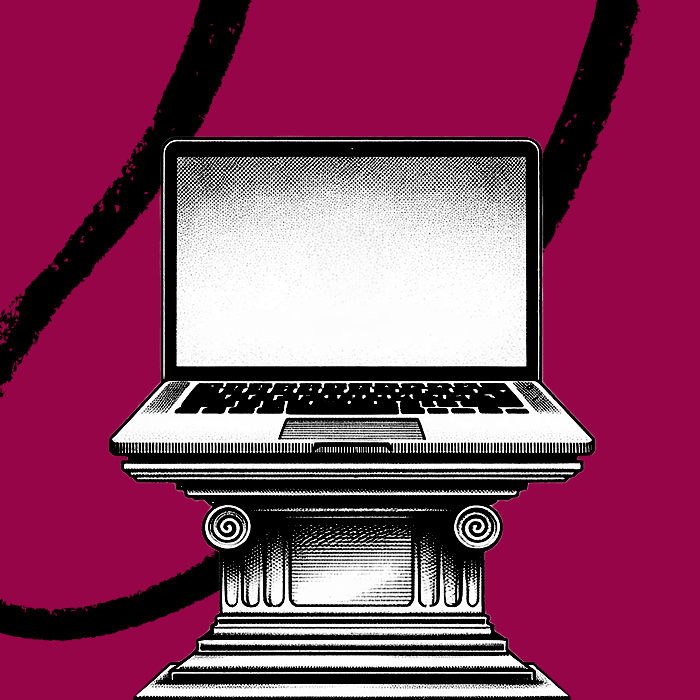
Some of my favorite writing is the kind that I think may be wrong, but makes me check all of my assumptions. The essay is one of those pieces. Will Manidis and John Kennedy—both founders themselves—propose a new theory of software companies, one where SaaS is fueled by low costs and generative AI. I’m not sure I fully buy it, but it did cause me to seriously reevaluate my assumptions about how tech companies will be built and grow in the future. Their thesis is strong, and their writing is compelling. It’s worth reading for a bold vision of what the future can be. —Evan
When we started our companies in the late 2010s, we heard the same advice about how to raise money from venture capitalists in the pursuit of fast growth: Founders would need to raise significant sums of venture capital to incur fixed costs like software developers and sales reps that would pay back over time. Robert Smith, who founded and grew the private equity firm Vista Equity Partners over the last two decades to manage over $100 billion worth of assets, did so on the idea that “all software companies taste like chicken. They’re selling different products, but 80 percent of what they do is pretty much the same.”
Our two companies pursued dramatically different opportunities—John’s offering analytics and automation to school districts, Will’s processing unstructured healthcare data—but the investments we made and pressures we felt were identical. We both raced to build out teams with expensive talent in the hopes of growing quickly, just to raise more money and accelerate the cycle again.
This paradigm is now saturated. Under the surface, software businesses are shifting from fixed cost to variable cost, enabling new high-margin business models and empowering founders with more leverage over the future of their companies. Software is becoming commoditized as a category, and the businesses asset-light. This new model will transform how software companies are built, funded, and operate.
The median bootstrapped B2B SaaS company currently spends around 90% of its annual recurring revenue on costs—25% on go-to-market strategy and execution, 24% on research and development, 15% on administrative and miscellaneous expenses, 13% on website hosting and implementation, and 10% on customer retention. Each of these categories of cost is now a whole ecosystem in the SaaS world. Because “all software companies taste like chicken,” whether you’re selling to hospitals, school districts, or local retailers, you’re tackling the same problems that SaaS companies before you have mastered—except that now there are innumerable LinkedIn thought leaders, expensive consultants, and off-the-shelf products to which you can turn for support.
The SaaS ecosystem has fully matured. Each of those buckets of fixed costs can be selectively ignored, automated, delegated, or consumed as a service. Today, companies can forgo hiring sales and marketing people, and produce millions in free cash flow by selling their products through re-sellers (like AWS) with large client bases. While a custom research and development project used to be the domain of million-dollar contracts, you can now buy a basic Django web app from a Replit bounty for $650 or monetize a scalable business intelligence tool built by AWS or Microsoft. Scaling operations used to require headcount; now all you need are virtual assistants. SaaS products like Zapier or Intercom that power countless vertical market software companies are increasingly charging for their service as consumption: software paid by the task, not, as customarily, by user by the year.
