacks look like? OK, they're brief, check that they should be pretty brief. Panic attacks don't last for half an hour or three hours.
Can you simulate interventions using behavioral therapy on the system, and panic attacks become less? Yeah, you can actually do that. But we also find, for example, that there are people who have panic attacks without developing panic disorder. And in our model, everybody who gets panic attacks gets panic disorder. So [we’re] showing you that there's also limits to these theories, and it's a very initial model. But in principle, there's work on theorizing using differential equations.
And I think that work is promising, although it is far away from being a Einstein's theory of relativity. I think it is a model to begin with. And it was indeed quite tricky to decide what's in the model, what's not, what is just important enough to warrant modeling. That's my first answer.
The second answer is, there's work on dynamic properties of systems. This work argues that it actually doesn't matter too much what particular nodes you assess in your system, as long as all of these nodes tap into the dynamics of the system, because it is measuring the dynamics that give you information about the system and not necessarily all the rest.
A researcher in our field has a really cool paper talking about the two worlds of psychopathology. In it, he shows that he has a couple dozen people undergoing psychotherapy. They use a system where they ask people once or maybe multiple times a day about their moods, feelings, thoughts, behaviors, and ecological momentary assessment. They track them for multiple weeks. And the cool thing is that every person gets different variables assessed. Everybody's different. They all agree with their own clinician on what is most central to their psychopathology, even if the diagnosis is the same. Some folks sleep too little, some sleep too much, even if they have the same diagnosis. Some people are sad, others are suicidal and so forth. The analysis in the paper shows that you can, independent of the content of the network or the system, use these dynamical principles to see if people are going to get better or not.
Now, this needs to be replicated, obviously, and we need better methods and other tools to look into this. But I think [it’s] also a nice approach to look into this general idea of complex dynamics rather than the content of the system.
DS: That's really interesting. I hear you on, rather than looking at the content of the nodes, so rather than looking at, for me, maybe I sleep too little. I know that I sleep too little. And if I sleep too little, that increases my symptoms. You're actually looking at, it sounds like, the relationship between nodes. What are some examples of nodes? And then what are some examples of relationships? How would you look at the relationships independent of the nodes as a way to assess things?
EF: So nodes in the system, such as the ones in the paper, and also the work we do, is thoughts, feelings, behaviors, and mental health-related, usually—affect states, sad mood, anger, sleep problems, activity, maybe even using a smartwatch. [It] doesn't always have to be smartphone data. It doesn't have to be self-reported. It can also be somewhat more objective digital phenotyping data. And then you can, in a system, model the relationships between these things. I can see that whenever I sleep really well, I'm relaxed the next morning. Whenever I'm outside or exercise, I'm less active at the next measurement moment. Things like this. You can model contiguous relationships at the moment, but also temporal relationships over time. This works fairly well, using these sort of network psychometric tools that we've developed.
A good example for these dynamics are an early warning sign called “critical slowing down” in the ecology literature, which has been talked about a bit in psychology, but there haven't been super-convincing studies. There're early studies in small populations, but that's part of the reason I think I got my study funded—to see if this early warning sign can be replicated in a large sample for forecasting depression.
The way critical slowing down works, without being super-technical about it, is that when a system transitions from one stable state into another stable state, and when this transition is abrupt, this is important. We'll talk about this later, perhaps because there's also slow transitions, and it doesn't really work that well then. But if the transition is abrupt, like a catastrophic shift, then there's evidence in ecology and cancer biology and economics and other climate science, that the elements of the system change their autocorrelations over time. The system becomes more predictable, and the system moves slower, so to speak.
That's why you say critical slowing down. So translating this to my mental health example, if I know your current mood or sleepiness or concentration or suicidal state right now, and I see that your state tomorrow will become more and more predictable from your current state, we're talking about critical slowing down, which is an early warning sign for an upcoming transition. This has been shown a couple of times in data with depression, for example, in usually just one particular person. There's other dynamic principles, connectivity, and so forth. But this early warning, critical slowing down, is one of the ones that has been discussed the most. If you think of a system like a river, and you can measure the speed of the river using different types of thermometers, this ideographic argument where the content doesn't really matter, the dynamic principles matter. [That] translates into, well, as long as you put your thermometer somewhere in the river, and you pick up some part of the system, that will give you enough information to pick up on changes, and for example, autocorrelations to tap into critical slowing down. If that works or not, we don't know.
DS: That makes a lot of sense. So it sounds like what you're saying is you have a system of interconnected parts. And what you've observed is that there's an abrupt or catastrophic change from one regime to another in the system. Thereafter, that system will slow down, or it will not change as quickly.
EF: Before.
DS: Before. I see.
