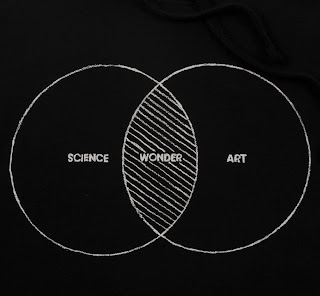
Sponsored By: LiveFlow
This essay is brought to you by LiveFlow, North America’s #1 Financial Analysis Software.
Planning your 2024 Budget but don’t know where to start? LiveFlow has already made the financial models and templates. Budget vs Actuals by Department, 13-week Cash Flow Forecasting, Consolidation… All you have to do is plug and play. Just connect your QuickBooks Online to their models and it’ll be fully set up in minutes. Say goodbye to manually entering financial data. Say hi to automatic updates of your custom models and fully streamlined financial management. Get 20% off for 3 months with promo code EVERY.
LiveFlow, the ideal choice for automation and efficiency. #1 Easiest to use software for Financial Planning & Analysis by G2.
When a new technology becomes consensus but is still somewhat novel or hard to parse, investors often try to distill companies into clear-cut and easily “findable” metrics in order to make decisions.
In many cases, this challenge pushes investors to focus on a consistent factor in startups—founders. And as they search for founder-company fit, they anchor on the next clearest signal—founder pedigree.
This framework can feel valid in technologies that are pre-breakthrough or -Cambrian explosion. Investors are looking for those capable of changing the world and doing something that has never been done before. In order to commercialize these breakthroughs, it’s a commonly held view that the “original” inventors—the scientists who’ve pioneered the breakthroughs—will be necessary to push technology to a production-ready state.
As an industry shifts from pre-breakthrough to post-breakthrough, you might see high-pedigree founders raising staggering amounts of money and capitalizing on investor narrative distillation and hype cycles.
As the first investor in companies like RunwayML, Wayve, and Deepgram, and the managing partner at venture capital firm Compound, this phenomenon is something I’ve seen often—especially in AI over the past 24 months. We live in a post-transformers and post-GPT world. At first, most of the well-funded AI labs and companies were founded by the original authors of the paper “Attention Is All You Need” (Vaswani, Ashish & Shazeer, Noam & Parmar, Niki & Uszkoreit, Jakob & Jones, Llion & Gomez, Aidan & Kaiser, Lukasz & Polosukhin, Illia, 2017), which introduced the groundbreaking transformer model—a neural network that can track relationships in sequential data to learn context and meaning. For example, Vaswani and Parmar went on to found Adept AI Labs, and Shazeer founded Character.AI. There’s now a second wave of stealth companies coming from those early on at these larger labs (or those who were senior hires for short periods of time).
This dynamic is not a rare one. Generally, in the 1-2 years following a breakthrough, incumbent entities rise massively in value, creating golden handcuffs for some and parachutes for others. At that point, early employees often look at the nuanced disagreements they have with the incumbent approaches and gather the confidence to leap into the founder pool. This results in the second-wave dynamic described above.
However, as a given space’s breakthroughs become better understood, those responsible for research breakthroughs might not be the right founders to innovate and capture its value—although it is likely they will be incredible team members. And investors tend to overrate how well these individuals can take a given technology toward a scalable product or down a cost curve while navigating commercialization and GTM.
Picture this: Effortless financial reporting without lifting a finger. LiveFlow is a game-changing platform that automates reporting, saving you over 5 weeks per year of manual work.
With a library of hundreds of financial models, all you have to do is plug and play. LiveFlow will connect to your QuickBooks to update your custom models in Google Sheets.
Join the ranks of those harnessing the power of LiveFlow to get weeks of your time back. Want to level up your finance management? Explore more now with LiveFlow. Get 20% off for 3 months with promo code EVERY.
Understanding how knowledge spreads post-breakthrough
As a founder and investor, the key thing to understand in order to build a successful and enduring company is the rate of proliferation of “key” knowledge in a breakthrough area, as well as how much that knowledge compounds internally and on an industry level.
The canonical example in AI is the number of people able to train models at the parameter size and scale of GPT-4. A year ago, industry watchers would put this number between 50-100. Since then, that number has greatly expanded on the infrastructure side. And this number will continue to grow as people adapt mixture-of-experts approaches—ensemble learning techniques in which different specialized models, or experts, are combined to make predictions. At this point, the wheel will have to be reinvented less often.
Additionally, between 2014-2020, learning within core innovation and architecture in AI spread like wildfire, enabled by open publishing and collaboration between the largest labs. It’s worth remembering that this expansion won’t go on forever—competition, a lack of publishing, more consolidation of talent, and less talent bouncing from lab to lab will certainly change this rate of proliferation. Still, it’s a useful example to look at.
Now that we’re approaching model quality that will be high-value enough for a variety of applications, it’s likely that we will see a similar proliferation of knowledge happen with local (and perhaps <200B parameter) models on the AI side. Most of the collective research is still open, and a large portion of the open-source community is collaborating online to push the limits of base models like LLaMa-2.
Yet it’s also likely that we will continue to see investors over-index on research pedigree, despite the possibility that an understanding of the bleeding edge, a fast pace of shipping, and creativity could be just as successful at pushing forward innovation in this next stage.