This future was emerging before our current generative AI moment; it will only accelerate if companies can use large language models to automate junior-level work. Generative AI is quickly ushering in a new frontier for software, where organizational structures, cost structures, and growth strategies can and should be re-imagined.
Three theories of an AI-driven SaaS future
The large language models we use, like GPT and Claude, represent an implementation of a general algorithm to simulate human intelligence. The key word is “simulate”: These models do not yet have intelligence—they simulate it, based on an enormous amount of training data. As the quantity and quality of training data increases, the simulations become more accurate, but in the absence of consistently high-quality training data, the models can “hallucinate” and produce a poor impersonation of what a human might say. So despite the rapid advancement, attention to, and speculation about what’s next, this technology is nascent. Even at this early stage, however, a few themes are clear.
Generative AI : content :: internet : distribution
The Internet offers programmatic, global-scale content distribution at no marginal cost. This feature was transformative to our economy and society. It enabled software products to be delivered over a browser and priced as a service (SaaS), instead of delivered on a CD and paid for up front. It connected disparate populations and geographies, as, for example, live meetings could happen on a videoconference instead of in person. As more of our social and economic interactions happened natively to the internet, it fragmented our legacy geographic ties, undercut the institutions of organized religion, and connected romantic partners.
Generative AI offers programmatic, global-scale content creation at a diminishing marginal cost. This feature is an industrial revolution for white-collar work. In the same way that capturing energy enabled the creation of goods with machines, not by hand, programmatic content creation enables an enormous amount of knowledge work to be done with computers, not by hand.
Generative AI represents a classic technological revolution
In Technological Revolutions and Financial Capital, researcher Carlota Perez argued that new technological paradigms—the microprocessor, the internet, the steam mill—follow similar patterns of deployment into the broader economy and society at scale. (You may also want to read this summary of the book or Ben Thompson’s analysis.) These revolutions create financial and political cycles that follow a similar pattern. They begin with a “big bang” that catalyzes a frenzy of investment into installing the technology. This frenzy drives a bubble, which ultimately crashes, but from which emerges a period of steady adoption and deployment.
Source: Technological Revolutions and Financial Capital by Carlota Perez.
Perez wrote her thesis in 2002, but if we were to roughly apply that frame to the internet adoption cycle of the past 30 years, it might look like this:
Installation period: the extended 90s (internet birth to dot-com crash)
- New technological frameworks form, cultural adoption intensifies, and the default interface develops: web browsers
- A full constellation of categories emerge: search, ecommerce, social networking
Financial slump: dot-com crash -> Great Recession
Deployment period: the extended 2010s (Great Recession to 2022)
- Full expansion of innovation and market potential: the maturation of core business SaaS platforms like Salesforce, the growth of infrastructure-as-a-service like AWS, innovative new economic models like AirBnB and Uber as marketplaces
- Last new products and industries: SaaS built for narrow vertical markets (like John’s company building automation software for school counselors) or next-generation cloud computing infrastructure pricing on consumption, like Snowflake
The cycle has recently started anew. We are in the installation period of the technological revolution brought on by generative AI. The launch of ChatGPT was a big bang that drove a frenzy of investment into the space: The $27 billion invested into generative AI startups in 2023 alone surpasses the GDP of Sudan.
New interfaces are quickly developing and solidifying. Most end users of generative AI have interacted with it through chat; natural language seems to be emerging as a standard. And new categories are emerging as companies race to build general models and compete with OpenAI, or enterprises look to build specific, narrow models trained off of their own data.
The financial slump to come is inevitable. Markets are lightweights. We’re all drunk on speculative hype and will be lucky to feel decent in the morning. Many will need to be bailed out of the drunk tank. The return on investment of generative AI is unknown, and while there are clearly real businesses, the unit economics are unclear and hidden under the comfortable blankets of venture capital and speculation. Even while Microsoft-owned AI programming tool Github Copilot has $100 million average recurring revenue and produces around half of the code written in Microsoft Visual Studio, the company is still losing around $20 per month per Copilot user on compute and training data.
