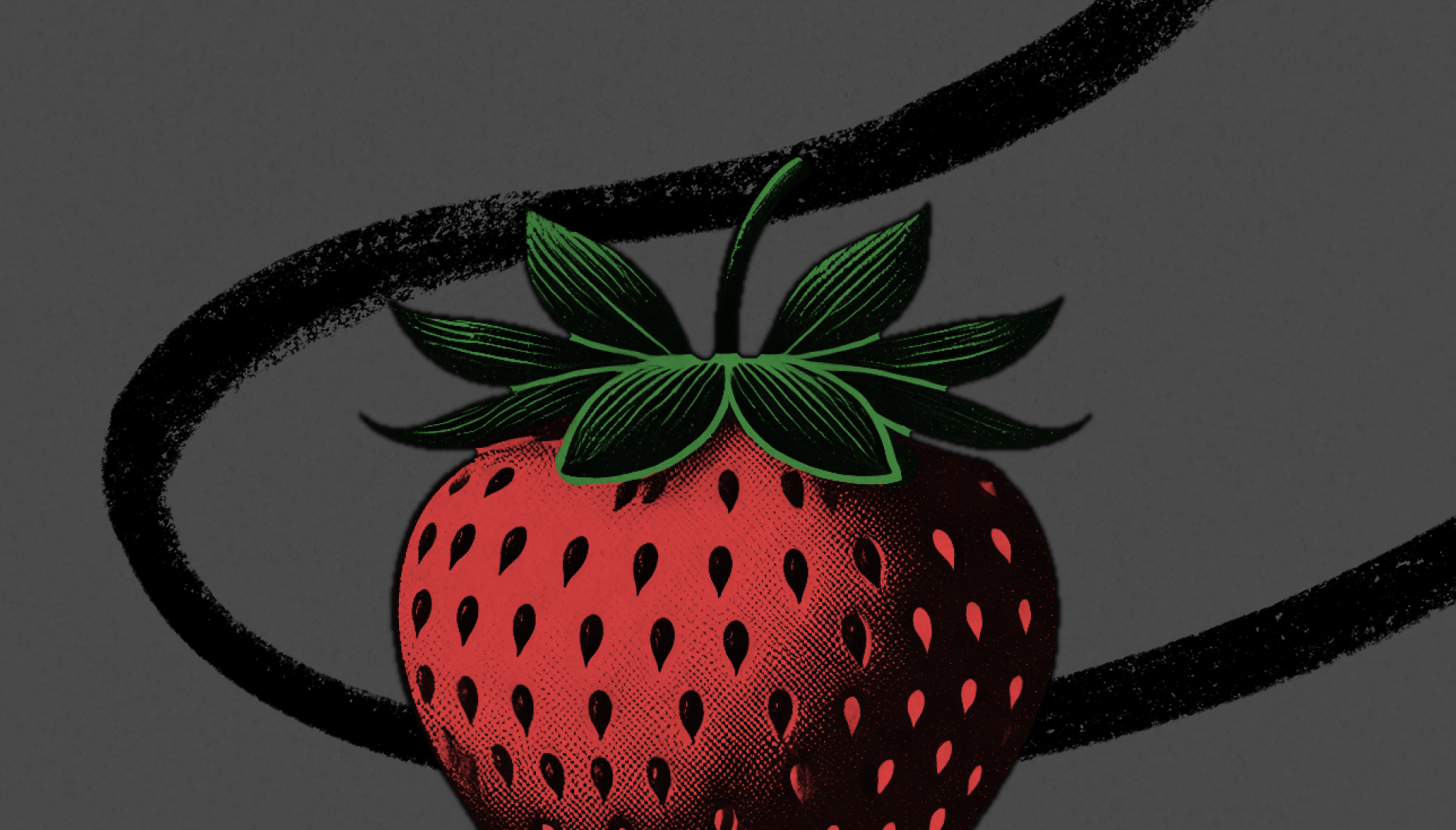
Was this newsletter forwarded to you? Sign up to get it in your inbox.
OpenAI has created a new model that could represent a major leap forward in its ability to reason. It’s called Strawberry. Here’s why:
If you ask ChatGPT how many “r”s are in the word “strawberry,” it famously fails miserably:
This occurs for two reasons. The first is that ChatGPT doesn’t actually see the word “strawberry.” Instead, it translates “strawberry” into an AI-readable language that represents words, not with letters, but with long strings of numbers. There’s no way for ChatGPT to count the number of letters in “strawberry.” It doesn’t see letters because of the way it’s built.The AI researcher Andrej Karpathy likens what ChatGPT sees to hieroglyphs, rather than letters. So asking it to count the number of “r”’s in strawberry is a little like asking it to count the number of “r”’s in 🍓. The question doesn’t really make sense, so it has to guess.