EF: So the goal of our R&D study is to use these markers as a forecast for an upcoming transition.
If there’s one question I’ve been obsessed with for the past six months, it’s this:
How might AI change the way scientific progress happens? In particular, how might it help us make progress in areas of science where progress has historically been slow, like psychology or other fields of social science?
I’m not the only one thinking about this. Demis Hassabis, the founder of DeepMind who is currently leading AI at Google, is famous for saying, “Just as mathematics turned out to be the right description language for physics, we think AI will prove to be the right method for understanding biology.”
I love the idea of AI as a new language for describing and solving problems in the world that traditional scientific methods have had a hard time cracking, which I’ve been writing about a lot lately. AI allows us to predict phenomena in the world before we have scientific explanations for them. For example, there is no unifying scientific theory for depression. But AI and machine learning techniques might be able to predict when someone is going to experience depression, which could help with prevention and treatment. This is a significant advance because we can make progress on the disease without needing to uncover a universal underlying theory for what it is.
I’ve been looking for researchers who are going down this path—and I found Eiko Fried. Dr. Fried is an associate professor in clinical psychology at Leiden University in the Netherlands who works on how to understand, measure, model, and classify mental health problems. His current research is a five-year project called WARN-D that uses statistics and machine learning techniques to try to predict depression before it happens. Eiko and his team have followed 2,000 students living in the Netherlands for two years, using the students’ smartwatches and smartphones to gather moment-by-moment data about them. They hope that once this project is done they’ll be able to more reliably predict when depression might occur—before it does.
Dr. Fried’s research focuses on the view that depression and other mental illnesses are complex, dynamical systems, rather than clear-cut categories with simple causes.
We had a wide-ranging conversation about the role of explanations and predictions in science, why many areas of science—particularly psychology—have struggled to make progress, the role of machine learning and AI in scientific research, and how his research is advancing our ability to both predict—and explain mental illnesses.
If you want to listen to this interview as a podcast, it’s available here:
This conversation has been lightly edited for clarity.
DS: Your research is about understanding, measuring, modeling, and classifying what mental disorders are. What are mental illnesses?
EF: What is the nature of mental illness? [That’s] the holy grail in our field that many scholars have ignored actually to some degree, because it's probably very tricky to answer. I think mental health problems are emergent. So they come out of systems of things that interact with each other. And these things that interact with each other are complex systems, and the elements are biological, psychological, and social. And I think most folks would agree with it, actually, it's not necessarily a very controversial idea, but putting this into sort of research or clinical practice is quite tricky because you have all these elements and systems, and then you have all these nonlinear relationships in which these elements interact with each other. And then where do you draw the boundary around the system?
There's the person system, so to speak, with your thoughts and behaviors and feelings, and your genetic setup and so forth. But there's your partner who influences you and your family history and your folks and life events and stressors, and all of that is part of what I think to be the mental health system of a person and your current state.
DS: That makes sense. It's so interesting because I think everyone sort of agrees with that story, or not everyone, but a lot of people would say they're emergent and it's sort of bio-psychosocial. It's a combination of all these things, and the combination is probably different for different people.
If you ask me what the orbits of the moon is, I have an equation. Do you think we'll ever get to a place where we're going to get down to that level, or that there's this very high-level story you can tell and then the details for each individual person are so complicated that having an explanation is going to be hard? An explanation that's compressible is going to be hard to find, or are you looking for that explanation?
EF: Right. So I have two answers. They're quite different answers. The first answer is that there are folks who are working on formal theories of mental health or mental disorders. They don't take everything into account and they probably will never be like Newton's theory of gravitation—which also ended up to be false, by the way. So maybe that's also okay in a way. In addition I think our models or theories are probably going to be useful idealizations. I like to use the map of Rome or the tube map of London as an example, where the map is useful for the purpose that you designed [it] to be, such as navigating the Metro system in London or finding your next Starbucks in Rome. So a good model is one that sort of leaves out unimportant stuff. But, of course, then the question is, what is unimportant to leave out? But the main point is that there is work happening right now on formal theorizing. We have a paper on panic disorder led by Don Robinaugh, for example, which is basically a system of eight or nine nodes or bio-psychosocial variables that have been shown to be really relevant to panic disorder.
We worked on panic disorder first because if you draw 50 random researchers on panic disorder from around the world to the table, most of them will actually agree on the etiology and phenomenology of panic disorder, which is not the case for some other mental problems. So we started there, and the model is basically a formal theory, a formal model, and their equations. And then you can simulate data from the model. And then you can see if the data you get for a person with panic attacks, for example, corresponds to data we observe in the real world. You can see, [what] does the phenomenology of panic attacks look like? OK, they're brief, check that they should be pretty brief. Panic attacks don't last for half an hour or three hours.