Generative AI is a sustaining innovation for products and a disruptive innovation for costs
Clayton Christensen classified innovations as either “sustaining,” meaning that they reinforced existing advantages, or “disruptive,” meaning that they undercut them and reset the market entirely. Apple building its own custom chips is a sustaining innovation; Apple combining a phone, a web browser, and an iPod to create a new category of smartphone is a disruptive one.
Commoditized general human intelligence services, accessible via an API, embeddable in any product—for example, ChatGPT—represent a sustaining innovation. The adoption of these services and interfaces will make software more personalized, automated, and usable. Improving these services will dramatically increase the capacity of software and make it stickier.
However, a dramatic increase in the capacity of software represents a disruptive innovation to the cost of white-collar work. Most white-collar work consists of generating text: writing code, support emails, summaries, blog posts. An army of infinite junior- to mid-level knowledge workers, accessible via an API, offers a substitute for junior- to mid-level employees, including developers, marketers, sales representatives, lawyers, customer support managers, product managers, and more.
But building a business with infinite junior employees presents infinite junior-level mistakes. There are enormous opportunities to build software businesses that delegate work to LLMs, but how will they minimize the threat of hallucinations and supervise that work at scale?
Maybe future organizational structures have significantly more senior members than they do now. The status quo of a big software engineering org chart looks like a pyramid: a few senior leaders and architects above more mid-level engineers and managers, which are themselves above more junior-level developers. What if future software organizations look like marketing orgs? Senior strategists, creatives, and leaders would manage automated systems in lieu of junior developers to deliver work at scale. Just like how Hubspot and Mailchimp automate the process of sending marketing emails, companies like Poolside could enable abundant software creation.
Technology moves operational leverage up the business chain. Before the introduction of spreadsheet software, clerks and bookkeepers outnumbered analysts, auditors, and managers by a third in the U.S. Twenty years later, there are two-and-a-half times more analysts and managers than clerks. Generative AI enables every clerk to become an analyst, every writer to become an editor, and every developer to become an architect.
SaaS is dead. Long live SaaS.
With all of these changes, nirvana is near: software as a variable cost business. No longer does a new software business need to default to developers, sales reps, or customer success managers to reliably generate cash flow at scale. Every cost is a choice, and founders can now choose to avoid the pitfalls and problems of the past.
If generative AI can reach its potential, it represents a new frontier for software, where organizational structures, cost structures, and growth strategies can and should be re-imagined. These new opportunities empower creative business development: dealmaking, marketing, elephant hunting. Imagine services businesses being able to spin up entire software lines, or the rigorous operating procedures of private equity funds being applied to building—not buying—software companies.
New ventures need new venture capital
With a new paradigm for software comes a new paradigm for financing software growth. Invested capital must be spent and underwritten entirely differently.
Venture capital as we know it today is a narrow product for a narrow set of companies in a narrow set of market conditions. Legacy venture capital was deployed into companies pursuing enormous, unsaturated markets where they could grow quickly and smother any competition. The goal was to monetize conviction in early-stage businesses and trust that someone else would write a much bigger check if your conviction paid off—distinct from the rigorous financial engineering and operational interventions of larger buyout firms like Vista or Warburg Pincus, which buy cash with precise expectations of the equity they receive for it.
The spectrum of software businesses is enormous: everything from 11-figure market cap companies fueled by machine learning engineers on drug stacks that would terrify fighter pilots to CPAs in Omaha building steady incomes from no-code tools. The financing available to those businesses is over-indexed toward the former and not the latter, but this new paradigm of software will sprout new generations of alternative software businesses that need financing options better aligned to their future than high-growth venture capital. The future median software founder will aspire to be Hank Hill, not Mark Zuckerberg. As the barrier for starting a software business lowers, founders will choose steady cash flow over frenzied hypergrowth, sleeping at home over sleeping in the office, and serving a niche audience well over conquering global markets. Venture capital rejects these founders—and these founders will reject venture capital, instead preferring non-dilutive and structured sources like credit, warrants, or loans.
