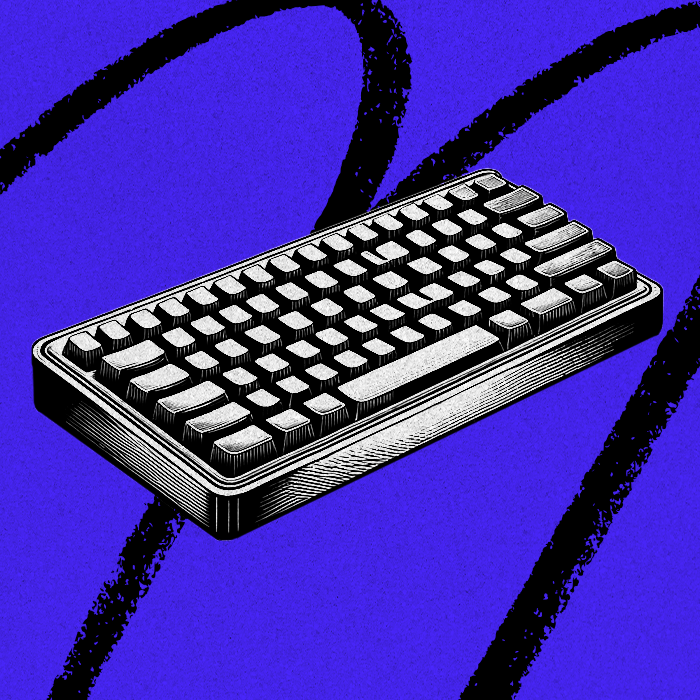
Whenever someone tells me they think ChatGPT isn’t useful, I always assume they just don’t know how to prompt it. Good prompting is the difference between AI that feels like magic and AI that feels mundane. But there are few well-written resources that teach you how to do it properly.
That’s why I’m so excited to have Michael Taylor write this guide for us. He was one of the best TAs in our first chatbot course cohort, and he’s writing a book about prompting for O’Reilly, so he really knows his stuff. If you want to become a great prompt engineer, this guide is a good place to start. —Dan
ChatGPT’s output is the average of the internet. It has seen the best and worst of human work, from angsty teenage fan fiction to the collected works of Ernest Hemingway, and everything in between. But because it is the average, the default response you get is often undifferentiated and bland. ChatGPT is capable of doing almost anything—you just need to ask in the right way.
Finding the right way to ask, or prompt, the model is known as prompt engineering. With the GPT-3 beta in early 2020, you had to hack the prompt to find the right combination of magic words or phrases to trick the model into giving you what you wanted.
As OpenAI released smarter, more sophisticated models like GPT-3.5 and GPT-4, many of these old tricks became unnecessary. As someone who freelances as a prompt engineer and created a popular course on the topic (we just passed 50,000 students!), many people ask me if prompt engineering will even be needed in the future when GPT-5 or GPT-6 comes out.
Sam Altman, the founder of OpenAI, certainly doesn’t think so. “I don’t think we’ll still be doing prompt engineering in five years,” he said in October 2022. OpenAI’s stated goal is to build AGI, or artificial general intelligence: a computer that performs at a human level across every task. When it reaches that point, we can ask the computer to do whatever we want in natural language, and it’ll be sophisticated enough to anticipate our needs.
Yet I don’t believe prompt engineering will disappear. It’s an important skill. Look around at your coworkers (or if you’re working from home, take a look at your Slack or Teams messages). Everybody you work with is, by definition, already at AGI—but they still need to be prompted.
You’re communicating a prompt every time you brief your designer, explain how to do something in Excel to an intern, or give a presentation to your manager. Every manager is a prompt engineer, using their communication and data analysis skills to align the team toward a common set of goals. Even your employment contract is a prompt: a standardized template of language designed to align your behavior with the commercial goals of the organization.
While we might not call it “prompt engineering” in five years, we’ll always need mechanisms to give direction to our AI—and human—coworkers. Those who get good at this set of skills will have an unfair advantage over those who don’t. Let’s dive into how to engineer prompts for text using five simple principles, as well as learn the basics of image generation.
The five pillars of prompting
As AI models get better, a consistent set of useful principles have emerged. It’s no coincidence that these principles are useful for both working with humans and AI. As these models approach human-level intelligence, the techniques that work for them will converge with what works for humans, too.