Can you simulate interventions using behavioral therapy on the system, and panic attacks become less? Yeah, you can actually do that. But we also find, for example, that there are people who have panic attacks without developing panic disorder. And in our model, everybody who gets panic attacks gets panic disorder. So [we’re] showing you that there's also limits to these theories, and it's a very initial model. But in principle, there's work on theorizing using differential equations.
And I think that work is promising, although it is far away from being a Einstein's theory of relativity. I think it is a model to begin with. And it was indeed quite tricky to decide what's in the model, what's not, what is just important enough to warrant modeling. That's my first answer.
The second answer is, there's work on dynamic properties of systems. This work argues that it actually doesn't matter too much what particular nodes you assess in your system, as long as all of these nodes tap into the dynamics of the system, because it is measuring the dynamics that give you information about the system and not necessarily all the rest.
A researcher in our field has a really cool paper talking about the two worlds of psychopathology. In it, he shows that he has a couple dozen people undergoing psychotherapy. They use a system where they ask people once or maybe multiple times a day about their moods, feelings, thoughts, behaviors, and ecological momentary assessment. They track them for multiple weeks. And the cool thing is that every person gets different variables assessed. Everybody's different. They all agree with their own clinician on what is most central to their psychopathology, even if the diagnosis is the same. Some folks sleep too little, some sleep too much, even if they have the same diagnosis. Some people are sad, others are suicidal and so forth. The analysis in the paper shows that you can, independent of the content of the network or the system, use these dynamical principles to see if people are going to get better or not.
Now, this needs to be replicated, obviously, and we need better methods and other tools to look into this. But I think [it’s] also a nice approach to look into this general idea of complex dynamics rather than the content of the system.
DS: That's really interesting. I hear you on, rather than looking at the content of the nodes, so rather than looking at, for me, maybe I sleep too little. I know that I sleep too little. And if I sleep too little, that increases my symptoms. You're actually looking at, it sounds like, the relationship between nodes. What are some examples of nodes? And then what are some examples of relationships? How would you look at the relationships independent of the nodes as a way to assess things?
EF: So nodes in the system, such as the ones in the paper, and also the work we do, is thoughts, feelings, behaviors, and mental health-related, usually—affect states, sad mood, anger, sleep problems, activity, maybe even using a smartwatch. [It] doesn't always have to be smartphone data. It doesn't have to be self-reported. It can also be somewhat more objective digital phenotyping data. And then you can, in a system, model the relationships between these things. I can see that whenever I sleep really well, I'm relaxed the next morning. Whenever I'm outside or exercise, I'm less active at the next measurement moment. Things like this. You can model contiguous relationships at the moment, but also temporal relationships over time. This works fairly well, using these sort of network psychometric tools that we've developed.
A good example for these dynamics are an early warning sign called “critical slowing down” in the ecology literature, which has been talked about a bit in psychology, but there haven't been super-convincing studies. There're early studies in small populations, but that's part of the reason I think I got my study funded—to see if this early warning sign can be replicated in a large sample for forecasting depression.
The way critical slowing down works, without being super-technical about it, is that when a system transitions from one stable state into another stable state, and when this transition is abrupt, this is important. We'll talk about this later, perhaps because there's also slow transitions, and it doesn't really work that well then. But if the transition is abrupt, like a catastrophic shift, then there's evidence in ecology and cancer biology and economics and other climate science, that the elements of the system change their autocorrelations over time. The system becomes more predictable, and the system moves slower, so to speak.
That's why you say critical slowing down. So translating this to my mental health example, if I know your current mood or sleepiness or concentration or suicidal state right now, and I see that your state tomorrow will become more and more predictable from your current state, we're talking about critical slowing down, which is an early warning sign for an upcoming transition. This has been shown a couple of times in data with depression, for example, in usually just one particular person. There's other dynamic principles, connectivity, and so forth. But this early warning, critical slowing down, is one of the ones that has been discussed the most. If you think of a system like a river, and you can measure the speed of the river using different types of thermometers, this ideographic argument where the content doesn't really matter, the dynamic principles matter. [That] translates into, well, as long as you put your thermometer somewhere in the river, and you pick up some part of the system, that will give you enough information to pick up on changes, and for example, autocorrelations to tap into critical slowing down. If that works or not, we don't know.
DS: That makes a lot of sense. So it sounds like what you're saying is you have a system of interconnected parts. And what you've observed is that there's an abrupt or catastrophic change from one regime to another in the system. Thereafter, that system will slow down, or it will not change as quickly.
EF: Before.
DS: Before. I see.
EF: So the goal of our R&D study is to use these markers as a forecast for an upcoming transition.