On looking for novel techniques
In Compound’s 2022 annual letter, we wrote that we have been looking for novel techniques that might emerge, akin to how creative AI went from GANs to diffusion models for image generation. (GANs deploy dual neural networks to distinguish authentic from synthetic data. In diffusion models, a single neural network reverses noise-infused processes to reconstruct coherent images.) “Could there be something better, like OpenAI’s recently published consistency models? Will transformer models be the final model to rule them all? While it is currently unclear to us what will change, we have a hard time believing that nothing will. We’ll have to keep looking closely.”
These statements are of incredible importance to understand. Will novel paradigms or breakthroughs come from existing players, effectively proving that the best researchers are able to continue to innovate as founders better than others?
It’s likely that linear progress will continue to compound with the talent and capital (and thus compute) moats of the largest research labs. And yet, it’s possible that entirely new architectures or building blocks could create nonlinear breakthroughs.
Even though scaling laws—the inevitable trade-off that many have to make between building larger, more competent networks and incurring the costs that come with them—could limit these breakthroughs, it’s also possible that this innovation push could come from scaling the research done for LLM agents or other adjacencies to model performance. The alignment of this concept was well-covered by Paul Christiano at Alignment Research Center (formerly of OpenAI). We’re also starting to see people try to set up open-source mixture-of-experts communities.
Instead of talent, perhaps the biggest limiting factor will be the politics, technical debt, and social capital that sits within these large groups that have accumulated and spent tens—or hundreds—of billions collectively on a transformer-centric approach.
Being pot-committed helped give us the conviction to partner with autonomous driving software Wayve, which had a non-consensus approach to solving and scaling self-driving. The larger players weren’t going to toss out their traditional approaches—they were seeing linear progress and had allocated billions of dollars of budgets. It took a team of relative outsiders to take a shot on an end-to-end approach in 2017—not the slew of ex-Tesla/Waymo/Cruise people who spun out and raised capital post-Cruise acquisition to build self-driving startups.
Although AI might seem unique in this regard, my gut tells me that as other emerging technical categories start to show obvious breakthroughs, we will see this dynamic play out again across biotech, robotics, and likely other deep tech categories, like quantum and AR/VR.
For example, over the past decade, biotech has historically been funded almost exclusively on pedigree. Now, as it shifts to a more engineering-centric and platform-driven paradigm, we’re seeing interdisciplinary teams that are equally as competent as traditional teams—if not more.
We’ll likely see similar pedigree-driven dynamics emerge within robotics in the coming years. As the progress made in large models and breakthroughs like RT-2 and Code as Policies continue to ripple through the community, investors will likely index heavily towards these labs or look for founders from large labs that have been dismantled or refocused (hello, OpenAI robotics team).
Many of these people will be paramount to creating the breakthrough. And yet, in order to build a venture-scale robotics company, it is not only important to be able to implement advanced hardware and embodied intelligence in the real world, but also to push forward the creative thinking of the modality or use case of a given robot in order to build the next great robotics company.
My advice for those at the top tier labs would be to make sure you are partnering with incredible product people on the founding team.
Our view continues to be that we are in the most important century. We will likely have a large number of “breakthrough” moments in the coming years in deep tech that are near impossible to pattern match.
And as asymmetry and exponentials are becoming better understood, it’s likely that there will be far more volatility—and opportunity—for founders, regardless of classic pedigree. This also means investors should be careful about over-indexing or pattern matching into obvious views of excellence.
I keep returning to the Venn diagram we used to describe our computational creativity thesis, our seed investment in Runway, and perhaps even the age of post-breakthrough, pre-scale innovation.
A mixture of the best scientists in the world doing things that have never been done before along with a different cohort of artists pushing these breakthroughs at scale to create wonder: founding teams building at the intersection of science and art.
Michael Dempsey is the managing partner at Compound, a research-centric, thesis-driven investment firm focusing on emerging technologies. He has been building and investing in the AI space since 2014 and was the first investor in RunwayML, Wayve, Deepgram, and many other companies. This piece was originally published on his website.
Find Out What
Comes Next in Tech.
Start your free trial.
New ideas to help you build the future—in your inbox, every day. Trusted by over 75,000 readers.
SubscribeAlready have an account? Sign in
What's included?
-
Unlimited access to our daily essays by Dan Shipper, Evan Armstrong, and a roster of the best tech writers on the internet
-
Full access to an archive of hundreds of in-depth articles
-
-
Priority access and subscriber-only discounts to courses, events, and more
-
Ad-free experience
-
Access to our Discord community
Thanks to our Sponsor: LiveFlow
Thanks again to our sponsor, LiveFlow. Why hire more when you can do more? LiveFlow shaves 5 weeks off your year by taking care of the tedium of reporting, so you can focus on the work that matters most.
With ready-to-go models and templates, your only task is to connect your QuickBooks. The magic begins when LiveFlow integrates your QuickBooks financial data into custom models in Google Sheets, fully automating your reporting and FP&A analysis.
Uncover a leaner, more efficient way to do financial reporting with LiveFlow – #1 Easiest to use software for Financial Planning & Analysis by G2.
Get 20% off for 3 months with promo code EVERY.
Comments
Don't have an account? Sign up!