I first put these prompt engineering principles together in July 2022 and was relieved to see they mapped closely to OpenAI’s prompt engineering guide, which came out a year later. The principles are as follows:
1. Give direction: Describe the desired style in detail or reference a relevant persona.
2. Specify format: Define what rules to follow and establish the structure of the response.
3. Provide examples: Supply a diverse set of test cases where the task was done correctly.
4. Evaluate quality: Identify errors and rate responses, testing what drives performance.
5. Divide labor: Split tasks into multiple steps, chained together for complex goals.
Use these principles as a checklist to run down as you improve your prompt. If you get sufficient results applying the first few principles, you probably don’t need to keep going down the list unless you need the prompt to be extremely robust. Robustness is a requirement if you plan to use it every day or are implementing it in a product.
Let’s work through an example using ChatGPT Plus (GPT-4), starting with a simple prompt for a common task:
In this case, we’re going to make the topic “productivity with time blocking.” Let’s replace the topic variable in curly brackets in the template.
ChatGPT creates a blog post on our topic, but it’s not worth publishing. If we’re just regurgitating the default answer from ChatGPT without adding anything of our own, we’re not contributing anything useful to the internet. So let’s turn to our principles.
1. Give direction: Describe the desired style in detail, or reference a relevant persona.
A common technique in prompt engineering is to copy the style of a famous persona. This is also common when briefing humans on, say, a project. For example, a brand will typically provide a style guide for its agency to follow when creating ad campaigns. ChatGPT is no different: It needs to know what sort of style you like.
We can emulate almost any style that is available in the training data. It’s more likely that a style will be present if there are multiple examples of it on the internet. In this case, we want to emulate the style of Jocko Willink, former Navy SEAL and author of Extreme Ownership.
ChatGPT is trained to avoid violating copyright, and often litters text with caveats and disclaimers to do so. We’ll have to include a few tricks to get around this, such as specifying that we don’t want to mention Willink’s name. It still outputs a disclaimer at the start, but the article itself is of higher quality than before.
This already fetches us better results than a generic prompt. By specifying a persona, we’ve fed ChatGPT a specific angle. Whether or not we choose to go with the military-inspired approach, we’ve found some inspiration and contributed something to the content.
From here, we can get more creative by unbundling some of the attributes that we liked from Jocko Willink and remixing them with other styles to get something new. First, we need to unpack the specific attributes into bullet points, using the following prompt:
Once we know what makes up the style we like, we can use these details to create something new. For example, we might like the motivational aspects of Jocko’s writing but plan to address an audience that works in tech, values a more relaxed vibe, and connects with pop culture references. Make these changes to the writing style bullet points, and insert them in the prompt.
This will take some experimentation. You’ll often find that ChatGPT goes over the top to try and please you. Keep trying different ways to describe your style in the prompt, and you’ll eventually land on something that’s more accurate.
2. Specify format: Define what rules to follow and the required structure of the response.
Giving ChatGPT direction improves the quality of its output, or at least differentiates it from what’s already out there. However, direction is rarely enough to get you the exact format of output you’re hoping for. With the previous prompt, the blog posts we generate are around 500 words, which is too short to rank on Google.
Just like you would brief a writer on word count or structure, you will need to tell ChatGPT how long you want your post to be. Unfortunately, ChatGPT is surprisingly bad at math and can’t count words, so we’ll need to resort to prompt engineering trickery. Ask it to generate an outline first. Then, prompt it to write each section with a minimum number of paragraphs. You can’t get a precise word count, but you can get more or less in the right zone.
The resulting blog post is over 700 words, which is 40 percent longer than what we had before. We’ll address lengthening it soon, but for now, this is good enough.
For most requirements, it’s enough to provide direction and specify format. I rarely need to move onto these next principles. However, if we’re planning to write not just one blog post but 100 or 1,000, we should work on optimizing the prompt further. If you can get 10 to 20 percent more traffic from spending the extra time on prompting, that can add up to tens of thousands of visits across hundreds of blog posts. In addition, having fewer errors saves thousands of dollars spent editing mistakes after the posts are generated.
3. Provide examples: Insert a diverse set of test cases where the task was done correctly.
When AI researchers publish new models or techniques, they typically break down performance by “zero shot,” “few shot,” or “many shot.” “Shot” refers to the number of examples the model was given of the task done correctly. One study shows that adding even one example to a prompt boosts accuracy between 10 percent and 45 percent. And if you have fewer than 2,000 data points, providing examples in the prompt can even beat fine-tuning a custom model.
Adding one example to the prompt (labeled 100 in the chart) took accuracy from 10 percent to 45 percent.