DS: I see. So before a big transition, your system won't change as much—it will start to look more and more stable. Is that what you're saying? So what is an example of that? Is it something like, before the onset of depression, I will tend to have more trouble sleeping and that will be a very constant thing rather than like, last night I didn't sleep well, but tonight I'm sleeping okay. And the next night I don't sleep well, but the next night I'm fine.
EF: Right. So indeed, the cool thing is that this is actually independent of severity, right? You can have lower variation in sleep problems in two ways. Maybe you sleep well every night or you sleep badly every night, but the lack of variability translates into higher auto correlations or lower standard deviation over time. So the system becomes more predictable. And that might signal an upcoming transition. People in my field say forecast rather than predict, because predict has this in 10 days, whereas a forecast test is soonish, like a weather forecast, which tend to be pretty bad in the Netherlands. Still, it's going to rain perhaps at some point in the next three days. So yeah, we use forecast at the moment.
DS: Okay. So there's some early data that this might be the case, but we're not sure yet. It's not totally clear.
EF: There's a couple of publications where they show that you cannot forecast the ups there. And a transition occurred. We only know this post-hoc, of course, after the transition occurred. There's a quite famous paper from 2016 where they followed a single participant for over a year every day. It's an open data set. It's quite remarkable. And this person tapered their antidepressants—I think they're a researcher themselves. And they reached out to folks in the Netherlands and said, “Hey, do you want to study me while I taper my antidepressants?” So what they did is they tapered the antidepressant blindly, meaning they didn't tell the person when exactly they switched out the antidepressant for a placebo. And unfortunately this person on day 200 or something pretty drastically relapses into depression. If you look at the mean severity score, the person has low variability in symptoms, and all of a sudden they go back into severe depression, and you don't pick this up based on the symptom scores before the transition.
When I talk to journalists about the warranty system we're building, I always say that measuring wind is probably a bad early warning sign for a thunderstorm or a hurricane, because when the wind starts, it's probably too late already. In the same way, measuring symptoms is probably a bad early warning for depression because when the symptoms start, you're probably already in the onset phase of depression. So they cannot forecast based on the severity of symptoms or the symptoms at the mean level, but based on the autocorrelations of the symptom relations or the affect relations over time.
DS: What's an autocorrelation?
EF: The lag one coefficient of one node in the system to the same node in the system over time. Linear regression of one is univariate. Just one has nothing to do with the system itself per se, just your sleep on your sleep on your sleep over 100 days. If the autocorrelation is extremely high, it means your sleep tomorrow is extremely predictable by your sleep today.
DS: I see. Meaning that you don't fluctuate as you should with the environment. Like I'm not stressed every day, but there's stuff that happens to me sometimes. And if you don't respond to that stuff with stress, that's not normal.
EF: Yeah. In our data, initially we see that a sign for depression might be that people have low mood, independent of the context. We have lots of context data in our data set. Are you with friends, with family at school, at work, traveling in nature? We see that some folks have context-independent, really low mood. That might be a marker for depression, for example.
DS: Isn't that just depression, itself?
EF: Yes. Probably.
DS: That's really interesting. What I'm hearing is one of the things you're theorizing for depression in particular, but maybe for any mental health issue, is [to] just gather evidence along a bunch of different variables about a particular person—how they're sleeping, what their heart rate is, what their mood is, what their thoughts are, what their behaviors are. And if you auto-correlate for each of those specific parameters over time before they enter a depressive state, you'll find that some of them—we don't know which ones necessarily—but some of them will tend to go into a more stable state. And then that will go into depression.
EF: Right. This is one of many early warning signals. This all only works if shifts are catastrophic, like for this particular person I talked about before they really relapsed. Some patients talk about it like a black wave falling over them, but it's very open to question whether depression onset looks like this in most people.
There's very little data because we've only now been able to collect these daily data for months and months in folks. One of my graduate students is actually working on the nature of onset at the moment, just phenomenologically to see how people onset depression.
DS: One of the things that strikes me about this approach is requires the moment-by-moment data. That seems dramatically easier to gather now. Tell me about that. Everyone's got a smartwatch. It's funny that you're mentioning this because I literally built a little text bot that texts me everyday, every hour with a bunch of different questions about me, and then has a readout of it. I haven't been doing any statistics on it, but the overall idea is maybe at some point, I don't know that it generalizes in a scientific way, but it might be helpful for me. I'm curious about it.
EF: So folks in our study are doing this for three months and wearing a smartwatch for three months…It's a pretty basic watch given that I needed to buy a lot of watches from my limited researcher budget, but the watch works.