Traditional “prestige” venture capital firms, by most measures, have already dug their own grave. By some crude math, most dollars invested in private software equity could not be sold for fifty cents. Most of the $1 trillion in investment tracked by Pitchbook since 2018 was in an environment with public market valuations above ten times annual recurring revenue. Currently, the median public SaaS company is valued at five times annual recurring revenue. Assuming public SaaS markets will revert to the historical mean, there is still a long way to fall. The impact on Atherton real estate remains to be seen.
A prosperous future awaits creative business developers, but on the other side of the smiling curve lies the hyperscale cloud providers building the infrastructure that will power these businesses.
One reason we’ll never make it in business journalism is because every day we’d lead our site with the headline:
AWS + AZURE + GOOGLE CLOUD AT $200 BILLION IN ANNUAL REVENUE GROWING 20 PERCENT PER YEAR
Vertical market software providers are solely focused on capturing, retaining, and upselling a specific vertical market, such as school districts, hospitals, or local retailers. An extremely generalized way to look at vertical market software is as industry-specific templates and integrations on top of commodity data warehousing and workflow offerings. Remember Robert Smith’s adage that all software companies taste like chicken—these companies are selling the same types of software, but they have to become experts in the technology stack of their vertical market: the types of data systems they have to integrate with, the standard reporting their market requires, the privacy or access concerns specific to their users.
Vertical market software works when clients have vertical-specific requirements, need vertical-specific personalization and features, and are drawn to vertical-specific brands. But as software costs become increasingly variable, cloud providers like AWS and Microsoft Azure can encroach on vertical market software providers more easily than ever before. Why pay separately for a niche supply chain software tool when Azure offers a "good enough" solution, configurable by a business manager, pre-integrated into all your other cloud services, and with vertical-specific personalization baked in, powered by generative AI?
In which markets might we see hyperscalers offer new vertical-specific applications as a loss leader for the beautifully sticky compute and storage revenue? One early example is Cybersyn, a market intelligence company offering deep economic data as a service. Its deep partnership with Snowflake allows the company to focus on building its datasets and let Snowflake deploy and monetize it. Snowflake even led Cybersyn’s $62.9 million Series A.
We wouldn’t want to compete with infrastructure companies making focused bets on vertical opportunities, but many startups are forced to. We’ll see where the hyperscalers can defeat the accumulated loyalty of a vertical client base. After all, you can’t automate taking a vice president to a steak dinner.
As hyperscalers and vertical software battle it out on the enterprise side, the lower and middle market will need services to take advantage of the opportunities to come. Enormous fortunes await those who can accelerate the deployment period of generative AI, to speed-run the Carlota Perez arc of technological revolutions. For the countless public and private sector organizations frustrated with their software, eager to adopt better practices, or just looking for a friendlier face to partner with, their business is yours for the taking.
Thanks to Kate Lee, Meghna Rao, Chris Brown, James Cham, Ben Cmejla, Hunter Hopcroft, Bill Janeway, and Anshu Mohan for editing and feedback.
John Kennedy leads education insights for Panorama Education, a data analytics company used by over a quarter of America’s public schools. Previously, he was the CEO of Mesa Cloud, a student progress platform that he founded as a high school student and led until its acquisition by Panorama.
Will Manidis is the co-founder and CEO of ScienceIO Previously he worked at Foundation Medicine, and was a Thiel fellow and the managing partner of Dorm Room Fund.
Find Out What
Comes Next in Tech.
Start your free trial.
New ideas to help you build the future—in your inbox, every day. Trusted by over 75,000 readers.
SubscribeAlready have an account? Sign in
What's included?
-
Unlimited access to our daily essays by Dan Shipper, Evan Armstrong, and a roster of the best tech writers on the internet
-
Full access to an archive of hundreds of in-depth articles
-
-
Priority access and subscriber-only discounts to courses, events, and more
-
Ad-free experience
-
Access to our Discord community
Comments
Don't have an account? Sign up!
When AI can reverse engineer the vertical specific workflows, which inform the software design itself—that is, AI as product manager—the tipping point will have arrived.