It’s often easier to include an example of something than it is to describe why you like it. However, finding good examples can be expensive—you’ll need to research, write, or generate samples, as well as process the prompt if you’re implementing your work in a product (as of January 2024, the OpenAI API charges $0.03 per 1,000 tokens, roughly ¾ of a word as explained by OpenAI).
Adding examples also comes with a tradeoff between creativity and reliability (how often it follows your instructions correctly). The more examples you provide, the more ChatGPT will follow your lead, improving the reliability of your responses. If you include too many examples or if they aren’t diverse enough, you risk constraining the creativity of the model’s response. It makes sense to experiment with different strategies to strike the right balance.
The examples you include don’t have to be from the real world. They can be cherry-picked from previous ChatGPT responses that you liked, which is known as synthetic data. For this example, I first ran the prompt on the topic of the Pomodoro technique. Then, I asked ChatGPT to extend it five times until it was over 1,000 words. I included the new, longer example post in my prompt for time blocking, and began to get responses that were consistently longer.
4. Evaluate quality: Identify errors and rate responses, testing what drives performance.
This step is the inflection point—it’s where you go from prompting to prompt engineering. For most requirements, it will be enough for you to give direction, specify the format, and provide examples. You can usually stop there. However, many of the clients I work with plan to put their prompts into production, either as a regularly used template for their team or as part of a product that their customers will run thousands of times.
When you’re operating a prompt at scale, you’ll need to know how it’s going to perform across multiple edge cases. What if ChatGPT hallucinates one in every 10 times and includes a made-up fact in your blog post? It will be important to have a system to identify and track these cases. For AI engineers, that usually means implementing a logging system like Weights and Biases or LangSmith, but it can be as simple as copying and pasting responses into Google Sheets and counting how often it went wrong. Once you’re tracking responses, you can optimize your prompt by A/B testing different strategies for reducing errors or increasing quality. We have a number of variables in our prompt to test: We can see how it works across multiple topics, tweak the writing style, or insert one or more example posts. We could try adding an additional instructions section, “you are an expert ghostwriter" to the beginning of the prompt, or “MAKE IT REALLY LONG OR I LOSE MY JOB” in all caps.
Surprisingly, emotional trickery seems to work with LLMs. I get 13 percent longer responses on average when I include the all-caps statement appealing to emotion, as documented in this GSheet template. There have been studies that show LLMs respond to emotional stimuli—for better or worse, they’re simulating the human brain. In the training data, it may have seen that emotional statements in all caps tend to be followed by more diligent responses, so it will try to emulate that.
Simple GSheet Template for A/B Testing Prompts. Source: the author.
The evaluation stage is the hardest. It can be time-consuming and difficult to measure performance. For example, if we were to A/B test two variations of this prompt for five different topics, we’d have to read 10 blog posts to make a value judgment about which was better. There are multiple things we care about that we’d have to check for, like writing style, compellingness, and hallucinated statistics or references.
This is the majority of the work I do with clients. Once you have a robust set of evaluation metrics, you actually improve the results of your prompt when making changes. Otherwise you’re just guessing, and you’re just as likely to harm your results as you are to improve them. Because there is an element of randomness to the process, you’ll get different results every time you prompt ChatGPT. You’ll need to run a prompt multiple times to ensure you weren’t just lucky (or unlucky) with the last response.
The most important and reliable source of evaluation is human ratings—manual reviews done by the prompt engineer, domain experts on the team, and third-party paid reviewers—but it’s also the most time-consuming and expensive. Typically, this method is paired with more automated evaluation metrics, like using code to programmatically calculate metrics with BLEU, ROUGE, or embedding distance, or even asking GPT-4 to review its own work. It sounds crazy to get an LLM to grade its own homework, but there is evidence that it is human-level at some forms of evaluation. And it tends to work well because it’s much easier for the model to identify issues with existing text than it is to generate new text.
5. Divide labor: Split tasks into multiple steps, chained together for complex goals.
No serious approach to automating a task with AI can rely only on a single prompt. Division of labor has been boosting human productivity ever since the hunter-gatherer era, and you’d struggle to find a single person who knows how to make any product entirely end to end in our modern economy. There’s no reason to assume it would be different for LLMs, which give confused results when trying to do too many things at once.
In our blog post task, we can get much better simply by splitting the prompt into two steps:
1. Generate an outline
2. Write each section, one at a time
This gives us a chance to review the outline and make any changes before we have to wait for the article to be generated.