They also fill out questionnaires four times a day. It's a lot actually. They're only two minutes, very short questionnaires. They have like, I don't know, 15, 20 questions. They're very short. How happy are you right now? One, two, three, four, five, six, seven, stuff like this. At the end of the day, there are a couple more questions about how was your day? What was the worst thing that happened to you today?
Including some qualitative open text fields, people can answer if they want to. They can also opt out, but we do find that people actually like talking about their days quite a bit. We also have a couple of questions on Sunday about reflecting back on the whole week.
I think the biggest insight in our field in the last decade of doing this work is that certain questions lend them. certain questions lend themselves more to certain timeframes. So I asked about very momentary moods four times a day. And at the end of the week, I asked about global stressors, or how well do you think you can deal with stress next week? People do their own forecasting. It's been much easier to collect these data. Passive data come for free, basically. It's a very low burden to participants, many of whom wear smartwatches already anyway. The EMA, the psychological momentary assessment is a bit disruptive sometimes for some participants. And they tell you so. We also assess burden in our study, which we find quite important. It's very little understood why people participate. So our compliance rate is about, I want to say 70% compliance rate of these 360 measurement points in these three months, which is very good for us. But it's unclear to us if that it’s because we pay them a little bit of money or we have an interactive website with a data report. People can log in after these three months and they can interactively explore their data, including network visualizations. They really liked that. We got very positive feedback on that.
We always tell them the more data you give us, the more elaborate and accurate this data report is. So there's ongoing work on this. And we just try to motivate participants based on intuition and the little research out there.
Sorry, I talked about a lot, but not really your question. So I think we can leverage this for daily observations. As you said, already it's quite cheap to assess for researchers and for participants.
Some people love it and other people really hate it. This work is done a lot in clinical populations now. And I remember a talk maybe two years ago by a colleague from Maastricht, I believe, who [was] doing a study on rumination, depression with rumination. Part of the rumination CBT [cognitive behavioral therapy] is to tell people not to ruminate. But they were asked four times a day, have you been ruminating right now? To which most clients responded with no, but thank you for reminding me—now I am ruminating again. So it depends a bit on the context in which you do this sort of research. But we're all finding out at the moment—this is all pretty new territory.
DS: What are your thoughts on the pursuit of these scientific models versus just getting a ton of data and using machine learning algorithms to predict the data? Where is each useful? How do they fit together or not?
EF: That ties into the debate I in my field known as explanation versus prediction, or understanding versus prediction, right? I tend to be on the side where fewer people are in the room about the debate. When there are a lot of prediction people in the room around me I do make the point for theorizing and summarizing the point, as the best theory is a true theory or the most useful theory is a true theory. If you really, truly understand the system, it will help you greatly with making predictions, right? So that will be really tricky in psychology, but in principle, I don't think we've done it enough to just give up on it.
I saw this on Twitter just two days ago, a screenshot of a, I want to say 1960s or ’70s book where the author argued, yes, many people have said psychology is too complex and we should give up. They always say physics, or I don't know, Einstein or Newton, but he said, remember that we've observed the stars for 5,000 years, very thoroughly—hundreds of scholars, probably more, have made incredibly rich discoveries about the motion of the planets for Newton to come up with his formula, or for Einstein to come up with his. It's not like physics is easy. It's just that it had a head start. So that's one perspective I have.
The other is that accurate prediction can work without understanding. I always use the tides as an example. We understood the regularity of the tides. We could predict the tides really well, centuries, probably thousands of years before we had any understanding about the mechanisms governing the tides, right? So this is certainly possible.
We were lucky to receive this grant on the WARN-D study. I think in part it got funded because I wrote both into my proposal. I'm well equipped to do both the theorizing and using well-known early warning signs from ecology, for example, that we can simply test in our data, which is theory-driven. But in addition to that, I can use all my data and fit machine learning or AI models to see if there are features in the dataset that predict onset better than others, and then find out what these features might be. I think there's a place for both. And I would be sad if psychology or clinical science would give up on either. I think we do much more prediction than we do explanation. I'm not sure that's great, but I personally do both, and I'm really excited about doing both.
DS: That's really interesting. You're the researcher, so you tell me, but I would have thought that in general, we're doing way more explanation and theorizing than we are doing prediction. If you look at the body of psychology research over the last 100 years, the place it starts is not really scientific or mathematical at all in terms of prediction. It's just theory. If you go look at your recent blog archives, for example, a lot of what you're doing is taking psychology research that purports to predict something and saying it actually doesn't. It's an interesting test of theory, but the statistics that they've gathered don't adequately predict the thing they think it's going to predict.
EF: I have a different take, but I understand your take. About three or four years ago —actually probably six at this point, it's 2023 already. I always think, and for some reason, my brain is in 2020, it's like seven years ago in 2013.