The second reason is that, even though ChatGPT can score a 700 on an SAT math test, it can’t actually count. It doesn’t reason logically. It simply makes good guesses about what comes next based on what its training data tells it is most statistically likely.
There are probably not many English sentences on the internet asking and answering how many “r”s are in the word strawberry—so its hunch is usually close, but not exactly right. This is true of all other math problems that ChatGPT is asked to solve, and it’s one reason why it often hallucinates on these types of inputs.
As initially reported by The Information, we don’t know a ton about Strawberry’s underlying architecture, but it appears to be a language model with significantly better reasoning abilities than current frontier models. This makes it better at solving problems that it hasn’t seen before, and less likely to hallucinate and make weird reasoning mistakes. Here’s what we know, and why it matters.
What Strawberry is
Strawberry is a language model trained through “process supervision.”
Process supervision means that during training, a model is rewarded for correctly moving through each reasoning step that will lead it to the answer. By comparison, most of today’s language models are trained via “outcome supervision.” They’re only rewarded if they get the answer right.
According to The Information, Strawberry “can solve math problems it hasn't seen before.” It can also “solve New York Times Connections, a complex word puzzle.” (I tried ChatGPT and Claude on Connections while writing this article. With the right prompting, I think I could get it to be a decent player, but it’s definitely not going to be expert-level.)
OpenAI originally developed Strawberry to create training data for its newest foundation model, codenamed Orion. Previous versions of its GPT models were trained by reading the entire internet. The problem was, there isn’t a lot of content on the internet where math and reasoning problems get solved step by step.
Strawberry can be prompted to generate a vast custom training set of problems with step-by-step solutions that Orion can learn to solve and, therefore, hopefully hallucinate less when it encounters similar problems in the wild.
While Strawberry was originally built to create training data, OpenAI has plans to release a smaller, faster version of it as a part of ChatGPT as soon as this fall, potentially representing a major upgrade to the LLM’s current reasoning abilities. Strawberry’s existence also implies that the next GPT model could be significantly more powerful, something that Microsoft and OpenAI have been signaling for a while.
Why Strawberry matters
Until now, the customary approach in frontier-model building has been fairly straightforward: more data and more compute.
Strawberry continues this trend, but with a particular flavor. OpenAI has chosen to use Strawberry to generate more synthetic data of a certain type: logic and reasoning problems, and their solutions.
This decision is probably due to two principles of model training and inference that we already know:
- Asking models to think step-by-step, also known as chain of thought reasoning (and the inspiration for the name of this column), improves their performance.
- Training models on programming code improves their performance even on non-coding tasks.
If both of those points are true, then a frontier model trained on lots of math and reasoning problems, with their solutions spelled out step-by-step, will produce a much smarter model. So OpenAI set out to build Strawberry, which could help it produce the examples of good reasoning it needs to be able to follow the trails of logical thought.
On that note, one interesting detail The Information mentioned about Strawberry is that it “can solve math problems it hasn't seen before—something today's chatbots cannot reliably do.”
This runs counter to my point last week about a language model being “like having 10,000 Ph.D.’s available at your fingertips.” I argued that LLMs are very good at transmitting the sum total of knowledge they’ve encountered during training, but less good at solving problems or answering questions they haven’t seen before.
I’m curious to get my hands on Strawberry. Based on what I’m seeing, I’m quite sure it’s more powerful and less likely to hallucinate. But novel problem solving is a big deal. It would upend everything we know about the promise and capabilities of language models.
Dan Shipper is the cofounder and CEO of Every, where he writes the Chain of Thought column and hosts the podcast AI & I. You can follow him on X at @danshipper and on LinkedIn, and Every on X at @every and on LinkedIn.
Comments
Don't have an account? Sign up!
Dan.... you are our canary in the mine!!!! Your extreme curiosity, extraordinary talent, and high sensitivity with all 5 senses makes you the best person to poke the Strawberry around your work table when you get it. The best part, Dan? The best is your strength in writing clearly about the uniqueness; specifically, "Here's what's so great about this AI thingie" and "Here's where humanity still rules and is absolutely necessary for technology businesses to even exist."