The benefit with an outline is that we can work alongside ChatGPT, giving it inline feedback when we don’t like a section, want to include something important, or need to correct a mistake. This gives us more control over its output and ultimately makes the final product more human, thanks to our ongoing contribution. This AI writing system is still significantly easier than writing the post ourselves, which now takes 10-15 minutes instead of 4-8 hours.
In this case, all of the tasks are done within the same context window or chat session, but for longer or more complex tasks, you might need to start a fresh session. The output of this chat will serve as the input to the next link in the chain, which might involve proof-reading or fact-checking on the final result, without the context the first chat session had (to avoid influencing the rating). In addition, to make the post more valuable, we might ask ChatGPT to browse the web or summarize a document in another session, which then becomes input for this session. Although the limits change all the time, GPT-4 currently has a 100,000-token limit for chat sessions, or approximately the size of the first Harry Potter book.
One day, we might delegate the decision of which subtask to focus on next to an LLM. We might even get it to check its own work. This thinking lies behind autonomous agent projects like AutoGPT, Baby AGI, and Autogen, as well as ChatGPT functionality like the Code Interpreter. They run in a loop, planning, executing, checking their own work, and retrying if they have made a mistake. Unfortunately, these agents are currently unreliable, expensive (because you’re paying through API), and require a lot of technical expertise to set up. If GPT-4.5 or GPT-5 are significantly better at reasoning, expect to see this category of product explode in popularity.
This even works for image generation
So far, we’ve covered text generation and LLMs, but these principles transfer to other modalities as well, like image, video, and audio. The same techniques work across models, including DALL-E, Midjourney, and Stable Diffusion. DALL-E 3 is integrated into ChatGPT, and the LLM actually does a good job of writing the prompt for you. However, you should still apply prompting principles to steer the model towards a more creative output, based on your vision.
We can use what we learned about prompt engineering principles to make it a little more interesting. I want something in the Corporate Memphis style (1. Give direction), with a similar design to this example (3. Provide examples). I also want to make sure the output is an illustration (2. Specify format).
This image is already good enough, but I’ll ask ChatGPT for the prompt it used, and then try that with an alternative model (5. Divide labor), because I prefer Midjourney’s aesthetics. Midjourney gives me four options to choose from (4. Evaluate quality), and can keep iterating on each part of the prompt until I’m happy with the result.
The future of work
My belief is that most of the skills you learn in this guide and during your own experimentation with AI models will be transferable to the future. They’ll be the core of a new form of management science, designed for an age when you’ll have more artificial coworkers than human ones. I’m dubbing this neo-Taylorism, after Frederick Taylor, the father of scientific management.
Taylor pioneered scientific methods to determine how long it should take men to perform a given piece of work, so he could optimize their productivity. His theory, known as scientific management, led to productivity gains throughout the early 20th century. Task-based analysis of efficiency is prevalent today across industries. However, Taylorism fell out of favor due to its tendency to dehumanize workers, ignore their needs, and treat them as parts of the machine.
This is not a concern with workers who actually are machines (at least, until they become sentient). No matter how repetitive or boring a task, ChatGPT will do it a million times without complaint or rest. Better still, you can A/B test your instructions to ChatGPT in ways you couldn’t with a human worker: Do it 100 times this way, do it 100 times another way, and then compare the results.
I believe that we’re on the brink of a new era of productivity, with new levels of organizational design not previously possible. I’m already seeing prompt engineering acting as a gateway drug—as you use AI to automate tasks, you’re forced to think about what success looks like. This also leads to changes in how you measure the success of your human-completed tasks. In many cases, I’m seeing it lead to greater appreciation of what humans can do, while moving them onto tasks that are more interesting, creative, and strategically valuable.
Disruption is coming, and it won’t be evenly distributed. There will be winners and losers, as there have been with every new technological shift. The great news is that this technology is open to everyone: You use it by speaking natural language. Prompt engineering is a unique skill, because AI is slowly learning all the other skills. By working more effectively with AI, you’ll have a higher-leverage impact than was ever possible before.
Mike Taylor is a freelance prompt engineer, the creator of the top prompt engineering course on Udemy, and the author of a forthcoming book on prompt engineering. He previously built Ladder, a 50-person marketing agency based out of New York and London.
Comments
Don't have an account? Sign up!
Been waiting for this one personally - thank you so much!
Very helpful. Thank you, Mike
Thank you, Mike.
Freaking awesome. thanks! We're getting a lot of business in the personal branding space. i found your podcast with Dwarkesh to be super helpful in the 1 hour interview I do to get the data and this prompting guide should help us a lot producing the brand guide from it.