DS: I'm a similar way.
EF: When I started working with Don Robinaugh, who came to Amsterdam, we shared an office for a year, and it was a very rich year, I think for both of us. We started working on these formal theories and dug ourselves into literature. It quickly became clear that, and I think this is quite widely established among folks who do this sort of work, that I wouldn't call most psychological theories, theories, actually, they're sort of these vague, imprecise narrative descriptions, which cannot really be corroborated or rejected.
We have a scholar in our field who was well known for being quite on the nose with his criticism. He famously said that psychological theories don't get rejected or refuted, they just slowly fade away as theorists die—Paul Meehl. And I think that is true to a large degree. If you look at the most popular theories at the moment in social science, if you try to formalize it, the way we do that with the panic model, it would be impossible very quickly, because the theorists don't really spell out auxiliary assumptions of the theory. And if you don't see that people are testing and trying to falsify these theories in social psych, for example, you can see that the most common rebuttal of a theorist is to say, oh, but that's not what I meant. Then you ask them, what did you mean? And why didn't you say this in your paper in the first place?
So that's why I didn't really think of these theories as theories in the way I was talking about them. Hence, I think we're focused much more on prediction, logistic regression, and any sort of statistical model than the phenomena that we try to explain.
DS: Got it. How does p-hacking and the replication crisis fit into this, in your view?
EF: You're really well informed, I have to say. To become tenured psychology faculty in the U.S., it's quite important that you have your own theory contribution. But it is mostly like a theory contribution, to be fair. I'm actually writing a paper about this.
I don't know how to do it, but I've long had the intuition that in psychology, people are more married to their theories than in other fields. I think the reason is that we don't formalize our theories. So with the panic model by Don Robinaugh, this is written up as a differential equation in our code. It's on the website. It's wrong. Because any formal theory, I think in our field will be wrong for the reason that it's incomplete. You never model all the nodes in the system that you truly need, but that's okay for a model. But it is false in the sense that it is incomplete. We call on people to take this code to make it better to add other nodes to reject our model to test it and so forth. That isn't really possible with some of the verbal theories we see in my field. And I think that opens the door to these questionable research practices, replication problems and reproducibility issues that you mentioned before.
DS: I feel like the introduction of statistics in psychology research was intended to make it feel more scientific. But it feels like in a lot of ways, people use it tojust dress up their narrative theories, but the statistics don't actually do the thing that they intend them to do. Does that feel that that's true? Or do you have a different perspective?
EF: I wrote two papers on this exact point in the last few years, which took me a long time to write because I got quite deeply into the philosophy of modeling and philosophy of
theory and what the difference is. My take now is that one of the biggest challenges is to try to bring your data to bear on your theory. When I explain this to students, I always use linear regression. I learned as a student that linear regression has an assumption, namely that variables need to be related linearly. But I never really got my head around truly what it means that a model has an assumption. How I think about this now is that if your theory predicts a linear relationship, then you should use a model that imposes a linear relationship on your data that you can then try to bring the data to bear on the theory. That's sort of the circle, but we don't have theories to impose assumptions on data to then bring them to bear on our theory so that people just use statistical models. We use linear regression. There's no justification for it. That's not easy, but I think it's indeed a challenge that we use models. And in my paper, I talk about a couple of areas of psychology, like factor models, for example, that everybody uses, but I rarely see the rationale for why these models are the right models to bring the data to bear on your theory. And if that step isn't taken, things get tricky very quickly.
DS: I've read a couple of the things you've written, recently. Can you explain what you mean by the terms “equifinality” and “multifinality”?
EF: Equifinality is the principle that in open systems, a given end state can breed from many potential starting states. Multifinality means different outcomes from the same start.
[Editor’s note: equifinality means that there may be many different causes of the same end state—like depression. Multifinality means that the same cause could result in different end states. For example, the same childhood trauma may trigger depression in one person, but not another.]
DS: I don't know if you're familiar with David Deutsch's work. He's doing the philosophy of science. He's very inspired by Popper, really into falsification and his underlying idea, or what makes something scientific, is A, that you have a theory that's falsifiable, and B, that your explanation for that theory is hard to vary. So if you change any of the elements of the theory, you get a different result. And one of the things that's come to mind for me is that may make sense in physics or chemistry. But if you're working in a system where equifinality or multifinality is in play, them being hard to vary, it's sort of impossible because you can have the same result from multiple different starting conditions, or you can have a different result from the same starting condition. And I'm curious how you would either square the idea of falsification and hard-to-vary explanations with working with these types of systems or not.
EF: That would be such a good exam question. In our exams, we always have this extra question where you need to think really hard. And it's a good one. That's a curveball. Very interesting. I wouldn't know how this would translate to the work we're doing in complex systems, because I wouldn't know [what] this variation would look like in particular. I do believe that, of course, people differ from each other.
But yeah, a good theory is one where initial parameters of the model shouldn't be varied or would be hard to vary because you would get a different outcome.
DS: It's an important question because I think it strikes at the heart of— for me, something that's an interesting question is, does science have to differ for if we're working in these high-dimensional, multi-variable, complex systems-type cases than it does in the regime of physics, for example? And does that change how we should try to understand and predict and treat mental disorders? So to lay all of my cards on the table. I would love for you to poke holes in this because I know you do the contrarian thing, and I think that that might be where you want to go, and I think I really want to know. Or if you agree, I'm also quite interested.
The thing that strikes me about this, and maybe some of the work that you're talking about with these differential equations for panic—I haven't looked at that, and that seems really interesting. And as someone who suffers from panic, I want to get in that model. But the thing that strikes me about it is it's totally possible that for something heterogeneous like depression, the explanation or the scientific model that predicts it or that helps us to understand it is so large that it's impossible for us to fit it into our heads.
And that rather than looking for that scientific explanation, we should just throw a bunch of data into a machine learning algorithm and then predict it. And what that does is it exchanges a scientific problem for an engineering problem. And it makes it into a very pragmatic thing where it's like, well, we may not be able to fully understand it in the romantic idea of understanding, but if we can build models that predict it, we can change it. That's ultimately one of the key things we're trying to do when we're trying to explain things, is make those predictions. I'm curious how that strikes you and where you think that goes wrong. Because I think if you agreed with it, it really underscores, for example, the importance in psychological research of doing lots of open data studies where you're finding massive, massive, massive data sets and fitting algorithms to those data sets rather than doing lots of small-scale studies that later on you hope to replicate and get more funding for. And I'm really curious about your take on that, as someone who's deeply in the field and probably knows a lot more about it than me, or certainly does. Where does it go wrong? Where is it right? What does it miss? All that kind of stuff.
EF: I'll start with the last point. The easiest to answer in our study, so we're collecting this data for five years, and everything will be open in the end that we are allowed to share. That just simply means I need to remove open text answers and where people might identify themselves. I need to remove data that could be used in the future by very smart AI to identify people in some way. There might be [a] signal [of] neural activity or heart rate. We don't know that yet, so I'll go through a thorough protocol.
But if our project doesn't work out and we can't forecast depression, I genuinely feel so happy about data collection, because I think it'll be a data set to work on for two decades for a lot of folks in the field. I wish this would be more common. There's certainly people who share their data, but it's rare that these big initiative data sets get shared immediately. So I'm super- looking forward to making this available. We've been spending two years on data collection and documentation, just writing code books to make sure other people can use the data properly and documenting all the changes we made to questionnaires, because some of them talked about fax machines, which might not be the right question anymore. And then do it right. How do we translate this and then so forth.
You raised a couple other points before I go to the black-box-machine-learning stuff. In principle, I can see different models being used for different purposes. So there might well be a model for depression. That is the formal theory.
Then there's another model that is different. That is one for forecasting onset. And another model is for diagnosis, for example, or treatment prediction. And they might have different variables in them, and they might work on different patterns. I'm completely okay with this. I'm not looking for the one overarching true model that does everything.
DS: Just to confirm, are you talking about a theoretical model or a machine learning model?
EF: Both. I'm fine with either. So I'm fine if it can be ML as well; it can be black box. And if you were constrained, your machine learning model that you identify to be the best working for predicting diagnosis to the forecasting case, it might not work very well. So I do grant that different models might have different use cases in the very same way that the Google Maps layers for topography or Starbucks overlay or whatever might be useful for different purposes. I really think of models as tools in that sense.
In our panic model, initial conditions can differ. I thought about this a little more now. So I can make—for some reason, we called the guy Panic Bob early on when we simulated data for his person. So Panic Bob, there's an infinite number of Panic Bobs. I can make Panic Bob that no matter how much I ramp up the stressors of life events, Panic Bob will never get panic attacks because he does not have, or they do not have, I should say, a strong relationship between the vicious cycle of physiological arousal and catastrophic over-interpretation of arousal that leads to onset of panic attacks. If I kill these relationships, then nothing can happen. So I can parameterize all these values for different people, and then see under which conditions who gets panic attacks and what conditions make one most vulnerable, and all of that stuff. That goes back a bit to this idea of David Deutsch, perhaps, but I'd have to think about it more. But in principle, the model is an N of one model, and people can differ from each other in the intercept or the relations of the system.
Now, the last point about black box prediction is maybe where the future lies. I think often of Hasock Chang's book, Inventing Temperature, where he talks about epistemic iteration, which I hadn't heard about before. We recently wrote a— Don always calls it jokingly my “magnum opus for depression,” because it summarizes 10 years of work and thinking, and views epistemic iteration as an example of why our field has not progressed. So I'm trying to expand this now and why that has importance for your question.
In Inventing Temperature, Hasok Chang talks about the tricky situation that we don't think about today: that people back then had a sense of temperature, but they didn't know what temperature is, nor did they have a measurement for temperature. So it was really hard. It took many, many smart people, 300, 400 years to get thermometers developed, because we didn't have the theory nor the measurement. And if both are missing, you are in trouble.
So Hasok Chang talks about epistemic iteration, which is the idea that you make really bad model measurements. And then they inform you about a really bad theory, which helps you improve really bad measures. Then you go back and forth between theory and measurement. I like the example of glasses. He says, well, even with really bad glasses, you can see the world in some shape or form, which can help you make better glasses.
In the depression measurement paper, we describe the reason why stuff hasn't progressed in 30 years, and we argue that one of the core reasons is that the measurement tools we use in clinical trials today, the most commonly used scale is from Hamilton in 1960. That was a great scale in 1960, perhaps, but it really doesn't conform at all to any measurement practices 60 years later. That's not how we would develop a scale today. That's not how we validate a scale. So many things that are part of this measurement instrument are quite irrelevant to what we think depression is today. And yet we still use it. And so the epistemic iteration hasn't really happened. We have learned a lot about the theory of depression, but our measures haven't improved. And this is relevant for the black box stuff, because I would raise the question, but what should we measure? You would say, “Everything,” but I'm like, I don't think that's how it works. So I think the most useful measurement will be a theory-based measurement, and then we can still apply black box models.
Actually, we have a protocol paper for the WARN-D study. I think it's the first protocol paper I've read where I spent three pages grappling with the phenotype of depression, the complexities of the phenotype of depression, and then explaining why I chose my very particular measurements, dynamic measurements, for example, to deal with this heterogeneous, multifinal, equifinal, and so forth, all these issues. So black box machine learning is great, but how do you select the data you collect in a study? Because you always need to make a trade-off. In our study, we had, I don't know, nine hours of questionnaires we wanted to give, and we gave 90 minutes. This is all theory-driven, of course—the selection of these 90-minute questionnaires in the end.
DS: We covered a lot of stuff. What did I miss? What should I have asked you that I didn't?
EF: We talked about prediction explanation on Twitter. I think that was what I was going to get into. That's been really exciting. I got to talk about the project we're doing a little, which is nice because my grad students worked super-mega-hard on it. I've been working on it for three years myself. It's nice to chat about a little now that the data are actually coming in and our first cohort is actually ending in December. We have two years of data done in the first 500 people and the others are still running. I just hired a postdoc to look into the data.
This was really insightful because you're also prepared. You knew about the replicability crisis in my field and stuff like this, about mental health, but also about AI and machine learning and David Deutsch and explanation versus prediction. It was really fun to chat.
DS: Thank you very much. I really appreciate your time.
EF: Thanks, Dan.
If you liked this interview, follow Dr. Fried on Twitter.
Ideas and Apps to
Thrive in the AI Age
The essential toolkit for those shaping the future
"This might be the best value you
can get from an AI subscription."
- Jay S.
Join 100,000+ leaders, builders, and innovators
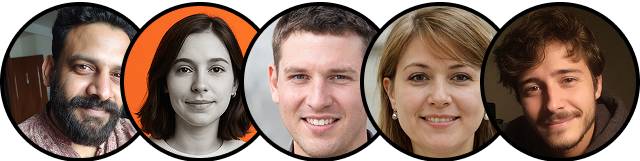
Email address
Already have an account? Sign in
What is included in a subscription?
Daily insights from AI pioneers + early access to powerful AI tools
Ideas and Apps to
Thrive in the AI Age
The essential toolkit for those shaping the future
"This might be the best value you
can get from an AI subscription."
- Jay S.
Join 100,000+ leaders, builders, and innovators
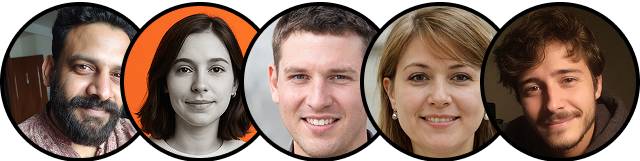
Email address
Already have an account? Sign in
What is included in a subscription?
Daily insights from AI pioneers + early access to powerful AI tools
Comments
Don't have an account? Sign up!